Servicios Personalizados
Revista
Articulo
Indicadores
-
Citado por SciELO
-
Accesos
Links relacionados
-
Similares en SciELO
Compartir
Contaduría y administración
versión impresa ISSN 0186-1042
Contad. Adm vol.67 no.1 Ciudad de México ene./mar. 2022 Epub 10-Sep-2024
https://doi.org/10.22201/fca.24488410e.2022.3035
Articles
Structured product to minimize production energy costs by substituting oil with gas
1 Universidad Autónoma Metropolitana, México
2 Instituto Politécnico Nacional, México
This work presents innovations with α-stable processes to value a structured product to minimize energy costs in a given period assuming that an organization selects between oil and gas to produce electricity. The short position of the European put option is to minimize energy costs and the long position of the risk-free bond has a nominal value equivalent to a forward contract and is the estimated energy cost that the organization is willing to pay. The innovation is the valuation of the structured product modeling the underlying returns with an α-stable stochastic process. The performance of the returns is analyzed with descriptive statistics and the α-stable parameters estimation, statistically justifying the relevance of the αstable process with goodness of fit tests. Concluding that hedging for price risk minimizes energy costs, α-stable options are statistically more efficient and less expensive than Gaussian options and that gas is less expensive than oil in the period studied.
JEL Code: C16; C46; C14; D81
Keywords: stochastic processes; financial engineering; structured products
Este trabajo presenta innovaciones con procesos α-estables para valuar un producto estructurado para minimizar costos energéticos en un periodo dado suponiendo que una organización selecciona entre petróleo y gas para producir electricidad. La posición corta de la opción europea de venta es para minimizar los costos energéticos y la posición larga del bono libre de riesgo tiene un valor nominal equivalente a un contrato a plazo (forward) y es el costo energético estimado que la organización está dispuesta a pagar. La innovación es la valuación del producto estructurado modelando el rendimiento subyacente con un proceso estocástico α-estable. El comportamiento de los rendimientos es analizado con las estimaciones de los estadísticos descriptivos y los parámetros α -estables, justificando estadísticamente la pertinencia del proceso α-estable con pruebas de bondad de ajuste. Concluyendo que la cobertura para el riesgo de precio minimiza los costos energéticos, las opciones α-estables son estadísticamente más eficientes y menos costosas que las opciones gaussianas y que el gas es menos costoso que el petróleo en el periodo estudiado.
Código JEL: C16; C46; C14; D81
Palabras clave: Procesos estocásticos; ingeniería financiera; productos estructurados
Introduction
If an organization has a choice between oil and gas to produce electricity, it will use oil when it is less expensive than gas and gas when it is less expensive than oil. That is, the organization uses either energy source to minimize production costs. Substitution of that source generates price competition between oil and gas for supply and demand. The price of gas is usually lower than the price of oil, but there are periods when the relation is inverse.
Hartley et al. (2008) analyze the relation between natural gas and crude oil prices, concluding that the relation between gas and oil prices is indirect, technology is important for the long-term relation between prices, and short-term deviations are influenced by inventories, weather, and seasonal factors. Venegas Martinez (2008) presents the valuation of options with Lévy processes.
Moutinho et al. (2011) analyze the short-run dynamics between commodity prices and electricity prices in Spain from 2002 to 2005 and the long-run relation between the stochastic trends of fuel, gas, coal, and Brent prices. They conclude that in the long run, the relation of gas and Brent prices is correlated and that the price of gas explains the price of electricity.
Dahl et al. (2012) indicate that gas prices in the UK spot market follow a similar pattern as the international oil price, the gas spot market is more liquid, an increasing proportion of gas is used to generate electricity, competing with coal and nuclear, and an increasing level of natural gas is imported. They find that the stochastic trend of Brent is less predominant than that of National Balancing Point gas.
Murphy and Oliveira (2013) examine the pricing of option contracts on the strategic petroleum reserve and consider how it is likely to be used by the government or refineries. The analysis shows that strategic petroleum reserve management produces social welfare.
Atil et al. (2014) analyze the translation of oil prices into gas and gasoline prices, concluding that oil prices influence gas and gasoline prices. Joëts (2014) analyzes price translations in European energy futures markets (oil, gas, coal, and electricity) with maturities of 1, 10, 20, and 30 months in periods of normal and extreme volatility, showing that the increases in energy prices are more marked with extreme volatility, while they are almost nonexistent with normal volatility. In other words, energy markets behave like financial assets, and portfolio diversification is more profitable in the long run.
González Pedraza et al. (2014) analyze the behavior of energy price risk, where the exposure to energy markets is a portfolio of oil, gas, coal, and electricity that models energy returns with generalized hyperbolic conditional distributions with daily energy futures data from August 2005 to March 2012. The analysis shows the importance of leptokurtosis and skewness in the distribution of energy risk factors, concluding that risk measures for energy portfolios with standard methods and in models with exponential extreme decay underestimate risk, particularly for short positions and short-termism.
Stern (2014) indicates that international gas prices reflect the market fundamentals of the 1970s and 1990s, when gas replaced oil and oil derivatives, and that gas and oil are energy sources competing with other energy products, such as coal.
