Introduction
Various biotic and abiotic factors are involved in the degradation and destruction of the forest-one of the world’s most important natural resources. This situation is the result of global warming, which is the main environmental problem for Earth’s current population. Incidence of drought and desertification are the strongest indicators of this situation. As a result of the destruction of the forest ecosystem, semi-arid areas are formed, and drought and desertification arise due to the increasing severity of the consequences of human-induced climate change (Türkeş, 2012). From a macroperspective, forest assets and diversity of species are also affected due to a decrease in the amount of precipitation (Fang et al., 2005; Food and Agriculture Organization of the United Nations [FAO], 2018; Park & Sohn, 2010; Yang, Fang, Ma, & Wang, 2008).
It is very difficult to maintain the continuity of forest assets in semi-arid regions, thus, resulting in the decrease of forest assets in semi-arid areas over the last 40 years (Song et al., 2018). Factors increasing deforestation also trigger landslides and erosion, which reduce the amount of living cover protecting the upper layer of the soil. Increasing the vegetation cover as much as possible is crucial for both water retention and reducing the extreme points in the water balance sheet (Tang et al., 2018).
Turkey is located in the temperate zone and considered a macroclimate (Yilmaz & Cicek, 2018). It borders the sea on three sides, and there are more forest assets in regions along the coast than further inland, which are considered semi-arid areas with little rainfall, due to the effects of climate change (General Directorate of Forestry [GDF], 2015). Afforestation and rehabilitation activities have been carried out for many years in order to increase the quantity and productivity of forests, which is especially scarce in the inner parts of Turkey (GDF, 2017). Considering that approximately 43 % of Turkey's forests are degraded (GDF, 2015), the severity of the situation is increasing.
In areas where degraded forests are widespread, it is necessary to increase the success of forest maintenance, regeneration, and rehabilitation works, and to ensure benefit from forests with minimum damage (Keller, Asner, Silva, & Palace, 2004; Soriano, Kainer, Staudhammer, & Soriano, 2012; Sirén, Ala-Ilomäki, Mäkinen, Lamminen, & Mikkola, 2013; Wilson & Oliver, 2000). The importance of precision forestry applications, especially in semi-arid regions, is increasing. The forests can be stabilized and then rehabilitated, even after many years, through technical and ecological measures, such as ecological restoration, rehabilitation, and afforestation, especially in areas with extreme climate (semi-arid, arid, dune, and erosion areas). However, in order to reestablish productive forests in semi-arid and arid areas, it is necessary to first obtain detailed, sensitive, and high-quality data during the implementation of technical and ecological measures, and to then carefully plan the forest establishment activities based on these data (Raum, 2017). These forest planning activities support the main concerns of the management, and objective assessment of forest projects, from a scientific and economic point of view. For this reason, well-designed planning procedures can be implemented to allow the monitoring of everything from simple anthropogenic disturbances to more complex climate, geomorphological, and physical disturbances (Vallauri, Aronson, Dudley, & Vallejo, 2005).
Considering the sensitive ecological conditions of both semi-arid and arid climates, the success of the aforementioned applications in these areas can be increased by (a) rehabilitation of degraded forests, reforestation, and harvesting activities carried out in existing forest assets (Daoyin & Yaoxiang, 2007), (b) planning them with a multidirectional approach by taking the factors affecting these activities into account (Bizikova & Krcmar, 2015).
Many studies in the current literature that were conducted in landslide areas used different modeling approaches for multidirectional evaluations. The basis of these approaches is the assessment of multiple factors. Some of these approaches are as follows: Machine Learning (Sahin, Colkesen, & Kavzoglu, 2018), Adaptive Neuro Fuzzy Inference System (Sezer, Pradhan, & Gokceoglu, 2011), Artificial Neural Networks (Chen, Pourghasemi, & Zhao, 2017), Support Vector Machine (Chen, Pourghasemi, & Naghibi, 2018), Logistic Regression (Ayalew & Yamagishi, 2005), Fuzzy Inference System (Pourghasemi, Pradhan, & Gokceoglu, 2012), Modified-Analytic Hierarchy Process (Kornejady, Pourghasemi, & Afzali, 2019), Bivariate Statistical Analysis (Jiménez-Perálvarez, Irigaray, El Hamdouni, & Chacón, 2011), Statistical Index (Pourghasemi, Moradi, & Aghda, 2013), and Frequency Ratio (Akgun, Dag, & Bulut, 2008).
Various factors are used in order to present landslide susceptibility map (LSM), including elevation (Ayalew & Yamagishi, 2005; Lee & Sambath, 2006), distance to roads (Dahal et al., 2008), land use (Bai et al., 2010), slope (Gokceoglu & Aksoy, 1996), distance to faults (Pourghasemi et al., 2012), lithology (Yalcin, 2008), distance to streams (Pourghasemi et al., 2013), aspect (Yesilnacar & Topal, 2005), plan curvature (Nefeslioglu, Duman, & Durmaz, 2008), and the topographic wetness index (Devkota et al., 2013).
