Servicios Personalizados
Revista
Articulo
Indicadores
-
Citado por SciELO
-
Accesos
Links relacionados
-
Similares en SciELO
Compartir
Revista mexicana de fitopatología
versión On-line ISSN 2007-8080versión impresa ISSN 0185-3309
Rev. mex. fitopatol vol.39 no.1 Texcoco ene. 2021 Epub 07-Mayo-2021
https://doi.org/10.18781/r.mex.fit.2009-3
Review articles
Omics sciences potential on bioprospecting of biological control microbial agents: the case of the Mexican agro-biotechnology
1 Escuela de Agronomía, Universidad De La Salle Bajío, Avenida Universidad 602. Colonia Lomas del Campestre C.P. 37150, León, Guanajuato, México;
2 Centro Nacional de Recursos Genéticos-INIFAP. Boulevard de la Biodiversidad # 400. Rancho Las Cruces. C.P. 47600. Tepatitlán de Morelos, Jalisco, México;
3 Universidad Autónoma Metropolitana Unidad Xochimilco, Xochimilco, Calzada del Hueso 1100, Coapa, Villa Quietud, Coyoacán, C.P. 04960, CDMX, México;
4 Instituto Tecnológico de Sonora, 5 de Febrero 818 Sur, Colonia Centro, C.P. 85000. Ciudad Obregón, Sonora, México;
5Centro Universitario de los Altos, Universidad de Guadalajara. Avenida Rafael Casillas Aceves No. 1200, Tepatitlán de Morelos, Jalisco, México;
6 Instituto Nacional de Investigaciones Forestales, Agrícolas y Pecuarias. Campo Experimental Norman E. Borlaug-INIFAP, Norman E. Borlaug Km. 12, C.P. 85000, Ciudad Obregón, Sonora, México;
7 INECOL, Carretera antigua a Coatepec 351, Colonia El Haya. C.P. 91073, Xalapa-Enríquez, Veracruz, México;
8 Posgrado en Manejo Sustentable de Recursos Naturales, Universidad Intercultural del Estado de Puebla, Calle Principal a Lipuntahuaca S/N, Lipuntahuaca, CP 73475, Huehuetla, Puebla, México;
At present, studies of biological control agents of microbial origin (BCA-M) have mainly focused on their taxonomic characterization, through the use of conventional molecular markers, and the in vitro evaluation of modes of action, or under greenhouse conditions, but limitedly under field conditions. Furthermore, recent bioprospecting studies of BCA of microbial origin mainly focus on Trichoderma, Paecilomyces, Beauveria, Pseudomonas, and Bacillus. Even when the research developed in Mexico on this topic has been active during the last years, the development and innovation of greater variety of registered and currently commercialized biopesticides need to be improved. In this context, the use of cut-edge techniques in the era of omics sciences (genomics, transcriptomics, and metabolomics) focused on the correct taxonomic affiliation of BCA-M, as well as their modes of action and ecology in agro-ecosystems, will expand the bioprospecting and extensive use of these BCA in a more efficient, biosafety, and cost-effective manner. In the framework of the international celebration of plant health, this review critically analyzes the knowledge status of the aspects that limit the bioprospecting and extensive use of BCA-M, mainly in Mexico, from the application of omics sciences for the identification, selection, and study of action mechanisms of those agents until the dissemination and socialization of the generated scientific knowledge. The foregoing is intended to promote reflection on this field of knowledge and encourage the new generation BCA-M with a holistic and systemic vision for the benefit of sustainable and resilient agriculture.
Key words: Bioplaguicides; taxonomy; genomic; transcriptomic; metabolomic
Actualmente, los estudios sobre agentes de control biológico de origen microbiano (ACB-M) generalmente están enfocados en la caracterización taxonómica mediante el uso de marcadores moleculares convencionales y en evaluar la capacidad antagónica/mecanismos de acción in vitro, en invernadero y eventualmente bajo condiciones de campo. Los ACB-M se centran principalmente en cepas de Trichoderma, Paecilomyces, Beauveria, Pseudomonas y Bacillus. Aunque la investigación en México en este campo ha sido muy activa en los últimos años, el desarrollo e innovación de una mayor diversidad de bioplaguicidas registrados y comercializados puede ser potenciada. En este contexto, el uso de técnicas vanguardistas en la era de las ciencias ómicas (genómica, transcriptómica, y metabolómica) enfocadas a la correcta afiliación taxonómica de los ACB-M y en el estudio de mecanismos de acción y comportamiento agroecológico es determinante para la bioprospección y uso extensivo de estos ACB-M de manera eficaz, biosegura y costo-efectiva. En el marco de la celebración internacional de la sanidad vegetal, la presente revisión analiza críticamente el estado del conocimiento y de aquellos aspectos que limitan la bioprospección y el uso extensivo de ACB-M con énfasis en México, desde la aplicación de las ciencias ómicas para la identificación, selección y estudio de los mecanismos de acción de dichos agentes hasta la difusión y socialización del conocimiento científico generado. Se pretende promover la reflexión sobre este campo del conocimiento e incentivar la nueva generación ACB-M con una visión holística y sistémica en beneficio de una agricultura sustentable y resiliente.
Palabras clave: Bioplaguicidas; taxonomía; genómica; transcriptómica; metabolómica
Crops are exposed to abiotic and biotic factors that can negatively impact their productivity, i.e., floods, droughts, degraded soils, and phytopathogens. Phytopathogens are responsible for up to 40 % of crop yield reductions (Valenzuela-Ruiz et al., 2020). Along with this, the growing demand for food fueled by population increases has led to the modification of agricultural practices to mitigate the losses caused by phytopathogens, without lowering the nutritional quality and safety of the products grown (FAO, 2018). A widely used strategy to address these aspects has been the use of synthetic pesticides, which increased 97.7% worldwide from 1992 (1.30 kg ha-1) to 2016 (2.57 kg ha-1) (FAO, 2018); in Mexico, the use of synthetic fungicides increased 76.9% (from 1.08 to 1.91 kg ha-1) in the same period (Roser, 2019). Reports show that only 0.1% of the applied synthetic pesticides reach the crop of interest (Gill and Garg, 2014), a fact that creates serious economic and environmental impacts, such as soil and aquifer contamination with recalcitrant wastes, soil, chemical, and microbial degradation, and biodiversity loss (de los Santos-Villalobos et al., 2018).
In this context, phytopathogen management in cropping systems must be extensively and comprehensibly addressed to be consistent with Goal 2, end hunger, of the 2030 Agenda for the Sustainable Development for Latin America and the Caribbean. Goal 2 establishes the need to concentrate efforts to achieve food security, improve nutrition, and promote sustainable agriculture (ONU, 2018). Mexico has collaterally contributed to these goals since 1940 when the first studies and applications of biological control began and academic programs were established to promote an integrated plant health management (Gutiérrez-Samperio, J. Personal communication. 2019). Biological management of phytopathogens is defined as “the use of beneficial organisms to reduce the negative effects caused by phytopathogens, through their antagonistic actions, either by direct actions of gene recognition or indirectly through metabolites or products derived from them” (Valenzuela-Ruiz et al., 2020). In a broader sense, biological management was initially promoted through campaigns implemented by government agencies, where predator insects, parasitoids or sterile insects were used, followed by the inclusion of entomopathogenic fungi (Beauveria bassiana and Metarhizium), filamentous fungi and bacteria, to control important pests nationally (Arredondo-Bernal and Rodríguez-del Bosque, 2015).
The use of biological control agents of microbial origin (BCA-M) in Mexico is an alternative to complement the conventional control of phytopathogens using synthetic pesticides (Arredondo-Bernal and Rodríguez-del Bosque, 2015). For example, in Mexico, the area sown in 2017 was approximately 32.4 million hectares, where the main crops were maize, sorghum, bean, coffee, sugarcane, and wheat (INEGI, 2017); the main phytopathogen genera reported in those crops were Fusarium, Colletotrichum, Leveillula, Botrytis, Rhizoctonia, Pythium, Phytophthora, Pseudomonas, Candidatus Liberibacter asiaticus, Leifsonia, and Xanthomonas, and the BCA-M that were most used for controlling these pathogens were the fungal species Trichoderma harzianum, Paecilomyces lilacinus, P. fumosoroseus and Beauveria bassiana, and the bacterial species Bacillus subtilis, B. pumilus, B. amyloliquefaciens, B. licheniformis and B. thuringiensis (García-Juárez et al., 2016; Villarreal-Delgado et al., 2017; Delgado-Ortiz et al., 2019). Although researches on BCA are significant (Tables 1 and 2), sometimes, no detailed information on the results of their application to nationally important crops in the field are published or are included in technical reports with restricted access and limited to describing crop yield increases and/or phytopathogen inhibition.
Identifying new agents and/or understanding the plant-pathogen-BCA of microbial origin interaction (under field conditions), as well as conducting robust studies of the polyphasic taxonomic affiliation (consensus classification based on the integration of all available phenotypic and genotypic data), action and ecology mechanisms of those BCA in the agroecosystems, using the recent advances in omics sciences, are essential for registration, marketing, innovation and success of formulated biopesticides. For this reason, in the framework of the international celebration of Plant Health, the objective of this review is to describe the knowledge status and analyze the aspects which limit the bioprospection and extensive use of BCA-M in Mexico, cutting-edge genomic strategies inherent to the omics sciences focused on the identification, selection and study of the mechanisms of action of those agents, and the dissemination and socialization of the scientific knowledge gained to enhance agro-biotechnological innovation and biopesticides success.
Biological control agents of microbial origin (BCA-M) and biopesticides in Mexico. The identification and introduction of natural enemies in economically important phytopathogens in Mexico became relevant in the 1940s due to the need to develop efficient and sustainable management strategies. In the more than 80 years of using BCA-M in our country (Bernal and Quezada, 1999), 230 bioproducts from 40 companies registered by COFEPRIS (https://www.gob.mx/cofepris) are available in the national market. However, some of these products have a limited number of active ingredients at the taxonomic group level (fungi, bacteria y/o viruses), or microbial genus/species for controlling the causal agents of diseases in plants that are cultivated in Mexico (Table 1). This reflects a limited availability of bioproducts to be used in the Mexican fields despite the efforts made and the research conducted in our country. This fact could be associated with the limited support to the scientific sector to conduct systemic research, bioprospecting new BCA-M, and obtaining patent registration of biotechnological products, as well as with the limited organizational structure at the institutional level to establish departments specialized in patent and licensing processes. The lack of financial resources has often impacted the development of partial research, mainly in vitro, from collections with limited biological and regional representation. On the other hand, there is no access to documentary information to scientifically support their effectiveness, nor statistical data of their production, consumption and success at the national level (De León and Mier, 2010), with the exception of products such as Fungifree®, which is an effective biofungicide for managing foliar diseases such as anthracnose (C. gloeosporioides, C. acutatum and C. fragariae), powdery mildew (L. taurica, E. chichoracearum and S. humili) and gray mold (B. cinerea) (Galindo et al., 2015), and the studies conducted by Centro Nacional de Referencia de Control Biológico SAGARPA-DGSV for controlling Diaphorina citri and lobster with entomopathogenic fungi (Ayala-Zermeño et al., 2015; Gallou et al., 2016).
Table 1 Biological control products of microbial origin in Mexico, registered by COFEPRIS (https://www.gob.mx/cofepris).