Kilian (2015) indicates that oil price shocks have been recurring since the 1970s. The author shows the evolution of the U.S. oil price from a historical perspective and compares it with the price of coal and gas, concluding that positive oil shocks are associated with recessions in importing countries and that an increase in the oil price is a sign of scarcity and a precondition for the development and adoption of alternative energy technologies.
Schöne (2015) indicates that modeling the cash flows of an investment project deserves attention in the valuation of real options on commodities where an empirical study reveals that prices are not stationary and have returns that do not exhibit a Gaussian distribution. The results suggest that the choice of the stochastic process has significant implications for valuation and optimal investments.
Gatfaoui (2015) states that the prices of energy products are based on factors such as development and delivery costs, i.e., supply. The balance between demand and supply determines the prices of energy products that depend on seasonal and periodic weather effects (temperature, rainfall, or humidity). Supply and demand depend on production costs. Electricity generation is the result of transforming fossil fuels (oil, gas, and coal), so there is a correlation among the prices of electricity, oil, and gas: consumers use gas compared to oil products. Gas consumption depends on the price differential with oil. Gas consumption generates efficiency gains in the transportation industry, while the heavy truck sector has tax incentives to substitute oil with gas. The electricity sector chooses oil or gas as an energy source to produce electricity, managing substitution opportunities. The substitution process requires investment (equipment or technology), which explains the limitations of the opportunities for substituting oil or oil products with gas after 2005 and that result from the investments in infrastructure necessary to enable substitution. Substitution between oil and gas establishes implicit gas prices and price competition between the two energy sources. The ability of consumers to switch from oil to gas or from gas to oil contributes to the stabilization process. Organizations arbitrage the oil and gas price differential to accommodate the efficiency differences between oil and gas as energy sources. In New York and New England, approximately 30% of electricity generation is a result of energy switching, and given the shortage of gas in cold periods, increases in energy consumption are generated and gas is the main energy source. Hence, power plants use oil to produce electricity and satisfy energy switching by compensating for the shortfall in gas supply.
Shahmoradi and Swishchuk (2016) indicate that oil price returns exhibit a distribution with heavy extremes and skewness (leptokurtosis and skewness). They then use the inverse Gaussian process, the diffusion process with jumps, and the gamma variance process as three Lévy processes with extremes with a higher frequency than the Gaussian distribution. They use the fast fractional Fourier transform to calibrate the parameters with data from European options on oil futures and conclude that these three Lévy processes perform best out of the sample of at-the-money options. Václavík and Klimešová (2016) analyze the valuation of gas-exchange options, which are a set of put options on a spread between the prices of various energy commodities, and indicate that the valuation model is important.
Arrigoni et al. (2019) state that the Canadian government introduced a carbon tax to reduce greenhouse gas emissions. They define a carbon pricing approach and carbon price as the tax needed to motivate electricity producers to switch from coal to natural gas. They model prices under three stochastic procedures: inverse Gaussian, Gaussian, and Heston, concluding that the inverse Gaussian process outperforms the Gaussian and Heston processes because it considers the nature of energy prices.
Hilliard and Hilliard (2019) develop a diffusion model with jumps for valuation and hedging with margined options on Brent futures. Model parameter estimation and out-of-sample pricing errors are calculated using data on Brent crude oil contracts. They conclude that an option on an underlying equity is effectively hedged by a portfolio with two margined options and the underlying.1
Fang and Chag (2020) use the fast fractional Fourier transform to analyze the efficiency of option pricing with the Lévy process. The results indicate that compared with the Gaussian model, the calibration errors for the Lévy process are smaller. They conclude that the parameter estimation makes the price valuation more accurate, reducing the risk of poor option valuation.
This paper is organized as follows: in section 2, the procedure for structuring the portfolio with the risk-free bond and the European put option on the relation between gas and oil prices is explained, and the definition of the α-stable distributions and the proposed model for the valuation of the European αstable put options are presented. In section 3, the analysis of the distribution of returns is carried out with the estimation of the descriptive statistics and the α-stable parameters; the Kolmogorov and Smirnov and Anderson and Darling goodness-of-fit tests are performed to justify the relevance of the α-stable distribution; and the fits of the Gaussian, α-stable, and t-Student distributions with the absolute frequencies of the returns are presented. In section 4, the European put options are valued, and a sensitivity analysis is performed. Finally, section 5 presents the conclusions and the bibliographical references.
Structured product
This section presents the theoretical framework and explains the procedure for the valuation of the European option to swap oil for gas.
Put option to substitute oil with gas
This study assumes an organization with a dual-energy infrastructure to alternately use two energy sources to operate a power plant and produce electricity. The organization generates electricity by switching between oil and gas. Then the power plant plans the future switching date to use an alternative energy source to cover consumption increases and compensate for supply shortages in the initial energy source.
Assuming that the organization uses oil or gas as a source of energy for production, then the organization has the following scenarios:
Buying oil when the price of oil is lower than the price of gas.
Buy gas when the price of oil is greater than or equal to the price of gas.
