INTRODUCTION
Severe acute respiratory syndrome coronavirus 2 (SARS-CoV-2), the etiologic agent of the pandemic disease coronavirus disease 2019 (COVID-19), is a positive-sense single-stranded RNA novel betacoronavirus that is highly transmissible and whose infection in humans manifests itself as mild symptoms to severe respiratory failure or even multiple organ failure1,2. According to genomic analyses results, SARS-CoV-2 shares 79.5% sequence identity with SARS-CoV and 50% with middle east respiratory syndrome coronavirus (MERS-CoV)3. Moreover, its genome consists of six major open-reading frames that are common to coronaviruses and a number of other accessory genes4.
With transmission predominantly by aerosols and respiratory droplets and a mean incubation period of 3-4 days, SARS-CoV-2 invades host cells via binding to the angiotensin-converting-enzyme-2 (ACE 2) receptor, which is found mainly in the epithelium of the respiratory tract, but also the epithelium of other organs such as the intestine and endothelial cells in the kidney and blood vessels5. The pathophysiological process triggered from this infection is linked not only to the viral mechanisms of infectivity but also to the pattern of the elicited host response. According to the three-step COVID-19 pathogenesis model, the complex interaction between host and viral responses results in a dynamic spectrum of clinical manifestations that can be schematically grouped into three phases: pulmonary phase, marked by ACE deficiency, pneumonia, and severe acute respiratory syndrome; pro-inflammatory phase, with hyperproduction of cytokines (resulting in a so-called “cytokine storm”), systemic inflammation, and acute lung injury; and pro-thrombotic phase, in which widespread platelet aggregation and thrombosis give rise to coagulopathy and multi-organ failure6.
In light of the high global morbidity and mortality of COVID-19 and the elevated socioeconomic costs imposed by this disease, the scientific community has engaged in efforts to develop and repurpose drugs for its treatment. In this scenario, considering the need for the swift development of therapeutic measures, the repurposing of clinically evaluated drugs represents a promising strategy for rapid identification and deployment of treatments for SARS-CoV-2 infection7. Several attractive molecular targets for viral inhibition that can be exploited by repurposed drugs are provided by the structure, life cycle, and pathogenic mechanisms of this virus8. Among those, chymotrypsin-like protease (3CLpro), also called main protease (Mpro), is a potential target of great interest.
3CLpro, which controls the activities of the coronavirus replication complex, is the main protease of this viral group, being observed at high degree of structural similarity and conservation of the active site among the main proteases of SARS-CoV-2, SARS-CoV, and MERS-CoV9,10. Since this protease cleaves the virus-encoded polyproteins, it is necessary for viral maturation and thus indispensable to the infectious process11,12.
Drug screens and structure-based designs targeting 3CLpro have identified a variety of compounds from different therapeutic classes that inhibit its activity. These compounds can be classified into two categories according to the integration of their effects in the infectious and pathophysiological process triggered by SARS-CoV-2: (i) drugs whose effect on the disease is restricted to antiviral action resulting from protein inhibition (and, possibly, by other virus-related mechanisms); and (ii) drugs that, in addition to antiviral action, exert a beneficial effect on the control of the host immune and respiratory function (through, for example, anti-inflammatory activity with cytokine suppression or anticoagulant activity or enhancing respiratory function property)13.
To contribute to the recognition of pre-existing drugs with this dual action on SARS-CoV-2 infection, and considering the pathophysiological progression of COVID-19, this work proposed the virtual screening of potential 3CLpro inhibitors based on chemical fingerprints among anti-inflammatory, anticoagulant, and respiratory system agents using a deep learning approach.
METHODS
This work was developed based on a drug property prediction framework, in which the evaluated property was the ability to inhibit the activity of the 3CLpro protein, treated as a binary variable (0 for inactive and 1 for active). The goal was to virtually screen this property in a set of drugs classified as “immunosuppressive agents”, “anticoagulants”, and “respiratory system agents”, to suggest potential candidates for repurposing for the treatment of SARS-CoV-2 infection, especially in patients manifesting respiratory conditions. Such prediction was performed using a dense neural network (DNN) trained and validated on bioassay data.