Ingrediente Activo | Producto comercial | Uso agrícola |
---|---|---|
Bacterias | ||
Acetobacter lovaniensis | Q-Bacter | Bio-bactericida |
Bacillus amyloliquefaciens | Serifel/Serenade Optimum / Stargus | Bio-fungicida |
B. subtilis | Fungifree-Ab/ Agrobacilo/ Serenade As/ Serenade Max/ Bacillios G / Baktillis / Serenade/ Rador/ Vici Active/ Fungi-Q/ Fungisei/ Protec-Root/ Cx-9030 | Bio-fungicida |
B. stearothermophilus | Bacillus 1537 / Labsa Bst / Bacillus Thermo | Bio-fungicida |
B. thurigiensis | Dipel 2x /Xentari Grd/ Delta Bt/ Bt-K/ Dipel-2x/ Phc Beretta/ Larvix/ Thuricide Ph/ Newbt-2x Wp/ Mvp/ Xentari/ Lepinox 15 Wdg/ Crymax Gda/ Phc Condor Wp/ Dipel 2x/ Turinsil | Bio-insecticida |
B. pumilus | Sonata As | Bio-fungicida |
Chromobacterium subtsugae | Grandevo | Bio-insecticida |
Streptomyces spp. | Blite Free | Bio-fungicida |
Hongos | ||
Beauveria bassiana | Bea-Sin/ Atento/ Bioin Dalife/Cercon Es/ Naturalis L | Bio-insecticida |
Metarhizium anisopliae | Meta-Sin/ Biomett/ Meta-Noc/ Spectrum Meta | Bio-insecticida |
Myrothecium verrucaria | Ditera Es/ Ditera Df | Bio-nematicida |
Paecilomyces fumosoroseus | Pae-Sin/ Bioamin-Insect-1/ Pfr-97 20% Wdg/ Nofly Wp | Bio-insecticida |
P. lilacinus | Lila-Sin / Chimal/ Bioact Wg/ Biostat/ Nemafin/ Spectrum Pae | Bio-nematicida |
P. variotii | Nemaquim / Nemakill / Pha.Da / Nem P.B. / Sinnemakill / Nemphada / O.B Golf | Bio-nematicida |
Pochonia chlamydosporia | Genexis Ph | Bio-nematicida |
Trichoderma harzianum | Tricho-Sin/ Labrador/ Trianum P/ Phc T22/ Spectrum Trico-Bio/ Bioamin-Fung-2/ Rootshield Plus Wp | Bio-fungicida |
T. lignorum | Mycobac | Bio-fungicida |
T. viride | Funqui | Bio-fungicida |
Verticillium lecanii | Verti-Sin/ Eday | Bio-insecticida |
Mezclas | ||
Bacillus subtilis, Rhodotorula minuta | Fungifree-Ab Plus | Bio-fungicida |
B. subtilis, Bacillus spp. Torulopsis inconspicua | Obiettivo | Bio-fungicida |
B. licheniformis, T. harzianum | Biowall | Bio-fungicida |
Bacillus spp., aceite de clavo y neem | Roya Out | Bio-fungicida |
B. subtilis, Trichoderma spp., P. lilacinus, quitosano, bicarbonato de potasio | Nemaxxion Biol | Bio-fungicida |
B. bassiana, Nomurea rileyi , aceitedeneem, B. thuringiensis var. Kurstaki y var. Israelensis | Larbiol 2X | Bio-insecticida |
B. bassiana, Nomuraea rileyi, Metarhizium anisopliae, Verticillium lecanii, P. fumosoroseus, concentrado oleico | Biomaxx Duo | Bio-insecticida |
T. harzianum, T. viride, T. fasciculatum | Juq | Bio-fungicida |
P. fumosoroseus, M. anisopliae, B. bassiana | Tri-Sin | Bio-insecticida |
M. anisopliae, B. bassiana | Biomabb/ QUIM | Bio-insecticida |
P. lilacinus, B. firmus | Arrecife | Bio-nematicida |
P. lilacinus, B. subtilis, Pseudomonas fluorescens, extracto de ruezno de nuez, Yucca schidigera, guiche de lechuguilla, cáscara de nuez, higuerilla, gel de nopal, chitosan | Nemmax | Bio-nematicida |
Furthermore, one of the main constraints for technology transfer and extensive use of bioproducts based on BCA-M is the insufficient publication and dissemination of scientific research conducted by different universities and research centers in the country, even after the product’s patent has been obtained (Bernal and Quezada, 1999). In this regard, the important role of the Mexican Journal of Phytopathology or RMF, for its acronym in Spanish (https://www.rmf.smf.org.mx/), must be recognized, because during the past eight years, different researchers have published about 50 scientific papers (23.7% of the total number of the published articles) reporting aspects related to the use of BCA-M, i.e., scientific articles, review articles, phytopathological notes and phytopathological reports (Table 2). Likewise, records provided by Scielo Analytics (https://analytics.scielo.org/) show that of the 100 articles of the JMP that are most consulted online, 24% correspond to subjects related to BCA-M, of which the following stand out: the Bacillus genus as a biological control agent and its implications in agricultural biosafety (Villarreal-Delgado et al., 2017), the action mode of Candida oleophila for managing Penicillium expansum and Botrytis cinerea (Guerrero et al., 2011), and “witch broom” biocontrol with Trichoderma spp. in mango crops under field conditions (Michel-Aceves et al., 2013), just to name a few examples.
In this regard, to strengthen the scientific research and enrich the functional range of bioproducts registered and sold in Mexico, it is essential to incorporate new cutting-edge and robust tools into scientific studies of BCA-M, especially about their polyphasic taxonomic affiliation, action mode in vitro, and under greenhouse and field conditions, as well as their interaction with biotic and abiotic factors. This would lead to the establishment of cost-effective production, formulation, scaling and innovation systems (Galindo et al., 2013; Serrano-Carreón et al., 2010), which, in turn, would help governmental agencies and professionals involved in agricultural production make an efficient, safe, and rigorous technology transfer of these bioproducts to farmers.
Taxonomic affiliation of BCA-M. The molecular taxonomic study of BCA-M has been conventionally undertaken using the 16S rRNA gen for prokaryote taxonomic affiliation, because i) it is present in that group of organisms and often exists as a multigenic family; ii) its function has not changed over time, thereby suggesting that slow changes occur in its sequence during the evolutionary course; and iii) its length (1500 bp) is great enough for technical and bioinformatic purposes but it is difficult to identify bacterial species because of its limited genetic diversity (Aguilar-Marcelino et al., 2020). Likewise, the ITS region (internal transcribed spacer) in eukaryotes, which is made up of the ITS1, 5.8S rRNA gene and ITS2, is highly conserved at the genus level, whose length (650-1,100 bp) and limited genetic diversity among species hampers their correct differentiation and taxonomic affiliation. The BCA-M taxonomic affiliation is based on the use of molecular techniques (RAPDs, ISSR, RFLP, AFLP) and/or PCR for rapid detection using marker genes, such as 16S rRNA, 28S rRNA, ITS, β-tubulin, RPB2, elongation factor, SCAR (Mazrou et al., 2020; Hassan et al., 2019). The taxonomic resolution at the species or subspecies level (i.e., haplotypes) will depend on the preferred molecular technique, restriction enzymes, and selected genes or regions.
Table 2 Scientific articles focused on biological control that have been published in the Mexican Journal of Phytopathology (https://www.rmf.smf.org.mx/) Period: 2012 al 2020.
Agente de control biológico | Análisis empleado para identificación | Cultivo | Agente fitopatógeno | Condiciones de evaluación | Mecanismo de acción propuesto | Herramienta empleada para detección de mecanismo de acción | Referencias |
---|---|---|---|---|---|---|---|
Bacillus y Burkholderia | Secuenciación del gen 16S ARNr | - | Colletotrichum gloeosporioides | In vitro | Se propone la excreción de metabolitos secundarios | Confrontación dual, estimación cualitativa con escala de 4 niveles de antagonismo y cuantitativo mediante análisis de imagen | Macedo-Castillo et al., 2012 |
Paecilomyces lilacinus | Claves taxonómicas | Solanum tuberosum | Nematodos de vida libre | In vitro | Parasitismo | Potencial parasítico | Carrión y Desgarennes, 2012 |
Pochonia hlamydosporia | Claves taxonómicas | Phaseolus vulgaris | Nacobbus aberrans | In vitro einvernadero | Parasitismo | Potencial parasitismo, colonización de raíces y prueba de efectividad | Franco-Navarro et al., 2013 |
Trichoderma | Claves taxonómicas | Mangifera indica | Fusarium oxysporum y F. subglutinans | Campo | Parasitismo | Severidad de la enfermedad | Michel-Aceves et al., 2013 |
Bacillus, Pseudomonas, Trichoderma y Penicillium | Claves taxonómicas, medios selectivos y pruebas bioquímicas | - | Rhizoctonia solani y F. oxysporum f sp. lycopersi | In vitro | Antibiosis, parasitismo | Confrontación en placa y suelo estéril | Rodríguez- Millán et al., 2013 |
Trichoderma y Bacillus | Claves taxonómicas | Agave tequilana weber var. Azul | Fusarium | Vivero | Parasitismo, resistencia sistémica, competencia, producción de enzimas líticas | Potencial parasitismo, colonización de raíces y supresión del agente causal de la enfermedad | Tlapal-Bolaños et al.,2014 |
B. subtilis y T. harzianum | Uso de microorganismos de productos comerciales | Capsicum annuum | Phytophthora capsici | Invernadero | Supresión de la enfermedad | Antagonismo, colonización y supresión del agente causal de la enfermedad | Lozano-Alejo et al., 2015 |
C. musae, C. loeosporioides e Idriella lunata | Claves taxonómicas | - | Staphylococcus aureus, Streptococcus pneumoniae, S. epidermis, Escherichia coli y Pseudomonas aeruginosa | - | Antibiosis de metabolitos secundarios | Evaluación mediante sensidiscos con los extractos fúngicos (halos de inhibición) | Lagunes-Castro et al., 2015 |
Actinomicetos | Claves taxonómicas | Solanum tuberosum | Ralstonia solanacearum, Pectobacterium carotovorum, P. infestans, Fusarium y R. solani | In vitro | Antibiosis, enzimas líticas, | Evaluación de extractos de actinomicetos mediante difusión en agar, halos de inhibición, confrontación dual, concentración mínima inhibitoria | Pérez-Rojas et al., 2015 |
T. viride y B. subtillis | Uso de microorganismos de productos comerciales | - | Sclerotinia minor, S. sclerotiorum, y S. cepivorum | In vitro | Competencia, micoparasitismo y antibiosis | Confrontación dual | Pérez-Moreno et al., 2015 |
Bacillus y Trichoderma | Claves taxonómicas y secuenciación del gen 18S ARNr y 16S ARNr | - | F. oxysporum, Botrytis cinerea, Penicillium crustosum, Aspergillus nidulans y Alternaria alternata | In vitro | Parasitismo, antibiosis, producción de enzimas líticas | Confrontación dual | Rios-Velasco et al., 2016 |
Bacillus spp. | Claves taxonómicas | Capsicum chinense | F. equiseti y F. solani | In vitro e invernadero | Competencia, producción de metabolitos y enzimas líticas, promoción del crecimiento vegetal, inducción de resistencia sistémica | Confrontación dual, inducción de resistencia, severidad de la enfermedad | Mejía-Bautista et al., 2016 |
Trichoderma | Claves taxonómicas y secuenciación del gen ITS1, ITS4 y tef1α | - | P. infestans | In vitro | Parasitismo | Confrontación dual | García-Núñez et al., 2017 |
Hongosmicorrícicosarbusculares | Uso de microorganismos de productos comerciales | Agave cupreata | F. oxysporum | Invernadero | Competencia por sitio de colonización, cambios en la composición de exudados radiculares, inducción de resistencia, promoción del crecimiento vegetal | Colonización de raíces y severidad de la enfermedad | Trinidad-Cruz et al., 2017 |
Saccharicola | Microorganismos provenientes de trabajos previos | Capsicum annuum | P. capsici, F. oxysporum y R. solani | In vitro e invernadero | Parasitismo, competencia, producción de enzimas, antibiosis | Confrontación dual, prueba de parasitismo de Riddell, microscopia de barrido, incidencia y severidad de la enfermedad | Uc-Arguelles et al., 2017 |
Trichoderma y Bacillus | Microorganismos provenientes de trabajos previos | M. domestica | P. cactorum | Invernadero | Promoción del crecimiento vegetal, producción de fitohormonas, inducción de resistencia | Confrontación, severidad de la enfermedad | Ruiz-Cisneros et al., 2017 |
Lecanicillium, alcarisporium, Sporothrix y Simplicillium | Claves taxonómicas | - | Hemileia vastatrix | In vitro | Micoparasitismo | Confrontación dual, parasitismo | Gómez-De la Cruz et al., 2017 |
B. amyloliquefaciens y B. thuringiensis | Claves taxonómicas y secuenciación del gen 16S ARNr | - | P. capsici | In vitro e invernadero | Antibiosis, producción de lipopéptidos, promoción del crecimiento vegetal | Inhibición germinativa de zoosporas con inoculo bacteriano y filtrados, eficacia de biocontrol | Ley-López et al., 2018 |
B. subtilis | AFLP (EcoR y MseI) | - | R. solani | In vitro | Antibiosis, producción de enzimas líticas , | Sobrenadante difusión en placa (metabolitos antagonistas), placas microtituladoras BIOLOG (diversidad metabólica) | Jiménez- Delgadillo et al., 2018 |
Trichoderma y Bacillus | Claves taxonómicas y secuenciación del gen 18S ARNr, ITS1 e ITS4). Bacillus proviene de trabajos previos | S. lycoper- sicum | A. solani, F. oxysporum y P. infestans | In vitro | Producción de enzimas líticas, metabolitos promotores de crecimiento vegetal, antibiosis, inducción de resistencia, producción de lipopéptidos | Confrontación dual, tipo de antagonismo (escala de Bell) | Ruiz-Cisneros et al., 2018 |
P. fluorescens | Claves taxonómicas y secuenciación del gen 16S ARNr | S. lycoper- sicum | A. alternata | In vitro e invernadero | Producción de compuestos extracelulares inhibidores enzimáticos, antibiosis | Difusión en agar de extractos, germinación de conidios y crecimiento micelial, incidencia y severidad de la enfermedad | Rodríguez- Romero et al., 2019 |
Simplicillium y Lecanicillium | Secuenciación del gen 18S ARNr, ITS1 e ITS4 | - | Hemileia vastatrix | In vitro | Parasitismo | Confrontación dual, parasitismo | García-Neváreze Hidalgo- Jaminson, 2019 |
T. harzianum | No se menciona | Carya illinoensis | Phymatotrichopsis omnivora | vivero | Antagonismo | Desinfestación reductiva | Samaniego- Gaxiola et al., 2019 |
T. harzianum y B. subtilis | Microorganismos provenientes de trabajos previos | Arachis hypogaea | Aspergillus flavus, Fusarium sp., Sclerotinia minor y Thecaphora frezzi | In vitro y campo | Producción de enzimas líticas, promoción del crecimiento vegetal | Inoculación de sustrato estéril, incidencia de enfermedad, crecimiento de plantas, peso seco. | Illa et al., 2020 |
B. amyloli- quefaciens y B. subtilis | Claves taxonómicas, pruebas bioquímicas, y metabolismo (tarjeta VITEX 2) | - | S. cepivorum | In vitro | Producción de metabolitos, sideróforos, enzimas líticas, promoción del crecimiento vegetal, tolerancia a estrés, antibiosis | Confrontación dual, actividad del ACC desaminasa, tolerancia a NaCl y prueba colorimétrica para producción de sideróforos y AIA | Ocegueda- Reyes et al., 2020 |
The correct taxonomic affiliation is decisive for bioprospecting biosafe BCA-M, considering that several genera have species with antagonistic activity against phytopathogens but may also have other species that cause diseases in plants, animals and/or humans. For example, Bacillus is one of the bacterial genera that has been most reported and studied worldwide because of its biological control capacity. The B. cereus group has been extensively studied and is made up of, at least, eight species that are closely related: B. anthracis, B. cereus, B. thuringiensis, B. mycoides, B. pseudomycoides, B. weihenstephanensis, B. cytotoxicus and B. toyonensis. However, the members of this group have a significant impact on human health, agriculture, and the food industry, i.e., B. anthracis is the etiological agent of anthrax, an obligated pathogen that poses a threat to human and herbivores health, while B. thuringiensis is a biological management agent which is extensively used and recognized as a safe biopesticide in the world (Liu et al., 2015). On the other hand, B. cereus is an opportunistic pathogen that often causes food poisoning and has recently been reported as an emerging phytopathogen. At the intraspecific level, members of the B. cereus group have sequences of the 16S rRNA gene that are very similar and highly conserved genomes (Ehling-Schulz et al., 2019), a fact that makes their correct taxonomic affiliation difficult. Another group of taxonomic importance with a molecular approach is the Burkholderia genus, due to biochemical, biological, and morphological characterization restrictions (Espinosa-Victoria et al., 2020; Serret-López et al., 2021; de los Santos-Villalobos et al., 2018). In recent years, the introduction of various next-generation sequencing strategies and platforms (NGS), as well as genomic, phylogenomic, and bioinformatic tools, have significantly contributed to a better understanding of the microorganism’s evolution and interaction with their environment (Jagadeesan et al., 2019). These tools have also made it possible to study microbial strains at the genomic level, which has strengthened their correct taxonomic affiliation and identification of the genes involved in colonization and adaptation to the host, and their mechanisms of action, among other aspects (Figure 1).