Assuming that the organization establishes an energy strategy for a given period and the energy choice is made at a future date T, then the organization optimizes energy costs at future date T by purchasing the least expensive energy source, and the optimization strategy is equivalent to forecasting the trend of energy costs in the short or medium term.
If GT and PT are the oil and gas prices at date T, when the energy choice decision is made, and if CT is the energy cost that the organization forecasts while making the energy decision at moment T, then the organization has the following scenarios:
The organization's energy cost at moment T is the minimum between the price of gas and the price of oil, equivalent to the following scenarios:
The resulting energy cost at moment T is:
Where MT is the underlying price, and S = 1 is the settlement price at maturity. Then, the organization uses oil when the price of oil is lower than the price of gas and uses gas when the price of gas is lower than the price of oil. Therefore, the organization's energy cost includes the price of a European option to substitute oil with gas as an energy source. The energy cost in Equation (1) is written, equivalently, as:
where S−max(S −MT, 0) is a portfolio formed by the long position of a risk-free bond and the short position of a European put option on the underlying (relation between the price of gas and the price of oil) with settlement price at maturity S=1 and maturity at moment T, i.e., a product structured on the relation between the price of gas and the price of oil.
The European put option is an option to substitute oil with gas because it is exercised when the price of gas is lower than the price of oil at moment T. Then the organization's energy cost is the long position of a risk-free bond with a face value equal to the price of oil at moment T, and the short position of a European put option to exchange oil for gas equal to the price of oil. Therefore, the option to substitute oil with gas minimizes the organization's energy costs compared to an oil-only energy policy. The energy cost of Equation (2) is equivalently written as:
where
The energy hedging strategy has the following patterns:
The decision date is set to define the horizon of the hedging strategy.
The short position of the European option to change the energy source is taken prior to the decision regarding the choice of energy sources.
Therefore, option valuation is necessary to structure the hedging strategy. The valuation of the European option quantifies the organization's savings through the ability to substitute the energy source. Therefore, the energy price uncertainty is modeled, and consequently, the risk is quantified with an option to optimize the organization's energy cost.
α-stable distributions
The α-stable distributions are characterized by four parameters and are
denoted by S(α, β, γ, δ) where the stability parameter 0 < α ≤ 2
indicates the degree of leptokurtosis and the slope with which the extremes
of the distribution decrease. The skewness parameter -1 ≤ β ≤ 1 indicates
the degree of asymmetry, and the scale parameter γ = 0 indicates the units
of dispersion for the location parameter -∞ < δ < ∞, which indicates
the location point and the distribution mode. The α-stable distributions
generally do not have a closed analytical expression to characterize the
random variable, but the characteristic function
where
The cumulant function of the random variable Z has the following form:
where
The α-stable distributions have closed analytical expressions for the following densities:
Valuation of european α-stable put options
The properties of historical returns allow the properties of risk-neutral returns to be deduced to perform the valuation of options considering stylized events and real market conditions. Consequently, in an incomplete and risk-free market, the price of the European put option is calculated as the present value of the expected settlement payment with respect to the risk-neutral measure:
where
Schöne (2015) and Gatfaoui (2015) indicate that the cash flows of an investment project deserve attention because an empirical study reveals that energy source prices exhibit returns without a Gaussian distribution. Thus the choice of the stochastic process has significant implications for the valuation of options and optimal investments. Therefore, the first innovation presented in this paper is to assume that the distribution of returns is α-stable, the second is to justify its suitability statistically, and the third is the valuation of the European option on the proposed index with the following α-stable model:
with:
where
The model applied is similar to the one proposed by Contreras Piedragil and Venegas Martínez (2011) and Rodríguez Aguilar and Cruz Aké (2013) and is based on the valuation of the structured guaranteed equity product proposed by Climent Hernández and Cruz Matú (2017), where the parameters of the α-stable distributions are α, β and γ. Considering that the bounds for the valuation of European put options are:
where the valuation of European put options is arbitrage-free and considers
a leptokurtic and asymmetric distribution of returns. The European put
option to exchange oil for gas is exercised when the relation between gas
and oil prices is less than unity
Analysis of the distribution of returns
With the data under consideration, the relation between the gas and oil prices with the data sample is calculated.
Oil and gas price returns
The daily sample data are from January 7, 1997, to July 19, 2019, with 5 350 observations for each series2, considering the Mexican export crude oil blend price in dollars per barrel and the Henry Hub natural gas price in dollars per million British thermal units. The gas and oil price relation is calculated with the thermal conversion of gas to equivalent oil barrels; according to Gatfaoui (2015), one oil barrel represents 5.65853 million British thermal units. Next, the gas price equivalent to the oil price is calculated by multiplying the gas price by 5.65853, and the relation of gas and oil prices is calculated as the quotient between gas price and oil price and the logarithmic yields are calculated as:
where
Table 1 Descriptive statistics
mín(R) | máx(R) |
|
|
|
SR | g1 | g2 |
---|---|---|---|---|---|---|---|
-0.5859 | 0.5594 | -0.0003 | -0.0004 | 0.0000 | 0.0503 | 0.4528 | 15.4672 |
Source: created by the authors with data from Banxico and EIA
Table 1 presents the descriptive statistics, and the following hypotheses are proposed:
Estimation of α-stable parameters
The returns analysis indicates that the distributions are leptokurtic and skewed. Next, the estimation of αstable parameters is performed with the maximum likelihood method with confidence intervals of 95 % and presented in Table 2.