The training data came from the screening bioassay record AID170614, which belongs to the assay project “Summary of probe development efforts to identify inhibitors of the SARS coronavirus 3C-like Protease”15, whose purpose was to identify compounds that inhibit SARS-3CLpro-mediated peptide cleavage using fluorescence measurements to estimate the average percentage inhibition for the compounds tested at a concentration of six micromolar. In this assay, compounds with an activity score of 0 to 15 were classified as inactive compounds, and compounds with an activity score of 15 to 100 were classified as active compounds. A total of 290,726 compounds were evaluated in the aforementioned assay, among which 405 were labeled as active. In order to create a less unbalanced training set, 270,321 negative and all 405 positive samples were selected to compose it, and the positive ones were oversampled by 200. Thus, the training set comprised 270,321 instances labeled as 0 and 81,000 instances labeled as 1, encompassing a total of 270,726 unique compounds.
The validation set consisted of 20,176 negative (inactive) and 69 positive (active) compounds. The negative samples were from: the AID1706 record14 (n = 20,000), which were randomly selected and not included in the training set); and the bioassay record AID 124035816 (n = 176), in which compounds were evaluated for inhibition of the 3C-like protease from bat coronavirus HKU4, the likely reservoir host to the human coronavirus that causes MERS17. The positive samples were from: the bioassay records AID 488958, AID 488999, AID 493245, AID 588771, AID 588772, and AID 58878618-23, confirmatory assays linked to the same assay Project15 (n = 41); and the bioassay record AID 124035816 (n = 28). There was, therefore, no overlap between the training and validation data, and in the validation set, there were active and inactive compounds evaluated in different projects/publications.
In addition, to add robustness to the predictive generability evaluation, an external test set was adopted, consisting of 71 positive and 1,253 negative molecule fragments for covalent or non-covalent binding to the active site of 3CLpro. This data were obtained through combined mass spectrometry and X-ray screen24. It is important to highlight that, while in the mentioned assays, the inhibition capacity of the protease activity demonstrated by the compounds was evaluated; this screening evaluated binding properties related to the active site of the protease. Thus, the difference in the nature of the data, despite the correlation between them, has to be considered.
The predictive variables – that is, the compounds – were represented in the form of PubChem Substructure Fingerprints25, which encodes molecular fragment's information with 881 binary bits. Each bit represents the presence of a certain feature (e.g., an element count, a type of ring system or an atom pairing) in a chemical structure. A DNN – whose structure is summarized in table 1 – was then trained on training set (receiving 881 binary features as input for each instance) and validated on validation set. As for the model's performance on this second set, sensitivity, specificity, and accuracy values were calculated. The receiver operating characteristic (ROC) curve was also plotted, along with the corresponding area under the curve (AUC) value.
Table 1 Drug-property prediction DNN summarized structure
Type of layer | Activation function | Number of units | Number of parameters |
---|---|---|---|
Dense | Relu | 256 | 225,792 |
Dense | Relu | 8 | 2,056 |
Dense | Sigmoid | 1 | 9 |
DNN: dense neural network; Relu: rectified linear unit.
The model was then used to screen (binary predicting 3CLpro inhibition activity or inactivity, based on prior training) 1,278 compounds with anti-inflammatory (n = 733), anticoagulant (n = 163) or respiratory (n = 382) action, and thus identify potential repurposing candidate drugs for the management of SARS-CoV-2 infection and disease. These compounds were collected in the PubChem Classification Browser repository, corresponding to records annotated with the medical subject headings descriptors “Anti-inflammatory Agents,” “Anticoagulants,” and “Respiratory System Agents” (excluding nasal decongestants and central respiratory stimulants).
The predictive significance of each of the features (PubChem Substructure Fingerprints bits) used in the representation of the molecular structures was also estimated. This was done using the Deep SHAP approach, an additive feature attribution method used for deep-neural networks that recursively passes the compositional approximation of Shapley values (representations of feature weights) backward through the network and, therefore, satisfies local precision, missingness, and consistency26,27.
All steps of data processing, model development and virtual screening were implemented in Python. To obtain the PubChem fingerprints, the PaDEL-Descriptor software28 was used, and the Keras library was used to develop the deep neural network.
RESULTS
The proposed DNN binary classifier was trained for 150 epochs using the Adam optimizer (with a learning rate of 0.001) and binary cross entropy as loss function. On the validation set, the model was able to correctly label compounds when it comes to their 3CLpro inhibition activity 52 out of 69 active compounds and 19,871 out of 20,176 inactive compounds, thus obtaining sensitivity and specificity rates of 75.4% and 98.5%, respectively. On the test set, the model predicted 3CLpro inhibition activity for 33 out of 71 active site binders and 1,186 out of 1,253 active site non-binders, thus obtaining “sensitivity” and “specificity” rates (if interchangeability between active site binding and protease inhibition is assumed) of 46.8% and 94.7%, respectively. The corresponding ROC curves for validation and test sets are depicted in figures 1 and 2, respectively.