Genomic studies for the taxonomic affiliation of BCA of microbial origin (BCA-M). At present, the robust taxonomic affiliation of microorganisms is based on the extraction, sequencing, and analysis of their genome, partial or total, supported by morphological, physiological, and metabolic traits (Figure 2). For this, sequencing high-quality DNA of the microorganism of interest and validating the obtained data through a quality control process are decisive factors, because reads of DNA without the required quality can be excluded in subsequent analysis (Xi et al., 2019). FastQC is a digital tool designed to perform numerous quality control checks of the sequences that are obtained (Andrews, 2010). Then, the adapters must be trimmed, and low-quality data of the obtained genomic information removed using programs such as Trimmomatic v0.36 (Bolger et al., 2014) and Cutadapt v1.18 (Martin, 2011). The genome is assembled using programs such as SPAdes v3.14.1, which assembles non-ribosomal peptides associated with biological control mechanisms (Bankevich et al., 2012), hybrid SPAdes (Antipov et al., 2016), Velvet v1.2.10 (Zerbino, 2008), IDBA-UD v1.1.1 (Peng et al., 2012), Canu (Koren et al., 2017) and SOAPdenovo2 (Luo et al., 2012). Once the genome has been assembled, the obtained contigs are rearranged based on a reference genome with the Mauve contig Mover 2.4.0 program (Darling et al., 2004). Finally, the genome annotation is carried out to identify the encoding sequences and associate them with a function or identify hypothetical proteins. For this, the National Center for Biotechnology Information (NCBI) provides an automatic prokaryotic genome annotation tool. Similarly, RAST and xBASE2 are web servers used to annotate genomes of bacteria and archaea (Aziz et al., 2008; Chaudhuri et al., 2008). Genome contamination can be quantified using different software packages, such as checkM and Quast, for prokaryotes and eukaryotes (Gurevich et al., 2013; Parks et al., 2015). Recently, bioinformatics tools in silico have been developed for taxonomic affiliation of bacterial species (Figure 2), of which the most important is the overall genome relatedness indices (OGRI), such as the average nucleotide identity (ANI) and the genome-to-genome distance calculator (GGDC), to replace the DNA-DNA hybridization standard (DDH) (Richter et al., 2016). This has been used to study prokaryotes only since there is no equivalent OGRIs for fungal strains (Raja et al., 2017).
ANI is widely used to establish similarity between two prokaryote species at the genomic level (Yoon et al., 2017) and is defined as the average percent of nucleotide sequences identity of orthologous genes shared between two genomes (Sentausa and Fournier, 2013). The ANI value of the genome under study corresponds to the average of the identity values among all the pairs of orthologous fragments between two genomes. The ANI value used to affiliate a strain to a bacterial species previously described is ≥ 95-96% (Varghese et al., 2015; Moriuchi et al., 2019).
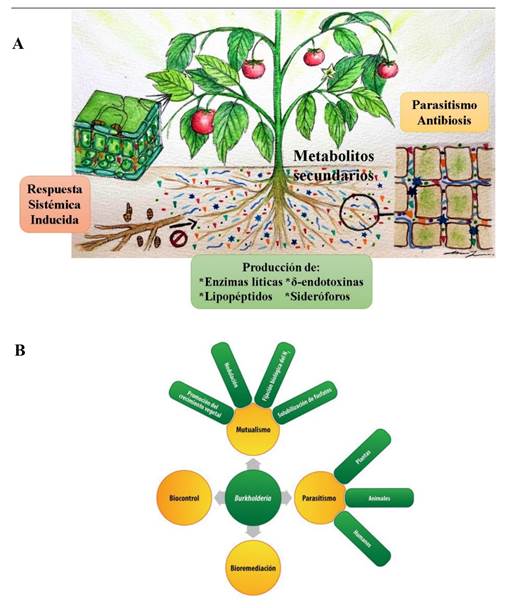
Figure 1 A. Mechanisms of action that have been most studied and reported in biological control agents of microbial origin (BCA-M) (Source: Authors). B. Multiple biotic-abiotic functions that can be associated with an BCA-M, Burkholderia case (Source: Espinosa-Victoria et al., 2020).

Figure 2 Workflow of the genomic and metabolic methodological processes used in taxonomic research and to establish the mechanisms of action of biological control agents of microbial origin (BCA-M) to suppress phytopathogens.
On the other hand, unlike ANI (index similarity type), GGDC (http://ggdc.dsmz.de/) is an OGRI based on the distance ratio which enables the alignment of the genome of interest with one of reference; then a set of high-scoring segment pairs (HSP) is obtained, known as “intergenomic coincidences”, from which specific distance formulae are calculated to transform the HSP data to a GGDC value (Meier-Kolthoff et al., 2013), using a >70% GGDC value to taxonomically affiliate a strain to a bacterial species previously reported.
Currently, the use of tools and strategies focused on robust and comprehensive BCA-M taxonomic affiliation (based on genome sequencing and polyphasic studies) is experiencing a boom in Mexico. For example, one of these studies was recently published by de los Santos-Villalobos et al. (2019), who reported a new bacterial species with biological control capacity against Bipolaris sorokiniana, an emerging agent that causes spot blotch in wheat in the Yaqui Valley, Mexico. Initially, this bacterial strain (TE3T) was affiliated to the Bacillus genus based on the partial sequencing of the 16S rRNA gene (Valenzuela-Aragón et al., 2018). Then, Villa-Rodriguez et al. (2019) proposed its taxonomic affiliation to the B. subtilis species by sequencing the whole 16S rRNA gene. Finally, the sequencing and study of the whole genome, the OGRIs analysis (ANI and GGDC), and the polyphasic characterization of strain TE3T allowed a robust taxonomic affiliation of this BCA to a new bacterial species, termed B. cabrialesii (de los Santos-Villalobos et al., 2019). Bacillus cabrialesii TE3T inoculation in wheat plants infected by B. sorokiniana mitigated the disease by reducing the 3-5 severity scale of leaf infection and the density of lesions to 3.06 ± 0.6 cm-2 leaves compared to plants inoculated with the phytopathogen (8-9 and 6.46 ± 1.46 lesions cm-2).
Study of the action mechanisms in BCA-M. In recent decades, studies of the effect of BCA-M in phytopathogens management have been carried out. This has been useful to elucidate the different mechanisms used by these agents to reduce the growth, development, and infection of phytopathogens in cultivated plants. Among the interaction mechanisms with suppressive effects that have been most studied and reported are i) mycoparasitism; ii) antibiosis; iii) lytic enzymes activity; iv) siderophore biosynthesis; v) production of δ-endotoxins; vi) lipopeptides biosynthesis; and vii) induced systemic response (Figure 1A). However, since the action of the BCA-M involves non-parasitic phases that are essential for their adaptation to the edaphic or endophytic environment and ensure their suppressive activity, they must be systematically studied to design soil, substrate, or plant bioremediation strategies (Figure 1B).
In Mexico, based on the contributions published by the Mexican Journal of Phytopathology and other national and international scientific journals, there are many scientific studies focused on the selection and evaluation in vitro of promising BCA-M. Although to a lesser extent, the specific mechanisms of action previously mentioned have been also explored (Table 2 and Figure 1). However, the innovation of biopesticides developed in Mexico and other countries requires that new BCA along with the mechanisms responsible for the activity observed be identified, both in new strains and in those that have been already reported, because the mechanisms or metabolites used by those BCA are not necessarily responsible for the observed effect or are directly related to their bioactivity in the field. Consequently, state-of-the-art and integrating strategies that allow a better understanding of the mechanisms of action of the BCA-M under field conditions must be implemented. This would increase the probabilities of success in implementing these agents in the agricultural sector, thereby optimizing the production processes, scaling new bioproducts, and promoting the innovation of those already registered and available on the market.
Genomic studies of BCA-M to identify potential mechanisms of action. So far, several strategies and platforms have been developed to identify clusters of genes in genomes associated with biological control, especially those related to non-ribosomal peptide synthetases (NRPS) and polyketide synthases (PKS) (Figure 2). These secondary metabolites exhibit a significant variety of biological activities, and many of them are clinically valuable antimicrobial, antifungal, antiparasitic, antitumor, and immunosuppressant compounds (Ansari et al., 2004). Among the tools to identify those clusters of genes in the genome of BCA of bacterial origin are PRISM (Skinnider et al., 2017), CLUSEAN (Weber et al., 2009), RIPPMiner (Agrawal et al., 2017), and for fungal genomes, SNAP (Jhonson et al., 2008), Augustus (Keller et al., 2011) and GeneMark-ES (Borodovsky and Lomsadze, 2011). However, the AntiSMASH 5.0 and BAGEL4 tools have been widely reported and used for these purposes, but the identification of genes associated with the BCA-M activity using these tools is predictive, and, for this reason, their expression and function in vivo must be further studied and complemented with other strategies that will be later described.
AntiSMASH 5.0 uses the HMMer3 tool (http://hmmer.janelia.org/) and the translations of the amino acid sequence of all the encoding genes, which are analyzed with profile hidden Markov models (pHMM) based on alignments of multiple sequences associated with experimentally characterized proteins or proteins/domains (Blin et al., 2019). The detection stages use a filtering logic of negative and positive pHMM and their cut-off points, based on the knowledge of the minimum basic components of each type of clusters of the genes reported in the scientific literature.