Table 2 Estimation of α-stable parameters
α | β | γ | δ |
---|---|---|---|
1.6569±0.0415 | 0.0072±0.1071 | 0.0254±0.0007 | -0.0007±0.0012 |
Source: created by the authors with data from Banxico and EIA
Table 2 presents the stability and skewness parameters that indicate that the distribution of returns is leptokurtic, with positive skewness approaching zero and negative location parameter also approaching zero, so the distribution of returns is leptokurtic and symmetric around the origin.
Goodness-of-fit tests
The quantitative analysis to test the hypothesis that the returns are α-stable with the Kolmogorov and Smirnov goodness-of-fit statistic is presented in Table 3.
Table 3 Kolmogorov and Smirnov tests (95 %)
Distribution | P (D>d) | D |
---|---|---|
Gaussiana | 0.0000 | 0.0764 |
α-estable | 0.4034 | 0.0122 |
t-Student | 0.9218 | 0.0075 |
VEG | 0.0000 | 0.1831 |
Source: created by the authors with data from Banxico and EIA
Table 3 presents the descriptive significance levels that indicate not to reject the hypotheses that the returns are α-stable or t-Student, and indicate to reject the hypotheses that the returns are Gaussian or generalized outliers. The quantitative analysis to test the hypothesis that the returns are α-stable is supplemented with the Anderson and Darling statistic and presented in Table 4.
Table 4 Anderson and Darling tests (95 %)
Distribution | P (A2> a2) | D |
---|---|---|
Gaussiana | 0.0000 | ∞ |
α-estable | 0.4710 | 0.8140 |
t-Student | 0.9156 | 0.3281 |
VEG | 0.0000 | ∞ |
Source: created by the authors with data from Banxico and EIA
Table 4 presents the descriptive significance levels that indicate not to reject the hypotheses that the returns are α-stable or t-Student, and indicate to reject the hypotheses that the returns are Gaussian or generalized outliers.
Distribution fits
The fits of the a-stable, t-Student and Gaussian distributions to the absolute frequencies of the returns are presented in Figure 1.
The results in Tables 3 and 4 indicate that α-stable or t-Student3 distributions are more efficient than Gaussian or generalized extreme value distributions for modeling the empirical behavior of returns. Therefore, α-stable distributions are relevant for modeling returns and managing market risks (price risk).
Figure 1 presents the fits to the distributions, and the fits indicate that the estimation of the α-stable distribution is relevant for the valuation of the European put option on the relation of gas and oil prices.
Valuation of the european put option
Estimating α-stable parameters for risk-neutral density and goodness-of-fit tests justifies the valuation of European put options on the relation of gas and oil prices to exchange oil for gas. Then, with the data of July 19, 2019, the valuations of European put options are performed with the following initial factors: M0 = 0.2284 , γ = 0.7148, i = 0.0822, r = 0.0188 and S =1 with hedging business periods from July 22, 2019, to March 13, 2020.
Options are a contingent right dependent on the underlying price at the maturity date, so the valuation of European options is performed with the Gaussian and α-stable models. The performance of the underlying during the hedging period is presented in Figure 2.
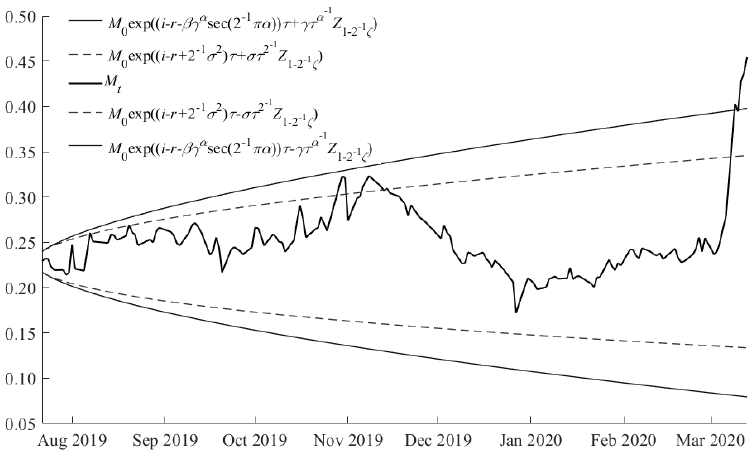
Source: created by the authors with data from Banxico, Federal Reserve, and EIA
Figure 2 Oil and gas relation performance
Figure 2 presents the performance of the
underlying, which indicates that on March 11, 12, and 13, 2020, the underlying