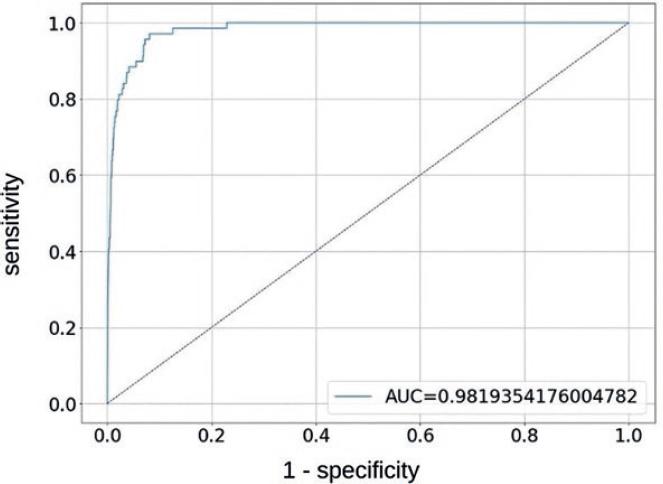
Figure 1 Receiver operating characteristic curve for models performance on validation set. AUC: area under the curve.
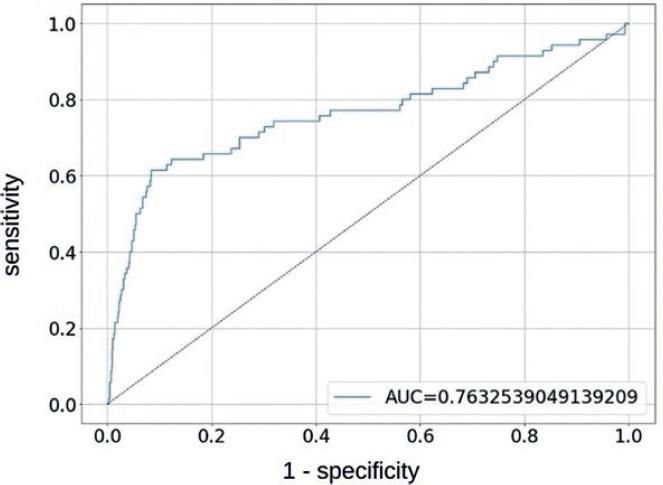
Figure 2 Receiver operating characteristic curve for models performance on test set. AUC: area under the curve.
Regarding the 1,278 compounds screened, the model indicated as potential 3CLpro inhibitors (predicted label = 1): the anti-inflammatory agents celecoxib (compound CID = 2662), gadolinium chloride (compound CID = 61486), fenoprofen calcium (compound CID = 64746, 64747, 14010989, and 67668959) and SC-236 (compound CID = 9865808); the anticoagulants DX-9065a (compound CID = 122128) and dpc-602 (compound CID = 9915041); and the respiratory agent zafirlukast (compound CID = 5717). The remaining compounds were classified as inactive (predicted label = 0). Among those, celecoxib, fenoprofen calcium, and zafirlukast are FDA-approved drugs, while the remaining are experimental drugs.
An adequate distribution of predictive weights was observed among the molecular features used in the representation of the compounds (according to the fingerprints approach adopted). The 20 bits of the molecular representation of the compounds with the highest predictive importance in model's outputs according to the Deep SHAP analysis are shown in table 2.