On the other hand, BAGEL4 translates into six proteins [one for each open reading framework (ORF) Α, each of the encoding genes of the BCA genome that is being studied (Van Heel et al., 2018). These proteins are examined to detect the occurrence of certain protein motifs (elements conserved in an amino acid sequence that is usually associated with a specific function) and compared to a peptide database. Finally, the areas of interest (AOI) are identified and thoroughly analyzed. The ORF in AOI are first analyzed using Glimmer3 (Delcher et al., 2007). This program is set up in such a way that Glimmer3 creates a model for each defined AOI. Later, small ORF are named in the intergenic regions. The default setup for these ORFs is a minimum length of 72 bp (24 amino acid residues) and a superposition of 10 bp is allowed with the ORF of Glimmer3.
Currently, the use of these tools for the integrative approach of the potential mechanisms of action of BCA-M are being exponentially developed in Mexico. An example of this is the research of Valenzuela-Ruiz et al. (2019), who used this strategy to identify an BCA-M taxonomically affiliated, by studying its genome and OGRIs, as Bacillus paralicheniformis TRQ65. The study of the clusters of genes associated with NRPS and PKS of the B. paralicheniformis TRQ65 genome made it possible to identify nine genes associated with the lipopetide biosythesis: lichenicidin, haloduracin, bacteriocin, enterocin and sonorensin (by BAGEL4), and eight genes associated with the lipopeptide biosynthesis: bacillibactin, butirosin, lichenysin, bacitracin, and haloduracin (by antiSMASH). The functionality of those genes was validated through essays of dual confrontation against Bipolaris sorokiniana, where an inhibition area of 1.6 ± 0.4 cm was observed, which confirmed the functionality of the putative genes found in the genome of strain TRQ65, associated with biological control of phytopathogens (Villa-Rodríguez et al., 2019; Valenzuela-Ruiz et al., 2019).
Transcriptomic studies to identify potential mechanisms of action of BCA-M. The modulation in the gene expression of an organism, associated with its interaction with biotic and/or abiotic factors, is found by conducting transcriptomic studies, which are focused on the study of the gene expression, co-expression patterns, signaling cascades, and regulated molecular mechanisms during a biological condition and specific time (Aguilar-Marcelino et al., 2020).
The transcriptomic studies are highly dependent on high-quality total RNA extracted under the specific conditions of the study, followed by sample preparation and sequencing (Figure 2). The reas taken are filtered through a quality analysis using FastQC (Andrews, 2010), the adapters and low-quality reads are removed using Trimmomatic v0.36 (Bolger et al., 2014) or Prinseq (Schmieder and Edwards, 2011). Subsequently, the reads are aligned with and mapped to a reference genome (or transcriptome) to identify and quantify the regulation of the genes and find out what their allelic variants are (Rollano-Peñaloza and Mollinedo-Portugal 2017). The organisms whose genomes have no introns are processed with contiguous aligners, such as Bowtie2 (Langmead and Salzberg 2012) or BWA (Li and Durbin 2009), which are designed to align DNA. Conversely, the best option when there are genomes with introns is the use of aligners such as Tophat2 (Kim et al., 2013), Hisat2 (Kim et al., 2013), STAR (Dobin et al., 2013), GSNAP (Wu and Nacu 2010), SOAP2 (Li et al., 2009) or Kallisto (Bray et al., 2016). The assembly of transcripts and estimation of abundances is usually performed with Cufflinks and Cuffmerge (Trapnell et al., 2009), but there are also reports of transcriptomic studies using DESeq (Love et al., 2014) or HTSeq-count (Anders and Huber, 2015).
The aligned sequences can be arranged with Samtools (Li, 2011) and Picard (http://broadinstitute.github.io/picard/). This information can be used by programs such as Bedtools (Quinland and Hall, 2010) to detect single-nucleotide polymorphisms (SNP) (Rollano-Peñaloza and Mollinedo-Portugal, 2017). The appropriate analysis of the gene expression is based on a standardization due to the different genome sizes and depth variation in each sample sequencing. Therefore, when studying de novo transcriptomes, it is recommended to use RSEM software (Li and Dewey, 2011) because it also provides standardization values. Then, to identify the differentially expressed genes, a genomic annotation must be performed by aligning the sequences of the genes that were identified as significantly expressed (Camacho et al., 2009). Then, the gene families and/or their products are identified using software packages such as HMMER (Potter et al., 2018), InterProScan (Mitchell et al., 2015), and SignalP (Petersen et al., 2011). Finally, a gene ontology analysis (GO) is carried out, whereby genes are grouped by biological processes, molecular function, cell component and metabolic pathway analysis (KEGG) (Kanehisa et al., 2004) and other more complex groups (PANTHER) (Mi et al., 2013), where tools such as AgriGO (Tian et al., 2017) are used to visualize, through hierarchical trees, the GO terms that are significantly expressed. Another approach can be the use of primers or primers of specific genes that have previously shown mechanisms of action of interest to quantify the gene expression through qPCR or ddPCR. This technique avoids the transcriptomic study of an organism.
Several reports have been published worldwide about the use of transcriptomics focused on understanding the biological control mechanisms during the plant-pathogen-BCA interaction by conducting studies of the gene expression in BCA-M (in the presence of a phytopathogen in vitro or in the field); the regulation of the gene expression in the pathogen or in the host plant (in the presence of the BCA-M or its metabolites); the impact of the phytopathogens on the gene expression profiles; and the modulation of these profiles by the presence or absence of an BCA-M. For example, Duke et al. (2017) reported that Brassica napus (canola) is greatly affected by Sclerotinia sclerotiorum, the causal agent of canola stem rot. However, when Pseudomonas chlororaphis PA23 was inoculated in canola in the presence of S. sclerotiorum, the infection rate was reduced by 91%. This result was associated with the transcriptional reprogramming in canola, i.e., the plants infected with S. sclerotiorum positively regulated approximately 8000 genes, while the preventive treatment with P. chlororaphis PA23 reduced 16 times the expression of the genes previously regulated by the phytopathogen. In addition, in the absence of the pathogen, P. chlororaphis PA23 caused the regulation of defense genes in canola, which are involved in the induced systemic response and production of reactive oxygen species.
Metabolomic studies to identify potential mechanisms of action of BCA-M. As a scientific approach based on data to detect and quantify hundreds of metabolites by differential analyses (Lloyd et al., 2015), metabolomics is ideal for analyzing complex interactions at the metabolic level during plant-microorganisms-environment interactions.
The metabolomic studies are based, in the first place, on establishing the experimental condition for the study (Figure 2) by which the strategy of sample preparation will be established (focused on the type of compounds to be detected, including extractions with organic solvents or solid-phase extractions), followed by sample preparation, either by concentration or purification (Mhlongo et al., 2018). Then, the separation and detection of metabolites of interest is carried out using gas chromatography (GC), liquid chromatography (LC) or capillary electrophoresis (CE) coupled to mass spectrometry (MS) (Naz, 2014). CE separates the compounds depending on the charge and size and provides high-resolution power. CE-MS is mainly used for primary-intermediate metabolic pathways and is usually coupled to a TOF mass analyzer (Ramautar et al., 2016). In recent years, MS imaging (MSI) has evolved and has been used in different metabolic studies to obtain the distribution of compounds in tissue areas (cells, tissue, or specific sections) (Mhlongo et al., 2018). Compared to other traditional molecular imaging techniques, MSI makes it possible to obtain more information by providing the distribution of characteristics of a wide range of metabolites at high resolution (Schwamborn, 2012).
Metabolomics, the same as other omics tools, produces a large amount of complex data which require storage and processing instruments. These data can be processed using free statistical tools such as MarVis1, Mzine, XCMS, MAVEN, Metaboanalyst and MetAlign (Benton et al., 2008), or commercial programs: Markerlynx (Waters), profile creation solutions (Shimadzu), Mass Profiler.pro (Agilent) and metabolic profiler (Bruker) (Mhlongo et al., 2018). Multivariate statistical tools are also used to reduce data dimensionality, variable discrimination, and to form groups based on shared characteristics among samples (i.e., n-strains); part of these are the Principal Component Analysis and Orthogonal Projections to Latent Structures Discriminant Analysis (OPLS-DA) (Worley and Powers, 2013). Finally, the metabolites are annotated and identified using complementary databases which contain mass ranges, compound name and structures, statistical models, and metabolic pathways (Fukushima and Kusano, 2013). Recently, several databases have been developed that contain statistical and metabolomic tools based on MS or nuclear magnetic resonance, i.e., MeRy-B, MeltDB, and SetupX (Fukushima and Kusano, 2013).
The addition of metabolomic approaches to understanding the mechanisms of action of the BCA-M are highly valuable for the agricultural sector due to the wide diversity of metabolites produced by the BCA-M with high agro-biotechnological potential, and the knowledge of the metabolites involved in phytopathogens biological control during the BCA-host-phytopathogen-environment interaction. For example, Vinale et. al. (2014), using metabolomic approaches, identified a new metabolite produced by Trichoderma harzianum, called isoharzianic acid, which inhibits the growth of phytopathogens such as Sclerotinia sclerotiorum and Rhizoctonia solani. The isoharzianic acid also improves tomato seed germination and induces systemic resistance in plants. Similarly, other metabolites associated with biological control were reported for Trichoderma, such as hetelidic acid, sorbicilinol, trichodermanone C, giocladic acid and bisorbicilinol (Kang et al., 2011).
It is essential that in Mexico important agricultural producer support be strengthened for cutting-edge scientific research focused on the polyphasic taxonomic affiliation of BCA-M and the integrative study of the knowledge of the mechanisms of action of those agents during the interaction with the host plant, the phytopathogen, and the environment. This will strengthen the development, innovation, and success of the use of formulated and registered bioproducts and thus provide a sustainable and ecological alternative to benefit crop health.
Agroecological perspectives of BCA-M supported by omics sciences. The use of BCA-M is a functional and economically viable alternative for controlling phytopathogens. However, it is important to create and strengthen regional stocks to identify potential BCA-M and delve into the study of their mechanisms of action under field conditions, since this will make it possible to know with a greater degree of certainty what the diverse aspects that impact their establishment and functionality are, such as specific suppression actions, as well as the action threshold density, population dynamics, interspecific competence and mechanisms of physicochemical adaptation to the edaphic environment and plant. This knowledge would help establish doses, frequency, and multiplication-application substrates.
Among the aspects identified that are determinant to increase the efficiency of the BCA-M actions in the field, is the activation of the induced systemic response (ISR) through the recognition of microorganisms-associated molecular patterns (MAMPs) to pathogens (PAMPs), or damage (DAMPs) (Figure 2). This kind of signal in plants makes it possible to detect the pathogen and reduce its colonization in the infection spot, and even activate the systemic mechanisms in the plant’s distal tissues (Burketova et al., 2015). Thus, ISR is a plant status which, through biotic factors (i.e., BCA-M) or chemicals, protects itself against continuous and future phytopathogen attacks (Ku´c, 1982). This process was first reported when the phytopathogen came into contact with the root, but studies about the colonization of resistance inductors through foliar applications have proposed the initial mechanisms of that resistance. For example, Lamdan et al. (2015) reported that the BCA belonging to the Gliocladium virens and G. atroviride species interact with the plant and the pathogen, thereby promoting the plant’s growth and inducing a systemic response through proteins that are secreted by these fungi, which reduces the disease in the aerial parts of the plant. In this way, microbial elicitors induce the systemic response and act as endogenous or exogenous activators by reprogramming the resistance genes (Gupta and Bar, 2020). Ergosterol, the main component of fungal cell membranes and action substrate of many chemical fungicides, has acted as a resistance inductor in tomato and tobacco (Granado et al., 1995; Vatsa et al., 2011). On the other hand, host and non-host plants of Cladosporium fulvum (tomato pathogen) showed that the CfHNNI1 gene induces a hypersensitive response in tomato and tobacco (Xu et al., 2012), which coincides with the report of Zhang et al. (2013), who mentioned that the Sclerotinia sclerotiorum protein elicitor SCFE1 induced immunity through PAMP in Arabidopsis thaliana. Nevertheless, compared to bacteria and oomycetes elicitors, the fungal elicitors are not yet totally understood, and the function of those detected so far is still unknown, so the need to conduct further studies prevails (Liu et al., 2013).
In this way, healthy plants can be treated with systemic response inductors, but since they are not universal, more research processes are needed. To date, the induction of preventive response in plants is achieved with BCA-M to sensitize the host to respond quickly, strong, lasting, and with the least waste of the energy required when a phytopathogen attack occurs (Mauch-Mani et al., 2017). This is because when plants are growing under field conditions, they are continually exposed to different stimuli of abiotic and biotic type (bacteria, fungi, herbivore, oomycetes, viruses, arthropods, among others), which could activate defenses in the plants and influence the improvement of their response capacity by maintaining basal levels of resistance or a biochemical memory (Mauch-Mani et al., 2017). So, when a plant receives the stimulus, changes occur at the physiological, transcriptional, metabolic, and epigenetic levels, an event which is known as a preventive response induction phase for the plant to develop a faster and stronger response to a potential attack, since the plant was already prepared. This kind of preventive resistance can last and be kept during the plant’s life cycle, and the induction of preventive response can be considered transgenerational (Mauch-Mani et al., 2017). However, to understand the molecular mechanisms that induce a preventive response, two potential mechanisms are suggested: i) epigenetic changes in DNA methylation and modification of histones which may carry stress memories and trigger immune responses, and ii) accumulation of kinase proteins activated by mitogens (MPK) (Espinas et al., 2016).