This study was carried out in a semi-arid region susceptible to landslides, which is one of the factors negatively affecting forest assets. The objective of the research was to model the landslide susceptibility in terms of topographic factors (elevation, slope, faults, lithology, aspect, and plan curvature) and to support decision-making in activities to be conducted in such areas. It will then be possible to carry out more successful forestry activities (construction of forest infrastructure facilities, harvesting, afforestation and rehabilitation) in forests susceptible to landslide during planning and application in the context of precision forestry. Also, with the addition of landslide susceptibility to decision-making processes as a factor, the success of forestry activities, which are long-term investments, could be increased.
Materials and Methods
Study Area
The study area is located within the area of responsibility of Sarikaya Forest Sub-District Directorate in Cankiri Forestry Management Directorate affiliated with Ankara Forestry Regional Directorate. This area is located between 40° 47' 56" - 40° 46' 51" northern latitude and 33° 58' 08" - 34° 01' 06" eastern longitude. It has a size of 171.37 km2 and landslides of various numbers and sizes have occurred in the past years (Figure 1). The study area is located in a high mountainous area. The average slope of this area is 15°, the dominant aspect is the south facing direction and the average height is 1 410 m, ranging from 1 083 m to 2 061 m.
There are not any common procedures to establish the most influential factors in LSM studies (Sahin et al., 2018). Combinations of different factors are used in each LSM study. The most important feature affecting these combinations comes from whether the data are available or not. This situation, which is to affect the success of the direct models, leads researchers to carry out modeling using different factors and their combinations in different landslide areas. Pourghasemi et al. (2012) stated that the factors of height, slope (degree), aspect, and lithology were most used in LSM studies. In addition to this, data quality affects the success of the model significantly (Jacobs et al., 2018). In this study, 12.5 m × 12.5 m high-resolution digital elevation model (DEM) data were used. All the factors used in this study were obtained from this DEM. Six factors were evaluated for the modeling in the study: elevation, slope (degree), distance to faults, lithology, aspect, and plan curvature.
Factors
Elevation is one of the most commonly used factors in landslide susceptibility studies (Buğday, 2018; Meng et al., 2016). The average height in the study area is 1 410 m, minimum height is 1 100 m, and maximum height is 2 050 m. In this study, the elevation factor has been classified in five classes (1 000 m to 2 250 m; Figure 2a). Slope (degree) is one of the most frequently used factors in landslide susceptibility studies (Behling, Roessner, Kaufmann, & Kleinschmit, 2014; Eskandari, Homaee, & Falamaki, 2016; Niu et al., 2018). The average slope in the study area is 14.33°, whereas the minimum is 3.25°, and maximum is 54.83°. In this study, the slope was classified into five different groups: 0-4.99°, 5-11.99°, 12-17.99°, 18-21.99° and 22-65.8° (Figure 2b). Distance to faults is an important factor increasing the landslide formation and the size of landslides considerably (Feizizadeh, Blaschke, & Nazmfar, 2014; Meng et al., 2016). Distance to faults was classified into six different groups: 0-0.99 km, 1-1.99 km, 2-2.99 km, 3-3.99 km, 4-4.99 km, and 5-10 km (Figure 2c). Lithology is a factor commonly included in landslide susceptibility studies (Pourghasemi & Kerle, 2016). Lithology map was derived from the 1:100 000 scale geological map (Duman, Çan, & Emre, 2011) obtained from the General Directorate of Mineral Research and Exploration (MTA). There are six different lithological groups in the study area: clastic and carbonate rocks (k2: Cretaceous), terrestrial clastic rocks (pl: Pliocene), evaporative sedimentary rocks (olm1: Oligocene-Miocene), clastic rocks (e1-2: Eocene), basic and ultrabasic rocks (of: Mesozoic) and Quaternary (Q) formations (Figure 2d). The study area is one of the areas where landslides occur frequently within the provincial boundaries. It is highly prone to landslides due to the Cretaceous age and sandstone content (Sahin et al., 2018). The aspect factor is one of the factors included in this type of study (Niu et al., 2018; Yalcin, Reis, Aydinoglu, & Yomralioglu, 2011), and it was classified into five different groups (plain, north, east, south and west) in this study (Figure 2e). The dominant aspect of the study area is the south. Plan curvature factor is one of the factors frequently referred to in order to establish the direction of slope in landslide studies (Oliveira, Zêzere, Guillard-Gonçalves, Garcia, & Pereira, 2017; Pourghasemi & Kerle, 2016). Plan curvature was divided into five groups for this study: -0.098, -0.023, 0.013, 0.093, and 0.639 (Figure 2f).