Table 2 Feature importance of the top-20 influential PubChem Fingerprint bits used for molecular representation in model's predictions on test set
Bit number | Bit description | Bit section | Average impact (×10²) |
---|---|---|---|
576 | N=C–C:C-[#1] | Simple SMARTS patterns | 1.05 |
539 | N=C–C–[#1] | Simple SMARTS patterns | 0.90 |
523 | N:C:C–C | Simple SMARTS patterns | 0.88 |
672 | O=C–C=C–[#1] | Simple SMARTS patterns | 0.87 |
531 | S–C:C–C | Simple SMARTS patterns | 0.83 |
259 | ≥ 3 aromatic rings | Rings in a canonic ESSR ring set | 0.81 |
528 | [#1]–N–C–[#1] | Simple SMARTS patterns | 0.78 |
602 | O=C–C–N–C | Simple SMARTS patterns | 0.74 |
180 | ≥ 1 saturated or aromatic nitrogen–containing ring size 6 | Rings in a canonic ESSR ring set | 0.72 |
659 | C–C–S–C–C | Simple SMARTS patterns | 0.71 |
691 | O–C–C–C–C–C–N | Simple SMARTS patterns | 0.69 |
357 | C(~C)(:C)(:N) | Simple atom nearest neighbors | 0.68 |
712 | C–C(C)–C(C)–C | Simple SMARTS patterns | 0.65 |
699 | O–C–C–C–C–C(C)–C | Simple SMARTS patterns | 0.64 |
698 | O–C–C–C–C–C–C–C | Simple SMARTS patterns | 0.64 |
372 | C(~H)(:C)(:N) | Simple atom nearest neighbors | 0.62 |
185 | ≥ 2 any ring size 6 | Rings in a canonic ESSR ring set | 0.60 |
412 | S(~C)(~C) | Simple atom nearest neighbors | 0.57 |
418 | C=N | Detailed atom neighborhoods | 0.56 |
405 | O(~C)(~C) | Simple atom nearest neighbors | 0.55 |
ESSR: extended set of smallest rings; SMARTS: SMILES arbitrary target specification.
DISCUSSION
Deep learning has shown high performance in virtual screening – among others, against chemical libraries to identify candidate compounds for drug repurposing –, contributing significantly to research in biological sciences and drug discovery29. Repurposing drugs available for other diseases would be beneficial for COVID-19 management, as these can be directly tested as anti-SARS-CoV-2 drugs30.
In clinical situations where a dual effect of the drug is desired, the strategy of repurposing drugs that are already known to act on one of the intended pathophysiological aspects, investigating potential action on another aspect, becomes even more beneficial. In the case of COVID-19, a multisystemic disease with potentially significant respiratory involvement and with major immune dysregulation involved, it is interesting to screen drugs that act in these systems (with potential symptomatic relief and prevention of complications) for combative properties to SARS-CoV-2 infection.
A deep learning-based virtual screening strategy was adopted in the present work, which evaluated the potential of 733 anti-inflammatory drugs, 163 anticoagulants, and 382 respiratory drugs for repurposing to treat COVID-19 based on the prediction of the inhibition property of 3CLpro, using as an input the complex molecular representation of the compounds based on 881 binary chemical features. The compounds celecoxib, gadolinium chloride, fenoprofen calcium, SC-236, DX-9065, dpc-602, and zafirlukast were predicted to be active, being celecoxib, fenoprofen calcium, and zafirlukast FDA-approved drugs.
Celecoxib, a pyrazole nonsteroidal anti-inflammatory drug (NSAID), selectively inhibits cyclo-oxygenase-2 (COX-2), which is expressed heavily in inflamed tissues where it is induced by inflammatory mediators31,32. It was pointed out as a possible SARS-CoV-2 Mpro inhibitor in a molecular-docking virtual screening33 and as an adjuvant treatment promotes the recovery of all types of COVID-19 and further reduces the mortality rate of elderly and those with comorbidities in a clinical study34. The NSAID fenoprofen calcium inhibits both isozymes of COX and activates both peroxisome proliferator activated receptors35; therefore, it may downregulate leukotriene B4 production and thereby interfere with the leukotriene pathway of inflammatory exacerbation, which has been demonstrated to mediate lung injury in several diseases35-37.
The experimental anti-inflammatory agent's gadolinium chloride and SC-236 are, respectively, a TRP channel blocker38 and a potent and selective COX-2 inhibitor39. Gadolinium chloride, which acts as a macrophage inhibitor, has been shown to attenuate acute lung injury and pulmonary apoptosis in septic patients40, as well as effectively attenuate lung ischemia-reperfusion injury by the reduction of macrophage-dependent damage41. SC-236 suppresses the nuclear translocation of RelA/p65 subunit of NF-κB, whose signaling cascade is abnormally activated in SARS-CoV-2 infection and whose inhibition has been touted as promising in the management of COVID-1942,43.