On the other hand, another approach in the study of BCA-M is the use of secondary metabolites (SM), which is considered as a green solution in agriculture since most of those BCA can secrete their metabolites (Köhl et al., 2019). In this way, the bioactive metabolites (polyketides, non-ribosomal peptides, hybrid metabolites of peptide-peptide, terpenes, siderophores, lytic enzymes, among others) are isolated and purified (Figures 1 and 2). These metabolites are of great interest not only for the scientific community but also for the agro-biotechnology industry because of their biological activities against phytopathogens, such as hormones or carriers of chemical elements during biopesticide and biofertilizer production (Woo et al., 2014). SM are highly diverse in chemical structure, but the biosynthesis pathways are related to the primary metabolism, such as the pathway of shikimato acid, acetyl Co-A, sugar derivatives or amino acids (Pott et al., 2019). Understanding these metabolic pathways is very important for the innovation of products focused on phytopathogens control, either using traditional techniques or those labeled as omics.
A classic holistic approach that has been recently re-valuated with the advent of new technologies as metagenomics is the function of suppressive soils, whose microbial community composition keeps the phytopathogens present from infecting or multiplying in the plant of interest. Those soils can be divided into two categories: i) general suppressive soils, with a high content of microbial mass but low suppression levels; and ii) specific suppressive soils, with a high concentration of one or more microbial species and high levels of plant protection against pathogens (Mousa and Raizada, 2016). Shen et al. (2015) reported that the composition of the microbial community of the rhizosphere of chill pepper plants inoculated with B. amyloliquefaciens (a beneficial bacterium) induced Fusarium suppression and increased the potentially stimulating bacterial diversity (acidobacteria, firmicutes, Leptosphaeria and Phaeosphaeriopsis). However, there are no recent studies about suppressive soils, especially where BCA-M are continually applied for phytopathogen management, so this kind of studies is a challenge for the agro-biotechnological sector focused on ensuring plant health in a sustainable and integrating way by using highly accurate approaches as the omics sciences.
The development and innovation of the agro-biotechnology sector is decisive for the extensive and successful implementation of the BCA-M in Mexico, yet it represents a great scientific challenge. There are multiple studies about BCA-M used in phytopathogens management, but further integrative and creative studies are still needed to develop or innovate profitable, effective, and ecological alternatives to strengthen the use of high-quality and safe biopesticide bioproducts required for plant health in the world.
CONCLUSIONS
The massive use of BCA-M in Mexico and around the world requires a greater diversity of registered and marketed biopesticides. However, it is necessary to abandon the reductionist concept of implementation by using specimens from microbial culture collections, and the unrestricted regional scalability from the agroecological environment. It is necessary to create and have unlimited access to technical and scientific information that supports the relative effectiveness to the complex interactions with biotic and/or abiotic factors in the agroecosystem. This will permit the establishment of more effective development, innovation, and adoption processes of these agro-biotechnological strategies. In Mexico and other countries, the systemic and holistic research of BCA-M must be strengthened with the integration of modern approaches based on the emergence and development of the omics sciences, which can only be enhanced with highly skilled human capital and specialized scientific infrastructure. This will enable the development of more effective and cost-effective BCA-M through the bioprospection of new BCA-M and a detailed study of their taxonomic affiliation and mechanisms of action at the agroecosystem level. This can be achieved based on transdisciplinary thematic areas, such as microbiomes, metapopulation dynamics, functional relations at the community level, and gene-metabolic interaction in the plant-microorganisms-environment system under an adaptative and evolutionary environment. The systemic and comprehensive understanding of BCA-M in the agroecosystems, the establishment of researcher networks and biotechnological companies, as well as knowledge dissemination, will enhance the adoption of BCA-M in the Mexican cropping fields, and contribute to plant health and food security in a sustainable, economically viable and biosafety way.
Acknowledgments
The authors wish to thank Instituto Tecnológico de Sonora (ITSON) for funding the project PROFAPI 2020_0013 “Bacillus sp. TSO9: Afiliación taxonómica a nivel del genoma e identificación de genes asociados a la promoción del crecimiento en el trigo”. They also thank the valuable suggestions from Dr. Gustavo Mora Aguilera and the panel of anonymous reviewers, which enriched the content of this manuscript.
REFERENCES
Agrawal P, Khate S, Gupta M, Sain N and Mohanty D. 2017. RiPPMiner: a bioinformatics resource for deciphering chemical structures of RiPPs based on prediction of cleavage and cross-links. Nucleic Acids Research 45(W1): W80-W88. https://doi.org/10.1093/nar/gkx408 [ Links ]
Aguilar-Marcelino L, Mendoza-de-Gives P, Al-Ani LKT, López-Arellano ME, Gómez-Rodríguez O, Villar-Luna E and Reyes-Guerrero DE. 2020. Using molecular techniques applied to beneficial microorganisms as biotechnological tools for controlling agricultural plant pathogens and pest. Pp: 333-349. In: Sharma V, Salwan R, and Al-Ani LKT (eds). Molecular Aspects of Plant Beneficial Microbes in Agriculture. https://doi.org/10.1016/b978-0-12-818469-1.00027-4 425p. [ Links ]
Anders S, Pyl PT and Huber W. 2015. HTSeq--a Python framework to work with highthroughput sequencing data. Bioinformatics 31(2):166-169. https://doi.org/10.1093/bioinformatics/btu638 [ Links ]
Andrews S. 2010. FastQC: a quality control tool for high throughput sequence data. http://www.bioinformatics.babraham The RAST Server: rapid annotations using subsystems technology.ac.uk/projects/fastq http://www.bioinformatics.babraham.ac.uk/projects/fastqc (Consulta, Mayo, 2020). [ Links ]
Ansari MZ, Yadav G, Gokhale RS and Mohanty D. 2004. NRPS-PKS: a knowledge-based resource for analysis of NRPS/PKS megasynthases. Nucleic acids research 32(2): W405-W413. https://doi.org/10.1093/nar/gkh359 [ Links ]
Antipov D, Korobeynikov A, McLean JS and Pevzner PA. 2016. HYBRIDSPADES: an algorithm for hybrid assembly of short and long reads. Bioinformatics 32(7):1009-1015, https://doi.org/10.1093/bioinformatics/btv688 [ Links ]
Arredondo-Bernal HC y Rodríguez-del Bosque LA. 2015. Casos de control biológico en México. Editorial Colegio de Postgraduados. Vol. 2. Texcoco, México. 413 p. [ Links ]
Ayala-Zermeño MA, Gallou A, Berlanga-Padilla AM, Serna-Domínguez MG, Arredondo-Bernal HC and Montesinos-Matías R. 2015. Characterization of entomopathogenic fungi used in the biological control programme of Diaphorina citri in Mexico. Biocontrol Science and Technology 25(10): 1192-1207. https://doi.org/10.1080/09583157.2015.1041878 [ Links ]
Aziz RK, Bartels D, Best AA, DeJongh M, Disz T, Edwards RA, Formsma K, Gerdes S, Glass EM, Kubal M, Meyer F, Olsen GJ, Olson R, Osterman AL, Overbeek RA, McNeil LK, Paarmann D, Paczian T, Parrello B, Pusch GD, Reich C, Stevens R, Vassieva O, Vonstein V, Wilke A and Zagnitko O. 2008. The RAST Server: Rapid annotations using subsystems technology. BMC Genomics 9(75): 1-15. https://doi.org/10.1186/1471-2164-9-75 [ Links ]
Bankevich A, Nurk S, Antipov D, Gurevich AA, Dvorkin M, Kulikov AS, Lesin VM, Nikolenko SI, Pham S, Prjibelski AD, Pyshkin AV, Sirotkin AV, Vyahhi N, Tesler G, Alekseyev MA and Pevzner PA. 2012. SPAdes: a new genome assembly algorithm and its applications to single-cell sequencing. Journal of computational biology: a journal of computational molecular cell biology 19(5): 455-477. https://doi.org/10.1089/cmb.2012.0021 [ Links ]
Benton HP, Wong DM, Trauger SA and Siuzdak G. 2008. XCMS2: processing tandem mass spectrometry data for metabolite identification and structural characterization. Analitical Chemistry 80(16): 6382-6389. https://doi.org/10.1021/ac800795f [ Links ]
Bernal JS and Quezada JR. 1999. Perspectivas y desafíos para el control biológico en México. Vedalia 6: 3-14. [ Links ]
Blin K, Shaw S, Steinke K, Villebro R, Ziemert N, Lee SY, Medema MH and Weber T. 2019. AntiSMASH 5.0: updates to the secondary metabolite genome mining pipeline. Nucleic Acids Research 47(1): W81-W87. https://doi.org/10.1093/nar/gkz310 [ Links ]
Bolger AM, Lohse M and Usadel B. 2014. Trimmomatic: A flexible trimmer for Illumina Sequence Data. Bioinformatics 30(15): 2114-2120. https://doi.org/10.1093/bioinformatics/btu170 [ Links ]
Borodovsky M and Lomsadze A. 2011. Eukaryotic gene prediction using GeneMark.hmm-E and GeneMark-ES. Current protocols in bioinformatics 35(1): 4.6.1-4.6.10. https://doi.org/10.1002/0471250953.bi0406s35 [ Links ]
Bray NL, Pimentel H, Melsted P and Pachter L. 2016. Near-optimal probabilistic RNA-seq quantification. Nature Biotechnology 34: 525-527. https://doi.org/10.1038/nbt.3519 [ Links ]
Burketova L, Trda L, Ott PG and Valentova O. 2015. Bio-based resistance inducers for sustainable plant protection against pathogens. Biotechnology Advances 33(6):994-1004. https://doi.org/10.1016/j.biotechadv.2015.01.004 [ Links ]
Camacho C, Coulouris G, Avagyan V, Ma N, Papadopoulos J, Bealer K and Madden TL. 2009. BLAST+: architecture and applications. BMC Bioinformatics 10: 421. https://doi.org/10.1186/1471-2105-10-421 [ Links ]
Carrión G y Desgarennes D. 2012. Efecto de Paecilomyces lilacinus en nemátodos de vida libre asociados a la rizósfera de papas cultivadas en la región del Cofre de Perote, Veracruz, México. Revista Mexicana de Fitopatología 30(1): 86-90. http://www.scielo.org.mx/pdf/rmfi/v30n1/v30n1a9.pdf [ Links ]
Chaudhuri RR, Loman NJ, Snyder LAS, Bailey CM, Stekel DJ and Pallen MJ. 2008. xBASE2: a comprehensive resource for comparative bacterial genomics. Nucleic Acids Research 36(1): D543-D546. https://doi.org/10.1093/nar/gkm928 [ Links ]
Darling AC, Mau B, Blattner FR and Perna NT. 2004. Mauve: multiple alignment of conserved genomic sequence with rearrangements. Genome research 14:1394-1403. https://doi.org/10.1101/gr.2289704 [ Links ]