Landslide Susceptibility Mapping Process
The process and procedures carried out to establish LSM in this study are provided in Figure 3’s flowchart.
In this study, the locations and sizes of landslides (Duman et al., 2011) were obtained from the MTA. A total of three different models were formed by using six factors in the study. The models were generated according to fuzzy inference system (FIS) and modified analytical hierarchy process (M-AHP) approaches. FIS is an approach that is widely used in simulation processes (Bellman & Zadeh, 1970; Chang & Zadeh, 1996). The values in the FIS approach are not limited to 0 to 1, but the studies with intermediate values are reflected in the database. In the models generated, according to the FIS approach, membership functions are assigned first. At this stage, depending on which factor is low or high, the degree to which landslide susceptibility will be impacted (very low-very high) is entered and learning rules are determined. In the following process, the model is generated as a result of the combination of factors on the scale of these rules. The M-AHP approach has various advantages in the modeling process in terms of eliminating the subjectivity of the classical AHP approach and not requiring user experience (Banuelas & Antony, 2004; Pourghasemi & Rossi, 2017). Here, after the values included within the factors are normalized, they are scored according to the rate of affecting the landslide susceptibility (1, 3, 5, 7, 9, etc.). Afterwards, these factors are combined and the models are obtained for LSM. The scores used for M-AHP in this study are displayed in Table 1. The combinations of the factors used in the models formed according to FIS and M-AHP approaches in this study are shown in Table 2.
Table 1 Factors’ Scores for modified analytical hierarchy process (M-AHP) in this study.
Factors | Classes | Score | Factors | Classes | Score |
---|---|---|---|---|---|
Elevation (m) | 1 000 - 1 250 | 1 | Slope (degree) | 0 - 5 | 1 |
1 250 - 1 500 | 3 | 5 - 12 | 3 | ||
1 500 - 1 750 | 5 | 12 - 18 | 5 | ||
1 750 - 2 000 | 7 | 18 - 22 | 7 | ||
2 000 - 2 250 | 9 | 22 - 66 | 9 | ||
Aspect | Flat | 1 | Plan Curvature | -0.098 | 9 |
North | 3 | -0.023 | 7 | ||
East | 3 | 0.013 | 5 | ||
South | 7 | 0.093 | 3 | ||
West | 5 | 0.639 | 1 | ||
Distance to Faults (km) | 0-1 | 9 | Lithology | Alluvium | 9 |
1-2 | 9 | Sandstone | 7 | ||
2-3 | 7 | Sandstone - Mudstone | 5 | ||
3-4 | 5 | Melange | 3 | ||
4-5 | 3 | Shale | 1 | ||
5-10 | 1 | Volcanite - Sedimentary rock | 5 | ||
Conglomerate - Sandstone - Mudstone | 5 |
Table 2 Combinations of factors used in the models formed according to fuzzy inference system (FIS) and modified analytical hierarchy process (M-AHP) approaches.
Factors | Model 1 | Model 2 | Model 3 |
---|---|---|---|
Elevation | ( | ( | ( |
Slope (degree) | ( | ( | ( |
Distance to faults | ( | ( | ( |
Lithology | ( | ( | ( |
Aspect | ( | ( | - |
Plan curvature | - | ( | - |
Model Validation
In this study, the widely used and reliable receiver operating characteristic (ROC) curve (Buğday, 2018; DeLong, DeLong, & Clarke-Pearson, 1988; Oh & Pradhan, 2011) was preferred for the validation of the models generated. The ROC curve is calculated by the ratio of sensitivity to accuracy, where the threshold value for binary classification systems differs (the threshold value was determined as 0.50). The success rate of the models was determined by the area under curve (AUC).
Results and Discussion
It was determined that, in this study area, a total of 107 landslides occurred (1965-2017), with an average size of 12.9 ha, a minimum of 1.11 ha, and a maximum of 144.76 ha, according to the landslide inventory obtained from the MTA. The total area of landslides within this area is 1 357.68 ha. The landslides that occurred in the area, according to the MTA classification, consist of 430.75 ha of former landslide areas, and 926.93 ha of active landslide areas. It is reasonable to assume that the study area is under constant landslide pressure.
A total of six models were generated in this study; three of them according to the FIS approach, and three according to the M-AHP approach (Figure 4). The values obtained after the validation of these models were as follows: Model 1 AUC = 79.9 %, Model 2 AUC = 78.3 %, and Model 3 AUC = 80.2 %, according to the FIS approach. According to M-AHP approach, the following values were determined: Model 1 AUC = 78.1 %, Model 2 AUC = 75.9 %, and Model 3 AUC = 72.9 % (Figure 5).