breaches the upper bound of the α-stable confidence interval of 99% because the
price of oil declined from 35.75 to 25.93. If the underlying price is greater
than or equal to the settlement price (MT ≥
S) then the structured product (portfolio) has bounded
profits because the long position of the bond and the obligations for the
settlement payment of the short option position are zero; therefore, the
portfolio's value is
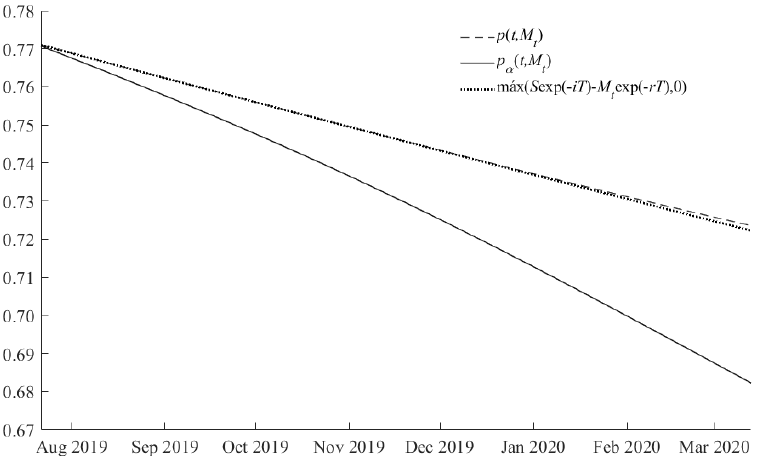
Source: created by the authors with data from Banxico, Federal Reserve, and EIA
Figure 3 Valuation of European put options
Figure 3 presents the valuation of
Gaussian, a-stable, and lower bound options for the hedging business period and
indicates that Gaussian options overestimate price risk, and α-stable options
minimize arbitrage opportunities because the price of European α-stable put
options written on the relation between gas and oil prices is the lower bound of
Equation (9), i.e.,
Table 5 Valuation of European put options
Maturity |
|
|
|
|
|
---|---|---|---|---|---|
16/08/2019 | 0.7657 | 0.7629 | 0.7657 | 0.7474 | 1.0231 |
20/09/2019 | 0.7583 | 0.7514 | 0.7583 | 0.7833 | 0.9858 |
18/10/2019 | 0.7524 | 0.7417 | 0.7524 | 0.7447 | 1.0233 |
15/11/2019 | 0.7466 | 0.7314 | 0.7466 | 0.6957 | 1.0712 |
13/12/2019 | 0.7410 | 0.7205 | 0.7408 | 0.7652 | 1.0006 |
17/01/2020 | 0.7340 | 0.7061 | 0.7336 | 0.7873 | 0.9770 |
14/02/2020 | 0.7287 | 0.6943 | 0.7279 | 0.7688 | 0.9944 |
13/03/2020 | 0.7236 | 0.6822 | 0.7223 | 0.5462 | 1.2158 |
Source: created by the authors with data from Banxico, Federal Reserve, and EIA
Table 5 presents the valuation of the
Gaussian, α-stable, lower bound, intrinsic value, and portfolio utility options,
and indicates that the value of the options decreases as the hedge period
increases, the underlying prices are less than the settlement price
(S =1) in the short term, the proposed hedge is efficient
in the short term, the savings (intrinsic value) from the energy cost decrease
in inverse proportion with the increase in the underlying price, and the
portfolio profits increase in direct proportion with the increase in the
underlying price. The structured product has a minimum guaranteed principal
equivalent to the valuation of put option

Source: created by the authors with data from Banxico, Federal Reserve, and EIA
Figure 4 . Intrinsic value
Figure 4 presents the energy cost savings and indicates that they are equivalent to the payoff and
decrease in inverse proportion to the increase in the underlying price. The
proposed hedge of the structured product is efficient because when the
underlying price exceeds the upper limit of the confidence interval, the
portfolio allows the trading of oil for gas and obtaining a profit equivalent to
the product of the oil price and the put option on the maturity
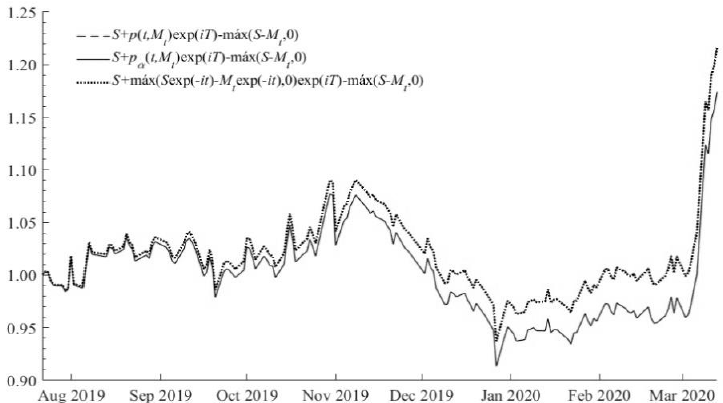
Source: created by the authors with data from Banxico, Federal Reserve, and EIA
Figure 5 Value of the structured product
Figure 5 presents the value of the
structured product at maturity date and indicates that the portfolio's value is
Sensitivity analysis
The sensitivity analysis evaluates the efficiency of the structured product for the organization's energy hedging, or equivalently, the ability to exchange oil for gas in the short term. The valuation of options where the underlying price varies in the interval 0 ≤ Mt ≤ 1.5 and with hedging periods in the interval 1 ≤ T ≤ 365 days is presented in Figure 6.