De León SG y Mier T. 2010. Visión general de la producción y aplicación de bioplaguicidas en México. Sociedades Rurales, Producción y Medio Ambiente 10(20): 37-63. https://biblat.unam.mx/hevila/Sociedadesruralesproduccionymedioambiente/2010/vol10/no20/2.pdf [ Links ]
De los Santos-Villalobos S, Kremer J M, Parra-Cota FI, Hayano-Kanashiro AC, García-Ortega LF, Gunturu SK, Tiedje JM, He SY, Peña-Cabriales JJ. 2018. Draft genome of the fungicidal biological control agent Burkholderia anthina strain XXVI. Archives of Microbiology 200:803-810. https://doi.org/10.1007/s00203-018-1490-6 [ Links ]
De los Santos-Villalobos S, Parra-Cota FI , Herrera-Sepúlveda A, Valenzuela-Aragón B y Estrada-Mora JC. 2018. Colmena: colección de microorganismos edáficos y endófitos nativos, para contribuir a la seguridad alimentaria nacional. Revista Mexicana de Ciencias Agrícolas 9(1): 191-202.https://doi.org/10.29312/remexca.v9i1.858 [ Links ]
De los Santos-Villalobos S, Robles-Montoya RI, Parra-Cota FI , Larsen J, Lozano P andTiedje JM . 2019. Bacillus cabrialesii sp. nov., an endophytic plant growth promoting bacterium isolated from wheat (Triticum turgidum subsp. durum) in the Yaqui Valley, Mexico. International Journal of Systematic and Evolutionary Microbiology 69(12): 3939-3945. https://doi.org/10.1099/ijsem.0.003711 [ Links ]
Delcher AL, Bratke KA, Powers EC and Salzberg SL. 2007. Identifying bacterial genes and endosymbiont DNA with Glimmer. Bioinformatics 23(6): 673-679. https://doi.org/10.1093/bioinformatics/btm009 [ Links ]
Delgado Ortiz JC, Beltrán Beache M, Cerna Chávez E, Aguirre Uribe LA, Landero Flores J, Rodríguez Pagaza Y y Ochoa Fuentes YM. 2019. Candidatus Liberibacter solanacearum patógeno vascular de solanáceas: Diagnóstico y control. TIP Revista Especializada en Ciencias Químico-Biológicas 22: 1-12. https://doi.org/10.22201/fesz.23958723e.2019.0.177 [ Links ]
Dobin A, Davis CA, Schlesinger F, Drenkow J, Zaleski C, Jha S and Gingeras TR 2013. STAR: Ultrafast universal RNA-seq aligner. Bioinformatics 29(1): 15-21. https://doi.org/10.1093/bioinformatics/bts635 [ Links ]
Duke KA, Becker MG, Girard LJ, Millar JL, Fernando WGD, Belmonte MF and De Kievit TR. 2017. The biocontrol agent Pseudomonas chlororaphis PA23 primes Brassica napus defenses through distinct gene networks. BMC Genomics 18: 1-16. https://doi.org/10.1186/s12864-017-3848-6 [ Links ]
Ehling-Schulzn M, Lereclus D and Koehler TM. 2019. TheBacillus cereusGroup:Bacillusspecies with pathogenic potential. Microbiology Spectrum 7(3): GPP3-0032. https://doi.org/10.1128/microbiolspec.GPP3-0032-2018 [ Links ]
Espinas NA, Saze H and Saijo Y. 2016. Epigenetic Control of Defense Signaling and Priming in Plants. Frontiers in Plant Science 7: 1201-1201. https://doi.org/10.3389/fpls.2016.01201 [ Links ]
Espinosa-Victoria D, López-Reyes L, Carcaño-Montiel MG and Serret-López M. 2020. The Burkholderia genus: between mutualism and pathogenicity. Mexican Journal of Phytopathology 38(3): 337-359. https://doi.org/10.18781/R.MEX.FIT.2004-5 [ Links ]
FAO. 2018. El futuro de la alimentación y la agricultura: Vías alternativas hacia el 2050. Versión resumida. Rome. 64 pp. http://www.fao.org/3/CA1553ES/ca1553es.pdf (Consulta, Mayo 2020). [ Links ]
Franco-Navarro F, Cid del Prado-Vera I y Romero-Tejeda, ML. 2013. Aislamiento y Potencial Parasítico de un Aislamiento Nativo de Pochonia chlamydosporia en Contra de Nacobbus aberrans en Frijol. Revista Mexicana de Fitopatología 30(2): 101-114. http://www.scielo.org.mx/pdf/rmfi/v30n2/v30n2a1.pdf [ Links ]
Fukushima A and Kusano M. 2013. Recent progress in the development of metabolome databases for plant systems biology. Frontier in Plant Science 4: 73. http://dx.doi.org/10.3389/fpls.2013.00073 [ Links ]
Galindo E, Serrano-Carreón L, Gutiérrez CR, Allende R, Balderas K, Patiño M, Trejo M, Wong MA, Rayo E, Isauro D and Jurado C. 2013. The challenges of introducing a new biofungicide to the market: A case study. Electronic Journal of Biotechnology 16(3): 5-5. http://dx.doi.org/10.2225/vol16-issue3-fulltext-6 [ Links ]
Galindo E, Serrano-Carreón L, Gutiérrez CR, Balderas-Ruíz KB, Muñoz-Celaya AL, Mezo-Villalobos M y Arroyo-Colín J. 2015. Desarrollo histórico y los retos tecnológicos y legales para comercializar Fungifree AB®, el primer biofungicida 100% mexicano. Revista Especializada en Ciencias Químico-Biológicas 18(1): 52-60. https://doi.org/10.1016/j.recqb.2015.05.005 [ Links ]
Gallou A, Serna-Domínguez MG, Berlanga-Padilla AM, Ayala-Zermeño MA, Mellín-Rosas MA, Montesinos-Matías R and Arredondo-Bernal HC. 2016. Species clarification of Isaria isolates used as biocontrol agents against Diaphorina citri (Hemiptera: Liviidae) in Mexico. Fungal biology 120(3): 414-423. https://doi.org/10.1016/j.funbio.2015.11.009 [ Links ]
García-Juárez HS, Ortiz-García CF, Salgado-García S, Valdez-Balero A, Silva-Rojas HV, and Ovalle-Saenz WR. 2016. Immunodetection of the Leifsopnia Xyli ssp. xyli bacteria in commercial clones of Saccharum spp. in Tabasco, Mexico. Agro Productividad 9(3): 3-9. https://www.colpos.mx/wb_pdf/Agroproductividad/2016/AGROPRODUCTIVIDAD_III_2016.pdf [ Links ]
García-Nevárez G and Hidalgo-Jaminson E. 2019. Efficacy of indigenous and commercial Simplicillium and Lecanicillium strains for controlling Hemileia vastatrix. Mexican Journal of Phytopathology 37(2): 237-250. https://doi.org/10.18781/R.MEX.FIT.1810-4 [ Links ]
García-Núñez HG, Martínez-Campos AR, Hermosa-Prieto MR; Monte-Vázquez E, Aguilar-Ortigoza CJ and González-Esquivel CE. 2017. Morphological and molecular characterization of native isolates of Trichoderma and its potential biocontrol against Phytophthora infestans. Revista Mexicana de Fitopatología 35(1): 58-79. https://doi.org/10.18781/R.MEX.FIT.1605-4 [ Links ]
Gill HK and Garg H. 2014. Pesticides: environmental impacts and management strategies. In: Larramendy ML and Soloneski S (eds.). Pesticides-toxic aspects. IntechOpen. http://dx.doi.org/10.5772/57399 [ Links ]
Gómez-De La Cruz I, Pérez-Portilla E, Escamilla-Prado E, Martínez-Bolaños M, Carrión-Villarnovo GLL and Hernández-Leal TI. 2017. Selection in vitro of mycoparasites with potential for biological control on Coffee Leaf Rust (Hemileia vastatrix). Revista Mexicana de Fitopatología 36(1): 172-183. https://doi.org/10.18781/R.MEX.FIT.1708-1 [ Links ]
Granado J, Felix G and Boller T. 1995. Perception of fungal sterols in plants-Subnanomolar concentrations of ergosterol elicits extracellular alkalinisation in tomato cells. Plant Physiology 107: 485-490. https://doi.org/10.1104/pp.107.2.485 [ Links ]
Guerrero PVM, Blanco PAC, Guigón LC, Tamayo UCJ, Molina CFJ, Berlanga RDI, Carvajal ME and Ávila QGD. 2011. Competencia por nutrientes; modo de acción de Candida oleophila contra Penicillium expansum y Botrytis cinerea. Revista Mexicana de Fitopatología 29 (2): 90-97. http://www.scielo.org.mx/pdf/rmfi/v29n2/v29n2a1.pdf [ Links ]
Gupta R and Bar M. 2020. Plant Immunity, Priming, and Systemic Resistance as Mechanisms for Trichoderma spp. Biocontrol. Pp: 81-110. In: Sharma A. and Sharma P. (eds) Trichoderma. Rhizosphere Biology. Springer, Singapore. https://doi.org/10.1007/978-981-15-3321-1_5 [ Links ]
Gurevich A, Saveliev V, Vyahhi N and Tesler G. 2013. QUAST: quality assessment tool for genome assemblies. Bioinformatics 29(8): 1072-1075. http://dx.doi.org/10.1093/bioinformatics/btt086 [ Links ]
Hassan MM, Farid MA and Gaber A. 2019. Rapid identification of Trichoderma koningiopsis and Trichoderma longibrachiatum using sequence-characterized amplified region markers. Egyptian Journal of Biological Pest Control 29 (13): 1-8. https://doi.org/10.1186/s41938-019-0113-0 [ Links ]
Illa C, Pérez AA, Torassa M and Pérez MA. 2020. Effect of biocontrol and promotion of peanut growth by inoculating Trichoderma harzianum and Bacillus subtilis under controlled conditions and field. Mexican Journal of Phytopathology 38(1): 119-131. https://doi.org/10.18781/R.MEX.FIT.1910-6 [ Links ]
Instituto Nacional de Estadística y Geografía (INEGI). 2017. Encuesta Nacional Agropecuaria 2017 MEX-INEGI.EEC2.05-ENA-2017. https://www.inegi.org.mx/rnm/index.php/catalog/498 (Consulta, Mayo, 2020). [ Links ]
Jagadeesan B, Gerner-Smidt P, Allard MW, Leuillet S, Winkler A, Xiao Y, Chaffron S, Vossen JVD, Tang S, Katase M, McClure P, Kimura B, Chai LC, Chapman J and Grant K. 2019. The Use of Next Generation Sequencing for Improving Food Safety: Translation into practice. Food Microbiology 79: 96-115. https://doi.org/10.1016/j.fm.2018.11.005 [ Links ]
Jhonson AD, Handsaker RE, Pulit SL, Nizzari MM, O’Donell CJ and andde Bakker PIW. 2008. SNAO: a web based tool for identification and annotation of proxy SNPs using hadmap. Bioinformatics 24(24): 2938-2939. https://doi.org/10.1093/bioinformatics/btn564 [ Links ]
Jiménez-Delgadillo R, Valdés-Rodríguez SE, Olalde-Portugal V, Abraham-Juárez R and García-Hernández JL. 2018. Effect of pH and temperature on the growth and antagonistic activity of Bacillus subtilis on Rhizoctonia solani. Revista Mexicana de Fitopatología 36(2): 256-275. https://doi.org/10.18781/R.MEX.FIT.1711-3 [ Links ]
Kanehisa M, Goto S, Kawashima S, Okuno Y and Hattori M. 2004. The KEGG resource for deciphering the genome. Nucleic Acids Research 32: 277-280. https://doi.org/10.1093/nar/gkh063 [ Links ]
Kang D, Kim J, Choi JN, Liu KH and Lee CH. 2011. Chemotaxonomy of Trichoderma spp. using mass spectrometry-based metabolite profiling. Journal of Microbiology and Biotechnology 21(1): 5-13. https://doi.org/10.4014/jmb.1008.08018 [ Links ]
Keller O, Kollmar M, Stanke M and Waack S. 2011. A novel hybrid gene prediction method employing protein multiple sequence alignments. Bioinformatics 27(6): 757-763. https://doi.org/10.1093/bioinformatics/btr010. [ Links ]
Kim D, Pertea G, Trapnell C, Pimentel H, Kelley R and Salzberg SL. 2013. TopHat2: accurate alignment of transcriptomes in the presence of insertions, deletions and gene fusions. Genome Biology 14(4):1-13. https://genomebiology.biomedcentral.com/articles/10.1186/gb-2013-14-4-r36 [ Links ]
Köhl J, Kolnaar R and Ravensberg WJ. 2019. Mode of action of microbial biological control agents against plant diseases: relevance beyond efficacy. Frontier in Plant Science 10: 845. https://doi.org/10.3389/fpls.2019.00845 [ Links ]
Koren S, Walenz BP, Berlin K, Miller JR, Bergman NH and Phillipy AM. 2017. Canu: scalable and accurate long-read assembly via adaptive k-mer weighting and repeat separation. Genome Research 27: 722-736. https://doi.org/10.1101/gr.215087.116 [ Links ]
Ku’c J. 1982. Induced immunity to plant disease. BioScience 32(11): 854-860. https://doi.org/10.2307/1309008 [ Links ]