DX-9065 and dpc-602 are experimental selective inhibitors of coagulation factor Xa (FXa), a serine protease, and are part of the group of novel anticoagulants with improved pharmacologic and clinical profiles, offering benefits over traditional therapies, that are in development44. It has been shown that elevated levels of FXa are related not only to hypercoagulability in patients with severe COVID-19, but also to inflammatory exacerbation and viral infection mechanisms, what positions FXa inhibitors as a potential prophylactic and therapeutic treatment for high-risk patients with COVID-1945. Furthermore, considerable active site similarity based on 3D fingerprints and the positioning of catalytic residues was observed between the FXa protease and the 3CL protease46, and three FXa inhibitors were screened as potential inhibitors of 3CLpro in an in silico molecular docking of ligand selection47.
Zafirlukast is a cysteinyl leukotriene type 1 receptor competitive and selective antagonist that has anti-inflammatory properties and leads to bronchodilation48. In a molecular docking study, zafirlukast was identified to interact significantly with 3CLpro49. According to other virtual screenings conducted from homology models of receptor binding domain, zafirlukast may have the potential to inhibit the binding of another SARS-CoV-2 protein, the spike glycoprotein, to the ACE-2 receptor, adding another potential mechanism of action of the drug against viral infection50,51. Another deep learning study using MACCS fingerprints as molecular representations predicted this drug to inhibit 3CLpro52. Furthermore, by virtue of its anti-inflammatory activity, zafirlukast could interfere with the hyperinflammatory cytokine profile of COVID-19.
The physiological effects played by the aforementioned compounds and the potential concurrent inhibition of 3CLpro point to a possible desirable synergistic effect in the management of patients with COVID-19, a multisystemic disease with an intricate pathophysiology. Importantly, several preclinical experiments (and possibly further clinical trials) are required to characterize their virus interaction profiles as well as to evaluate the clinical benefits and safety profile of these compounds in the context of SARS-CoV-2 infection. Furthermore, by adopting a drug property prediction framework, this study did not focus on other aspects (e.g., adverse effects) that are essential to choosing candidates for repurposing. This should be taken into consideration in further studies.
Considering structure-activity relationship (SAR), a concept in which molecules with similar structures are destined to have similar biological activities, as a central concept in deep learning models for drug property prediction, it is important to identify the most influential features on the predictions, to confer explainability to the model. Thus, visualization of the decision distribution and recognition of the bits of the compounds' fingerprints with the highest weights in the analysis performed by the model to predict 3CLpro inhibition activity or inactivity, helps to remove biases related to over-attribution of weight to point features observed in instances of the training set, to inform the predictive decision, and to provide insights into molecular structural aspects related to such activity. In this sense, it is worth noting that among the 20 bits of greatest predictive importance, 12 tested for the presence of simple SMILES arbitrary target specification patterns, including the first 4.
Regarding the performance of the proposed predictive model, some considerations need to be made. The first concerns the exuberant discrepancy between the number of negative and positive samples for training the neural network. Since the model is exposed to few positive examples, there tends to be a relative restriction of sensitivity, which was observed especially in the evaluation on the test set. However, as long as low false-positive rates are maintained (which was observed in both validation and testing), this does not compromise the validity of the screenings performed, even though potentially active compounds may not be identified due to greater structural divergence from the training active compounds. The different nature of the test data compared to the training and validation data should also be noted. Although interchangeability between active site binding and inhibitory activity was assumed for predictive evaluation purposes, it is not possible to infer that all compounds that demonstrated binding (covalent or non-covalent) to the active site would, in an assay, demonstrate inhibition of sufficient appreciable magnitude to be classified as active for this property. Still, the high sensitivity in both sets adds robustness to the predictions made in the screening performed by the model.
Since deep learning models are a highly data-driven approach, the major limitation of this study was the low availability of bioassay data of compounds positive for 3CLpro inhibition activity. This limitation even conditioned the inclusion of data from different assays – although integrated in the same project – with differences in quantification strategies and methodological orientation: while the training data and the negative test data came from a screening assay, the positive data from the test set came from a confirmatory assay. The binarization of the predicted variable was a strategy adopted to deal with this limitation; moreover, despite this, the model achieved a great performance in the test set, with good sensitivity and specificity values, indicating that there was an adequate learning of patterns.
In conclusion, property prediction with deep learning models, in an approach based on the SAR, shows great potential to screen repurposing candidate drugs for the treatment of COVID-19, especially from the search for antiviral mechanisms in compounds with already established actions potentially beneficial in the pathophysiological context of the disease. As an illustration of this potential, the present work reported four anti-inflammatory agents, two anticoagulants, and one respiratory agent as potential inhibitors of the main protease of SARS-CoV-2. These data provide possible directions for in vitro and in vivo research, which are indispensable for the validation of their results.