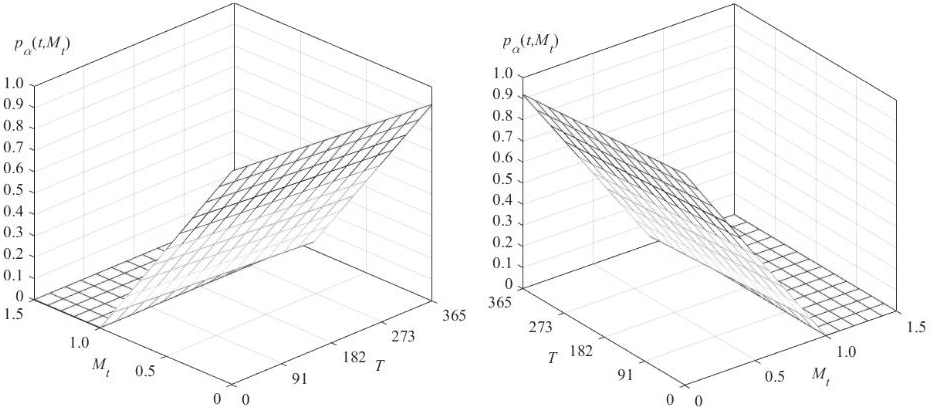
Source: created by the authors with data from Banxico, Federal Reserve, and EIA
Figure 6 Sensitivity analysis with respect to period and price
Figure 6 presents the valuation of the
options as a function of the hedge period and underlying price and confirms
that the value of the options decreases as the hedge period increases; it
decreases as the underlying price on the trade date increases to equal the
settlement price where the options have zero value. The strategy is
effective, and the valuation of the α-stable options adequately quantifies
the price risk because when the options are traded in-the-money
(Mt < S), then the value of the
structured product on the expiration date allows oil to be traded for gas
and the profit is

Source: created by the authors with data from Banxico, Federal Reserve, and EIA
Figure 7 Probability of exercise with respect to period and price
Figure 7 presents the probabilities of options being in-the-money as a function of the hedge period and the underlying price and indicates that the probabilities of trading oil for gas decrease as the underlying price increases and increase as the hedge period increases; so the probabilities of options being out-of-the-money are decreasing with respect to the underlying price. Therefore, the probabilities of the options being out-of-the-money are decreasing with respect to the underlying price and increasing with respect to the hedging period. The valuation of options with varying volatility in interval 0.1 ≤ γ ≤ 0.9 and with hedging periods in interval 1 ≤ T ≤ 365 days is presented in Figure 8.
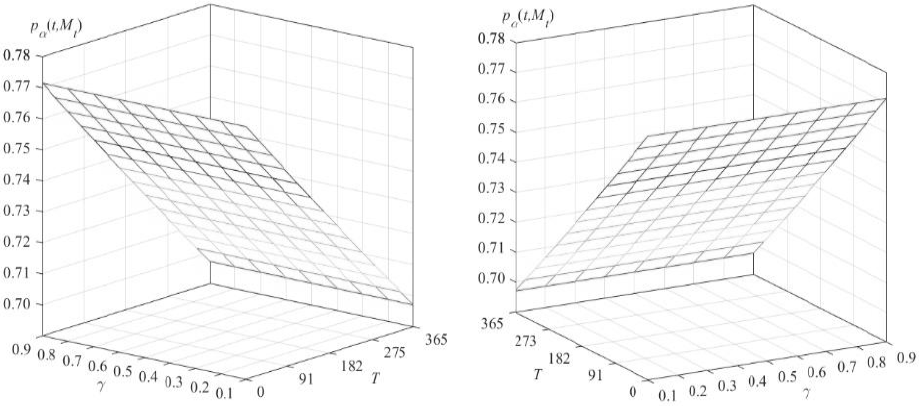
Source: created by the authors with data from Banxico, Federal Reserve, and EIA
Figure 8 Sensitivity analysis with respect to period and volatility
Figure 8 presents the valuation of
options as a function of the hedge period and the scaling parameter. It
confirms that the value of the options decreases as the hedging period
increases and is constant with the change in volatility because the lower
bound on the value of the European put options is

Source: created by the authors with data from Banxico, Federal Reserve, and EIA
Figure 9 Sensitivity analysis with respect to period and rate
Figure 9 presents the valuation of the options as a function of the hedge period and interest rate and indicates that the value of the options is a convex function that decreases as the hedge period or interest rate increase. The valuation of the options varying the settlement price in the interval 0.1 ≤ S ≤ 1 and with hedging periods in the interval 1 ≤ T ≤ 365 days is presented in Figure 10.