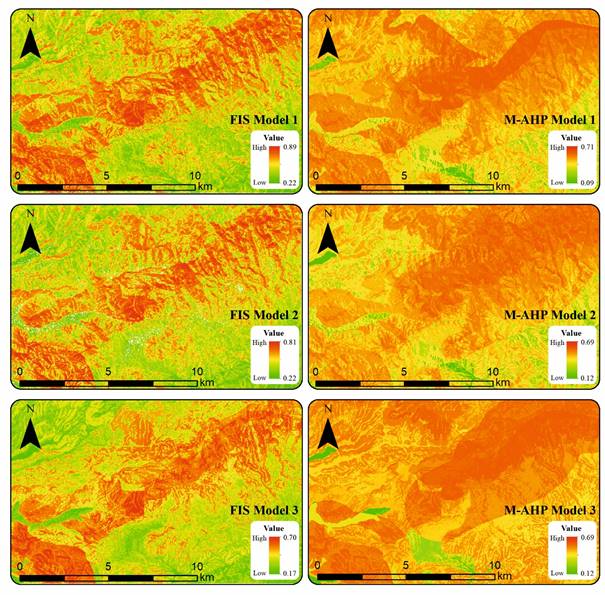
Figure 4 Models layouts according to fuzzy inference system (FIS) and modified analytical hierarchy process (M-AHP) approaches.
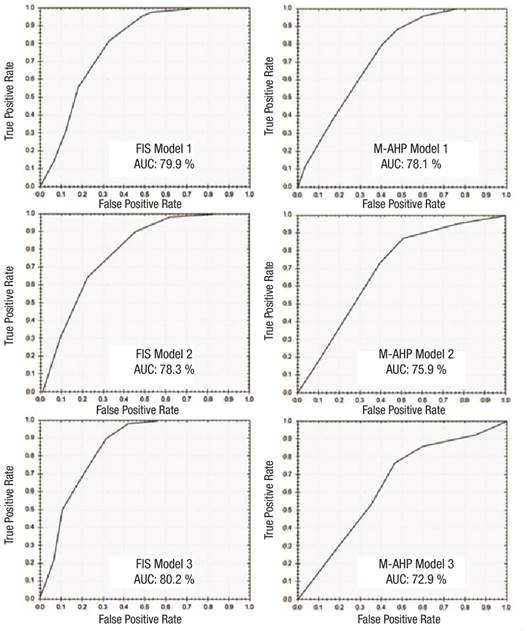
Figure 5 Models’ validations (receiver operating characteristic curve and area under curve [AUC] values) using fuzzy inference system (FIS) and modified analytical hierarchy process (M-AHP) approaches.
Landslide susceptibility should be considered as a factor in all forestry activities carried out in Turkey in order to increase the forest assets in the semi-arid areas and ensure their sustainability. Within this context, this study was carried out in order to support planning in landslide sensitive areas and their surrounding forest areas using advanced GIS methods. The model that best conveys the LSM among the six models obtained from the study is FIS Model 3 (AUC = 80.2 %). Factors composing FIS Model 3 are elevation, distance to faults, and lithology. By using the FIS method, Vahidnia et al. (2010), Akgun et al. (2012), and Pourghasemi et al. (2012) generated LSM model with 90.5 %, 85.5 %, and 89.7 % success rates, respectively. Jiménez-Perálvarez (2011) and Buğday (2019) obtained results similar to those of this study, generating a model with an 83.2 % and 82.1 % success rate, respectively, using the FIS approach in the mountainous semi-arid area. In this study, more successful results were obtained with FIS models than M-AHP models. Similar to this study, Pourghasemi et al. (2012) compared the FIS approach and AHP approach and found that FIS generated more successful models. As with all modeling studies, success in conducting landslide susceptibility modeling depends on quality data (Buğday, 2018; Yalcin, 2008). Precision forestry principles can be used to access quality information. In such studies, obtaining sensitive data and the success of models generated from this data were considered to be higher.
Conclusions
The main purpose of modeling is to perform analyses with multiple parameters and data to reveal the most successful models with the least number of factors. In framework of sustainable forest management, and precision forestry principles, evaluating unpredictable factors using models increases the reliability of conducting forestry applications (road construction, harvesting, reforestation, afforestation, protection, etc.), in landslide-susceptible forestlands. Therefore, it is important to model the negative effects such as climatic, topographical, environmental, and anthropogenic, that may affect the success of forestry activities. In further studies, the success of planning can be increased by using other parameters in the modeling of landslide sensitive areas in forestlands. In addition, these parameters can be obtained more precisely by using new technologies (unmanned aerial vehicle, light detection and ranging, etc.) to produce more successful models. This will be a great advantage for both decision makers and planners.