Lagunes-Castro MS, López Monteon A, Ramos Ligonio A, Trigos A, Salinas A y Espinoza C. 2015. Actividad antibacteriana de extractos metanol:cloroformo de hongos fitopatógenos. Revista Mexicana de Fitopatología 33(1): 87-94. http://www.scielo.org.mx/pdf/rmfi/v33n1/2007-8080-rmfi-33-01-00087.pdf [ Links ]
Lamdan NL, Shalaby S, Ziv T, Kenerley CM and Horwitz BA. 2015. Secretome of Trichoderma interacting with maize roots: role in induced systemic resistance. Molecular Cell Proteomics 14(4): 1054-1063. https://doi.org/10.1074/mcp.M114.046607 [ Links ]
Langmead B and Salzberg SL. 2012. Fast gapped-read alignment with Bowtie 2. Nature Methods 9: 357-359.https://www.nature.com/articles/nmeth.1923 [ Links ]
Ley-López N, Márquez-Zequera I, Carrillo-Fasio JA, León-Félix J, Cruz-Lachica I, García-Estrada RS and Allende-Molar R. 2018. Effect of biocontrol and germinative inhibition of Bacillus spp. on zoospores of Phytophthora capsici. Revista Mexicana de Fitopatología 36(2): 215-232. https://doi.org/10.18781/r.mex.fit.1711-2 [ Links ]
Li B and Dewey CN. 2011. RSEM: accurate transcript quantification from RNA-Seq data with or without a reference genome. BMCBioinformatics 12: 323. https://doi.org/10.1186/1471-2105-12-323 [ Links ]
Li H and Durbin R. 2009. Fast and accurate short read alignment with Burrows-Wheeler transform. Bioinformatics 25(14): 1754-1760. https://doi.org/10.1093/bioinformatics/btp324 [ Links ]
Li H. 2011. ImprovingSNP discovery by base alignment quality. Bioinformatics 27(8): 1157-1158. https://doi.org/10.1093/bioinformatics/btr076 [ Links ]
Li R, Yu C, Li Y, Lam TW, Yiu SM, Kristiansen K and Wang J. 2009. SOAP2: an improved ultrafast tool for short read alignment. Bioinformatics 25(15): 1966-1967. https://doi.org/10.1093/bioinformatics/btp336 [ Links ]
Liu W, Liu J, Ning Y, Ding B, Wang X, Wang Z and Wan GL. 2013. Recent progress in understanding PAMP- and effector-triggered immunity against the rice blast fungus Magnaporthe oryzae. Molecular Plant 6(3): 605-620. https://doi.org/10.1093/mp/sst015 [ Links ]
Liu Y, Lai Q, Göker M, Meier-Kolthoff JP, Wang M, Sun Y, Wang L and Shao Z. 2015. Genomic insights into the taxonomic status of the Bacillus cereus group. Scientific Reports 5: 14082. https://doi.org/10.1038/srep14082 [ Links ]
Lloyd N, Johnson DL and Herderich MJ. 2015. Metabolomics approaches for resolving and harnessing chemical diversity in grapes, yeast and wine. Australian Journal of Grape Wine Research 21(S1): 723-740. https://doi.org/10.1111/ajgw.12202 [ Links ]
Love MI, Huber W and Anders S. 2014. Moderated estimation of fold change and dispersion for RNA-seq data with DESeq2. Genome Biology 15: 550. http://dx.doi.org/10.1186/s13059-014-0550-8 [ Links ]
Lozano-Alejo N, Guzmán-Plazola RA, Zavaleta-Mejía E, Aguilar-Rincón VH y Ayala-Escobar V. 2015. Etiología y evaluación de alternativas de control de la Marchitez del chile de árbol (Capsicum annuum L.) en La Vega de Metzitlán, Hidalgo, México. Revista Mexicana de Fitopatología 33(1): 31-53. http://www.scielo.org.mx/pdf/rmfi/v33n1/2007-8080-rmfi-33-01-00031.pdf [ Links ]
Luo R, Liu B, XieY, Li Z, Huang W, Yuan J, He G, Chen Y, Pan Q, Liu Y, Tang J, Wu G, Zhang H, Shi Y, Liu Y, Yu C, Wang B, Lu Y, Han C, Cheung DW, Yiu SM, Peng S, Xiaoqian Z, Liu G, Liao X, Li Y, Yang H, Wang J, Lam TW and Wang J. 2012. SOAPdenovo2: an empirically improved memory-efficient short read de novo assembler. Gigascience 1(1): 18. https://doi.org/10.1186/2047-217X-1-18 [ Links ]
Macedo CA, Martínez HA y Lara RJ. 2012. Rizobacterias aisladas del trópico húmedo con actividad antagónica sobre Colletotrichum gloeosporioides, evaluación cuantitativa e identificación molecular. Revista Mexicana de Fitopatología 30(1): 11-30. http://www.scielo.org.mx/pdf/rmfi/v30n1/v30n1a2.pdf [ Links ]
Martin M. 2011. Cutadapt removes adapter sequences from high-throughput sequencing reads. EMBnet.journal 17(1): 10-12. https://doi.org/10.14806/ej.17.1.200 [ Links ]
Mauch-Mani B, Baccelli I, Luna E and Flors V. 2017. Defense Priming: An Adaptive Part of Induced Resistance. Annual Review of Plant Biology 68: 485-512. https://doi.org/10.1146/annurev-arplant-042916-041132 [ Links ]
Mazrou YSA, Makhlouf AH, Elseehy MM, Awad MF and Hassan MM. 2020. Antagonistic activity and molecular characterization of biological control agent Trichoderma harzianum from Saudi Arabia. Egyptian Journal of Biological Pest Control 30(4): 1-8. https://doi.org/10.1186/s41938-020-0207-8 [ Links ]
Meier-Kolthoff JP, Auch AF, Klenk HP and Göker M. 2013. Genome sequence-based species delimitation with confidence intervals and improved distance functions. BMC Bioinformatics 14(60): 1-14. https://doi.org/10.1186/1471-2105-14-60 [ Links ]
Mejía-Bautista MA, Reyes-Ramírez A, Cristobal-Alejo J, Tun-Suárez JM, Borges-Gómez LC y Pacheco-Aguilar JR. 2016. Bacillus spp. in the control of wilt caused by Fusarium spp. in Capsicum chinense. Revista Mexicana de Fitopatología 34(3): 208-222. https://doi.org/10.18781/R.MEX.FIT.1603-1 [ Links ]
Mhlongo MI, Piater LA, Madala NE, Labuschagne N and Dubery IA. 2018. The Chemistry of Plant-Microbe interactions in the rhizosphere and the potential for metabolomics to reveal signaling related to defense priming and Induced Systemic Resistance. Frontiers in Plant Science 9: 112. https://doi.org/10.3389/fpls.2018.00112 [ Links ]
Mi H, Muruganujan A, Casagrande JT and Thomas PD. 2013. Large-scale gene function analysis with the PANTHER classification system. Nature Protocols 8: 1551-1566. https://doi.org/10.1038/nprot.2013.092 [ Links ]
Michel-Aceves AC, Otero-Sánchez MA, Díaz-Castro A, Martínez-Rojero RD, Ariza-Flores R y Barrios-Ayala A. 2013. Biocontrol de la “Escoba de Bruja” del mango, con Trichoderma spp., en condiciones de campo. Revista Mexicana de Fitopatología 31(1): 1-12. http://www.scielo.org.mx/pdf/rmfi/v31n1/v31n1a1.pdf [ Links ]
Mitchell A, Chang HY, Daugherty L, Fraser M, Hunter S, López R, McAnulla C, McMenamin C, Nuka G, Pesseat S, Sangrador-Vegas A, Scheremetjew M, Rato C, Yong SY, Bateman A, Punta M, Attwood TK, Sigrist CJA, Redaschi N, Rivoire C, Xenarios I, Kahn D, Guyot D, Bork P, Letunic I, Gough J, Oates M, Haft D, Huang H, Natale DA, Wu CH, Orengo C, Sillitoe I, Mi H, Thomas PD and Finn R. 2015. The InterPro protein families datábase: the classification resource after 15 years. Nucleic Acids Research 43(D1): 1-9. https://doi.org/10.1093/nar/gku1243 [ Links ]
Moriuchi R, Dohra H, Kanesaki Y and Ogawa N. 2019. Complete Genome Sequence of Bacterium Cupriavidus necator NH9 and Reclassification of the Strains of the Genera Cupriavidus and Ralstonia Based on Phylogenetic and Whole-Genome Sequence Analyses. Frontiers in Microbiology 10: 1-21. https://doi.org/10.3389/fmicb.2019.00133 [ Links ]
Mousa WK and Raizada MN. 2016. Natural disease control in cereal grains. Pp:1-7. In: reference module in Food Science. https://doi.org/10.1016/B978-0-08-100596-5.00206-7 [ Links ]
Naz S. 2014. Analytical protocols based on LC-MS, GC-MS and CE-MS for nontargeted metabolomics of biological tissues. Bioanalysis 6(12): 1657-1677. https://doi.org/10.4155/BIO.14.119 [ Links ]
Ocegueda-Reyes MD, Casas-Solís J, Virgen-Calleros G, González-Eguiarte DR, López-Alcocer E and Olalde-Portugal V. 2020. Isolation, identification and characterization of antagonistic rhizobacteria to Sclerotium cepivorum. Mexican Journal of Phytopathology 38(1): 146-159. https://doi.org/10.18781/R.MEX.FIT.1911-2 [ Links ]
ONU. 2018. La Agenda 2030 y los Objetivos de Desarrollo Sostenible: una oportunidad para América Latina y el Caribe (LC/G.2681-P/Rev.3), Santiago. https://repositorio.cepal.org/bitstream/handle/11362/40155/24/S1801141_es.pdf (Consulta, Junio 2020). [ Links ]
Parks DH, Imelfort M, Skennerton CT, Hugenholtz P and Tyson GW. 2015 CheckM: assessing the quality of microbial genomes recovered from isolates, single cells, and metagenomes. Genome Research 25: 1043 1055. https://doi.org/10.1101/gr.186072.114 [ Links ]
Peng Y, Leung HCM, Yiu SM, Chin FYL and IDBA-UD. 2012. a de novo assembler for single-cell and metagenomic sequencing data with highly uneven depth. Bioinformatics 28(11): 1420-1428. https://doi.org/10.1093/bioinformatics/bts174 [ Links ]
Pérez-Moreno L, Belmonte-Vargas JR, Núñez-Palenius HG, Guzmán-Mendoza R y Mendoza-Celedón B. 2015. Sensibilidad de especies de Sclerotinia spp. y Sclerotium cepivorum a agentes de control biológico y fungicidas. Revista Mexicana de Fitopatología 33(2): 256-267. http://www.scielo.org.mx/pdf/rmfi/v33n2/2007-8080-rmfi-33-02-00256.pdf [ Links ]
Pérez-Rojas F, León-Quispe J y Galindo-Cabello N. 2015. Actinomicetos Aislados del Compost y su Actividad Antagonista a Fitopatógenos de la papa (Solanum tuberosum spp. andigena Hawkes). Revista Mexicana de Fitopatología 33(2): 116-139. http://www.scielo.org.mx/pdf/rmfi/v33n2/2007-8080-rmfi-33-02-00116-en.pdf [ Links ]
Petersen TN, Brunak S, von Heijne G and Nielsen H. 2011. SignalP 4.0: Discriminating signal peptides from transmembrane regions. Natural Methods 8: 785-786. https://doi.org/10.1038/nmeth.1701 [ Links ]
Pott DM, Osorio S and Vallarino JG. 2019. From central to specialized metabolism: an overview of some secondary compounds derived from the primary metabolism for their role in conferring nutritional and organoleptic characteristics to fruit. Frontiers in Plant Science 10: 835. https://doi.org/10.3389/fpls.2019.00835 [ Links ]
Potter SC, Aurélien Luciani, Sean R Eddy, Youngmi Park, Rodrigo Lopez and Robert D Finn. 2018. HMMER web server: 2018 update. Nucleic Acids Research 46(W1): W200-W204. https://doi.org/10.1093/nar/gky448 [ Links ]
Quinland A and Hall IM. 2010. BEDTools: a flexible suite of utilities for comparing genomic features. Bioinformática 26(6): 841-2 https://doi.org/10.1093/bioinformatics/btq033 [ Links ]
Raja HA, Miller AN, Pearce CJ and Oberlies NH. 2017. Fungal Identification Using Molecular Tools: A Primer for the Natural Products Research Community. Journal of Natural Products 80(3): 756-770. https://doi.org/10.1021/acs.jnatprod.6b01085 [ Links ]
Ramautar R, Somsen GW and de Jong GJ. 2016. CE-MS for metabolomics: developments and applications in the period 2014-2016. Electrophoresis 38(1): 1-13. https://doi.org/10.1002/elps.201600370 [ Links ]
Richter M, Rosselló-Móra R, Oliver Glöckner F and Peplies J. 2016. JSpeciesWS: a web server for prokaryotic species circumscription based on pairwise genome comparison. Bioinformatics 32(6): 929-931. https://doi.org/10.1093/bioinformatics/btv681 [ Links ]