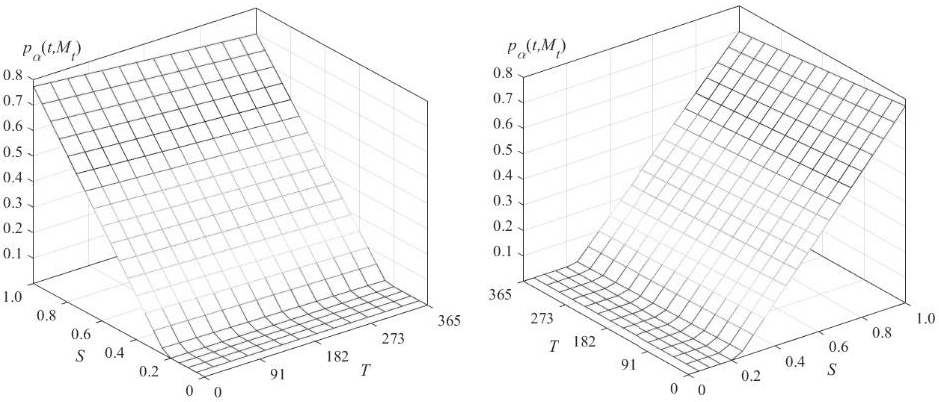
Source: created by the authors with data from Banxico, Federal Reserve, and EIA
Figure 10 Sensitivity analysis with respect to period and settlement price
Figure 10 presents the valuation of the options as a function of the hedging period and the settlement price presented, and indicates that the value of the options is a convex function that decreases as the hedging period increases, and increases as the interest rate increases.
The sensitivity analysis indicates that the savings and energy costs of switching from oil to gas or continuing to use oil to produce electricity, respectively, are maximum when the underlying price approaches zero and minimum when the underlying price approaches the settlement price. The organization's strategy using the structured product is financially efficient, even when the settlement price is less than unity because the value of the portfolio minus the energy cost of production is:
Then, if the price of gas is lower than the price of oil, the organization's
profit is
The above results exhibit that α-stable options are statistically more efficient than Gaussian options in quantifying market risk, specifically price risk. The prices and profits of α-stable options are lower than those of Gaussian options, and the use of gas is less costly than the use of oil to generate electric power during the study period.
Conclusions
The structured product is analyzed as a diversified portfolio with a long position in a domestic risk-free bond and a short position in a European put option issued on the relation between the price of gas and the price of oil with a risk and return profile that is difficult to replicate with doubtful or capital market products and provides access to a synthetic underlying.
Investors are generally unaware of the characteristics or valuation method, and their information is focused on the payout diagrams and not on the probability of the contingent payout of the portfolio. On the other hand, the issuers of the structured product hedge implicit risks by using derivative products, in this case, a European put option.
The structure provides policies to minimize production costs to increase the competitiveness of electricity-producing organizations. Changes in the price of oil generate changes in the energy costs of electricity production that have economic consequences because energy costs are reflected in profits and consumption.
The opportunity assumption to substitute oil with gas persists in the face of changes in oil and gas prices, and the creation of hedges to minimize production costs generates savings that promote competition. Parameter estimation and fit to the α-stable distribution confirm the ability to substitute one energy source with an alternative source with a lower price and the innovation with a guaranteed capitalstructured product with the statistical justification of a stochastic α-stable process of the underlying return because the descriptive statistics indicate that the underlying returns are leptokurtic and skewed. The Gaussian assumption of the analyzed returns is rejected with goodness-of-fit tests, whereas they do not reject the α-stable hypothesis. Therefore, the α-stable process applied to the valuation of the European put option is relevant and statistically justified. Therefore, the proposed energy strategy shows that α-stable options are statistically more efficient than Gaussian options in quantifying price risk, in which the gains obtained by European α-stable options are lower than those obtained by Gaussian options.
Sensitivity analysis indicates that the structured guaranteed equity product efficiently minimizes costs and allows investors to participate in the potential gains of the underlying price while hedging against potential losses. The European put option quantifies the organization's savings from substituting the energy source of production, allowing it to earn a higher return than the national risk-free interest rate. The structured guaranteed equity product maximizes the value of the investors' assets with the following limitations:
The portfolio structure is an imperfect hedge, i.e., there is a risk of making wrong decisions.
A significant portion of the investment is made in the risk-free interest rate.
In future studies, it would be possible to justify the valuation of t-Student options if the relevance is statistically justified or to evaluate an investment project, including the initial investment costs for the facilities that allow the change of the energy source, production costs, electricity distribution revenues, and profits by applying measures such as net present value, internal rate of return, benefit-cost ratio, and real option valuation.