Rios-Velasco C, Caro-Cisneros JN, Berlanga-Reyes DI, Ruíz-Cisneros MF, Ornelas-Paz JJ, Salas-Marina MA, Villalobos-Pérez E and Guerrero-Prieto VM. 2016. Identification and antagonistic activity in vitro af Bacillus spp. and Trichoderma spp. isolates against common phytopathogenic fungi. Revista Mexicana de Fitopatología 34(1): 84-99. https://doi.org/10.18781/R.MEX.FIT.1507-1 [ Links ]
Rodríguez-Millán KA, Monreal Vargas CT, Huerta Díaz J, Soria Colunga JC y Jarquín Gálvez R. 2013. Aporte de microorganismos benéficos por la incorporación al suelo de residuos deshidratados de col (Brassica oleracea var capitata) y su efecto en el pH. Revista Mexicana de Fitopatología 31(1):29-44. http://www.scielo.org.mx/pdf/rmfi/v31n1/v31n1a4.pdf [ Links ]
Rodríguez-Romero VM, Villanueva-Arce R, Trejo-Raya AB and Bautista-Baños S. 2019. Chitosan and Pseudomonas fluorescens extracts for Alternaria alternata control in tomato (Solanum lycopersicum). Mexican Journal of Phytopathology 37(2): 202-219. https://doi.org/10.18781/R.MEX.FIT.1812-2 [ Links ]
Rollano-Peñaloza OM y Mollinedo-Portugal P. 2017. Análisis bioinformático de Arn-Seq con una perspectiva para Bolivia. Revista Boliviana de Química 4(2): 50-55. http://www.scielo.org.bo/pdf/rbq/v34n2/v34n2_a02.pdf [ Links ]
Roser M. 2019. Pesticides. University of Oxford. https://ourworldindata.org/pesticides (Consulta, Mayo de 2020). [ Links ]
Ruiz-Cisneros MF, Ornelas-Paz JJ, Olivas-Orozco GI, Acosta-Muñiz CH, Sepúlveda-Ahumada DR, Pérez-Corral DA, Rios-Velasco C, Salas-Marina MA and Fernández-Pavía SP. 2018. Efect of Trichoderma spp. and phytopathogenic fungi on plant growth and tomato fruit quality. Revista Mexicana de Fitopatología 36(3): 444-456. https://doi.org/10.18781/R.MEX.FIT.1804-5 [ Links ]
Ruiz-Cisneros MF, Rios-Velasco C, Berlanga-Reyes DI, Ornelas-Paz JJ, Acosta-Muñiz CH, Romo-Chacón A, Zamudio-Flores PB, Pérez-Corral DA, Salas-Marina MÁ, Ibarra-Rendón JE and Fernández-Pavía SP. 2017. Incidence and causal agents of root diseases and its antagonists in apple orchards of Chihuahua, México. Revista Mexicana de Fitopatología 35(3): 437-462. https://doi.org/10.18781/R.MEX.FIT.1704-3 [ Links ]
Samaniego-Gaxiola JA, Pedroza-Sandoval A, Chew-Madinaveitia Y and Gaytán-Mascorro A. 2019. Reductive disinfestation, soil desiccation and Trichoderma harzianum to control Phymatotrichopsis omnivora in pecan tree nursery. Mexican Journal of Phytopathology 37(2): 287-303. https://doi.org/10.18781/R.MEX.FIT.1808-7 [ Links ]
Serret-López M, Aranda-Ocampo S, Espinosa-Victoria D, Ortiz-Martínez LE and Ramírez-Razo K. 2021. Polyphasic characterization of Burkholderia gladioli isolated from onion and evaluation of its potential pathogenicity for other crops. Mexican Journal of Phytopathology 39(1): 1-20. https://doi.org/10.18781/R.MEX.FIT.2007-2 [ Links ]
Schmieder R and Edwards R. 2011. Quality control and preprocessing of metagenomic datasets. Bioinformatics 27(6): 863-864. https://doi.org/10.1093/bioinformatics/btr026 [ Links ]
Schwamborn K. 2012. Imaging mass spectrometry in biomarker discovery and validation.Journal of Proteomics 75(16): 4990-4998. https://doi.org/10.1016/j.jprot.2012.06.015 [ Links ]
Sentausa E and Fournier PE. 2013. Advantages and limitations of genomics in prokaryotic taxonomy. Clinical microbiology and infection. European Society of Clinical Microbiology and Infectious Diseases 19(9): 790-795. https://doi.org/10.1111/1469-0691.12181 [ Links ]
Serrano-Carreón L, Balderas K, Wong MA, Rosas DR y Galindo-Fentanes EG. 2010. Biofungicidas para el control de la antracnosis del mango: Logrando frutos de alta calidad internacional para mercados exigentes. Claridades Agropecuarias 208: 28-37. https://info.aserca.gob.mx/claridades/revistas/208/ca208-28.pdf [ Links ]
Shen Z, Ruan Y and Chao X. 2015. Rhizosphere microbial community manipulated by 2 years of consecutive biofertilizer application associated with banana Fusarium wilt disease suppression. Biology and Fertility Soils 51: 553-562. https://doi.org/10.1007/s00374-015-1002-7 [ Links ]
Skinnider MA, Merwin NJ, Johnston CW and Magarvey NA. 2017. PRISM 3: expanded prediction of natural product chemical structures from microbial genomes. Nucleic Acids Research 45(W1): W49-W54. https://doi.org/10.1093/nar/gkx320 [ Links ]
Tian T, Liu Y, Yan H, You Q, Yi X, Du Z, Xu W and Su Z. 2017. agriGO v2.0: a GO analysis toolkit for the agricultural community, 2017 update. Nucleic acids research 45(W1): W122-W129. https://doi.org/10.1093/nar/gkx382 [ Links ]
Tlapal-Bolaños B, González Hernández H, Zavaleta Mejía E, Sánchez García P, Mora Aguilera G, Nava Díaz C, Del Real Laborde JI y Rubio Cortes R. 2014. Colonización de Trichoderma y Bacillus en plántulas de Agave tequilana Weber, var. Azul y el efecto sobre la fisiología de la planta y densidad de Fusarium. Revista Mexicana de Fitopatología 32(1): 62-74. http://www.scielo.org.mx/pdf/rmfi/v32n1/v32n1a6.pdf [ Links ]
Trapnell C, Pachter L and Salzberg SL. 2009. TopHat: discovering splice junctions with RNA-Seq. Bioinformatics 25(9): 1105-1111. https://doi.org/10.1093/bioinformatics/btp120 [ Links ]
Trinidad-Cruz JR, Quiñones-Aguilar EE, Rincón-Enríquez G, López-Pérez L y Hernández-Cuevas LV. 2017. Mycorrhization of Agave cupreata: Biocontrol of Fusarium oxysporum and plant growth promotion. Revista Mexicana de Fitopatología 35(2): 151-169. https://doi.org/10.18781/R.MEX.FIT.1607-5 [ Links ]
Uc-Arguelles AK, Perez-Moreno J, Ayala-EscobaR V y Zavaleta-Mejia E. 2017. Antagonismo de Saccharicola sp. contra fitopatógenos de la raíz de chile jalapeño (Capsicum annuum). Revista Mexicana de Fitopatología 35(2):263-283. http://dx.doi.org/10.18781/R.MEX.FIT.1611-6 [ Links ]
Valenzuela-Aragon B, Parra-Cota FI , Santoyo G Arellano-Wattenbarger GL and De los Santos-Villalobos S . 2019. Plant-assisted selection: a promising alternative for in vivo identification of wheat (Triticum turgidum L. subsp. Durum) growth promoting bacteria. Plant Soil 435: 367-384. https://doi.org/10.1007/s11104-018-03901-1 [ Links ]
Valenzuela-Ruiz V, Gálvez-Gamboa GT, Villa-Rodríguez ED, Parra-Cota FI , Santoyo G andDe los Santos-Villalobos S . 2020. Lipopeptides produced by biological control agents of the genus Bacillus: a review of analytical tools used for their study. Revista Mexicana de Ciencias Agrícolas 11(2): 419-432. https://doi.org/10.29312/remexca.v11i2.2191 [ Links ]
Valenzuela-Ruiz V, Robles-Montoya R, Parra-Cota FI , Santoyo G, Orozco-Mosqueda, Ma. Del Carmen, Rodríguez-Ramírez R and De los Santos-Villalobos S . 2019. Draft genome sequence of Bacillus paralicheniformis TRQ65, a biological control agent and plant growth-promoting bacterium isolated from wheat (Triticum turgidum subsp. durum) rhizosphere in the Yaqui Valley, Mexico. 3 Biotech 9:436. https://doi.org/10.1007/s13205-019-1972-5 [ Links ]
Van Heel AJ, de Jong A, Song C, Viel JH, Kok J and Kuipers OP. 2018. BAGEL4: a user-friendly web server to thoroughly mine RiPPs and bacteriocins. Nucleic Acids Research 46(W1): W278-W281. https://doi.org/10.1093/nar/gky383 [ Links ]
Varghese NJ, Mukherjee S, Ivanova N, Konstantinidis KT, Mavrommatis K, Kyrpides NC and Pati A. 2015. Microbial species delineation using whole genome sequences. Nucleic Acids Research 43(14): 6761-6771. https://doi.org/10.1093/nar/gkv657 [ Links ]
Vatsa P, Chiltz A, Luini E, Vandelle E, Pugin A and Roblin G. 2011. Cytosolic calcium rises and related events in ergosterol-treated Nicotiana cells. Plant Physiology and Biochemestry 49(7): 764-773. https://doi.org/10.1016/j.plaphy.2011.04.002 [ Links ]
Villa-Rodríguez E, Parra-Cota F, Castro-Longoria E, López-Cervantes J and De los Santos-Villalobos S . 2019. Bacillus subtilis TE3: A promising biological control agent against Bipolaris sorokiniana, the causal agent of spot blotch in wheat (Triticum turgidum L. subsp. durum). Biological control 132: 135-143. https://doi.org/10.1016/j.biocontrol.2019.02.012 [ Links ]
Villarreal-Delgado MF, Villa-Rodríguez ED, Cira-Chávez LA, Estrada-Alvarado MI, Parra-Cota FI and De los Santos-Villalobos S . 2017. The genus Bacillus as a biological control agent and its implications in the agricultural biosecurity. Mexican Journal of Phytopathology 36(1): 95-130. [ Links ]
Vinale F, Manganiello G, Nigro M, Mazzei P, Piccolo A, Pascale A, Ruocco M, Marra R, Lombardi N, Lanzuise S, Varlese R, Cavallo P, Lorito M and Woo SL. 2014. A novel fungal metabolite with beneficial properties for agricultural applications. Molecules 19(7): 9760-9772. https://doi.org/10.3390/molecules19079760 [ Links ]
Weber T, Rausch C, Lopez P, Hoof I, Gaykova V, Huson DH and Wohlleben W. 2009. CLUSEAN: a computer-based framework for the automated analysis of bacterial secondary metabolite biosynthetic gene clusters. Journal of Biotechnology 140(1-2): 13-17. https://doi.org/10.1016/j.jbiotec.2009.01.007 [ Links ]
Woo SL, Ruocco M, Vinale F, Nigro M, Marra R, Lombardi N, Pascale A, Lanzuise S, Manganiello G and Lorito M. 2014. Trichoderma-based products and their widespread use in agricultura. Open Mycology Journal 8: 71-126. https://doi.org/10.2174/1874437001408010071 [ Links ]
Worley B and Powers R. 2013. Multivariate analysis in metabolomics. Current Metabolomics 1(1):92-107. https://doi.org/10.2174/2213235X11301010092 [ Links ]
Wu TD and Nacu S. 2010. Fast and SNP-tolerant detection of complex variants and splicing in short reads. Bioinformatics 26(7): 873-881. https://doi.org/10.1093/bioinformatics/btq057 [ Links ]
Xi W, Gao Y, Cheng Z, Chen C, Han M, Yang P, Xiong G and Ning K. 2019. Using QC-Blind for Quality Control and Contamination Screening of Bacteria DNA Sequencing Data Without Reference Genome. Frontiers in Microbiology 10: 1-15. https://doi.org/10.3389/fmicb.2019.01560 [ Links ]
Xu Y, Chen H, Zhon X and Cai X. 2012. Induction of hypersensitive response and nonhost resistance by a Cladosporium fulvum elicitor CfHNNI1 is dose-dependent and negatively regulated by salicylic acid. Journal of Integrative Agriculture 11(10): 1665-1674. https://doi.org/10.1016/S2095-3119(12)60169-5 [ Links ]
Yoon SH, Ha SM, Lim J, Kwon S and Chun J. 2017. A large-scale evaluation of algorithms to calculate average nucleotide identity. Antonie van Leeuwenhoek 110: 1281-1286. https://doi.org/10.1007/s10482-017-0844-4 [ Links ]
Zerbino DR and Birney E. 2008. Velvet: algorithms forde novoshort read assembly using de Bruijn graphs. Genome Research 18:821-829. https://doi.org/10.1101/gr.074492.107 [ Links ]
Zhang W, Fraiture M, Kolb D, Löffelhardt B, Desaki Y and Boutrot FF. 2013. Arabidopsis receptor-like protein30 and receptor-like kinase suppressor of BIR1-1/EVERSHED mediate innate immunity to necrotrophic fungi. Plant Cell 25(10): 4227-4241. https://doi.org/10.1105/tpc.113.117010 [ Links ]
Received: September 20, 2020; Accepted: December 21, 2020