REFERENCES
Arrigoni, A., Lu, W., Swishchuk, A. y Goutte, S. (2019). Energy-Switching using Lévy processes -an application to Canadian and North American data, consultado el 02/03/2020 y disponible en: Energy-Switching using Lévy processes -an application to Canadian and North American data, consultado el 02/03/2020 y disponible en: https://ssrn.com/abstract=3408174 . http://dx.doi.org/10.2139/ssrn.3408174. [ Links ]
Atil, A., Lahiani, A. y Nguyen, D. (2014). Asymmetric and non linear pass-through of crude oil prices to gasoline and natural gas prices. Energy Policy, 65(2): 567-573. https://doi.org/10.1016/j.enpol.2013.09.064. [ Links ]
Cassidy, D. T., Hamp, M. J. y Ouyed, R. (2010). Pricing European options with a log Students tdistribution: A Gosset formula. Physica A: Statistical Mechanics and its Applications, 389(24): 5736-5748. https://doi.org/10.1016/j.physa.2010.08.037. [ Links ]
Climent Hernández, J. A. y Cruz Matú, C. (2017). Pricing of a structured product on the SX5E when the uncertainty of returns is modeled as a log-stable process. Contaduría y Administración, 62(4): 1160-1182. https://doi.org/10.1016/j.cya.2017.06.012. [ Links ]
Contreras Piedragil, C. E. y Venegas Martínez, F. (2011). Valuación de opciones sobre activos subyacentes con distribuciones estables. Estocástica: Finanzas y Riesgo, 1(1): 55-71. [ Links ]
Dahl, R., Oglend, A., Osmundsen, P. y Sikveland, M. (2012). Are oil and natural gas going separate ways in the United Kingdom? Cointegration tests with structural shifts. Journal of Energy Markets, 5(2): 33-58. https://doi.org/10.21314/JEM.2012.073. [ Links ]
Fang, M. y Chang, C. (2020). Fractional Fast Fourier Transform on Pricing Cruel Oil Options under Lévy Process, Cyber Security Intelligence and Analytics, 249-254. Springer, Cham. https://doi.org/10.1007/978-3-030-43309-3_34. [ Links ]
Gatfaoui, H. (2015). Pricing the (European) optiontos witch between two energy sources: An application to crude oil and natural gas. Energy Policy , 87(12): 270-283. https://doi.org/10.1016/j.enpol.2015.09.010. [ Links ]
González Pedraza, C., Moreno, M. y Peña, J. I. (2014). Tail risk in energy portfolios, Energy Economics, 46: 422-434. https://doi.org/10.1016/j.eneco.2014.05.004. [ Links ]
Hartley, P. R., Medlock III, K. y Rosthal, J. (2008). The relationship of natural gas to oil prices. Energy Journal, 29(3): 47-65. https://doi.org/10.5547/ISSN0195-6574-EJ-Vol29-No3-3. [ Links ]
Hilliard, J. E. y Hilliard, J. (2019). A jump-diffusion model for pricing and hedging with margined options: An application to Brent crude oil contracts, Journal of Banking & Finance, 98: 137-155. https://doi.org/10.1016/j.jbankfin.2018.10.013. [ Links ]
Joëts, M. (2014). Energy price transmissions during extreme movements. Economic Modelling, 40(6): 392-3399. https://doi.org/10.1016/j.econmod.2013.11.023. [ Links ]
Kilian, L. (2015). Energy Price Shocks. The New Palgrave Dictionary of Economics. London: Palgrave Macmillan: 1-12. ISBN: 978-1-349-95121-5. https://doi.org/10.1057/978-1-349-95121-5_3008-1. [ Links ]
Murphy, F. y Oliveira, F. S. (2013). Pricing option contracts on the strategic petroleum reserve, Energy Economics , 40: 242-250. http://dx.doi.org/10.1016/j.eneco.2013.06.016. [ Links ]
Moutinho, V., Vieira, J. y Moreira, A. C. (2011). The crucial relationship among energy commodity prices: Evidence from the Spanish electricity market. Energy Policy, 39(10): 5898-5908. https://doi.org/10.1016/j.enpol.2011.06.043. [ Links ]
Rodríguez Aguilar, R. y Cruz Aké, S. (2013). Valuación de opciones de tipo de cambio asumiendo distribuciones α-estables. Contaduría y Administración , 58(3): 149-172. https://doi.org/10.1016/S0186-1042(13)71225-7. [ Links ]
Shahmoradi, A. y Swishchuk, A. V. (2016). Pricing crude oil options using Lévy processes, Journal of Energy Markets , 9(1): 47-63. http://dx.doi.org/10.21314/JEM.2016.140. [ Links ]
Schöne, M. (2015). Real Options Valuation: The Importance of Stochastic Process Choice in Commodity Price Modelling. Springer. ISBN: 978-3-658-07492-0. [ Links ]
Stern, J. (2014). International gas pricingin Europe and Asia: a crisis of fundamentals. Energy Policy , 64(1): 43-48. https://doi.org/10.1016/j.enpol.2013.05.127. [ Links ]
Václavík, T. y Klimešová, A. (2016). Gas swing options: Introduction and pricing using Monte Carlo methods, Acta Oeconomica Pragensia, 2016(1): 15-32. https://doi.org/10.18267/j.aop.496. [ Links ]
Venegas Martínez, F. (2008). Riesgos financieros y económicos: productos derivados y decisiones económicas bajo incertidumbre, Cengage Learning Latin America. Segunda edición. [ Links ]
2
Oil price from www.banxico.org.mx/apps/gc/precios-spot-del-petroleo-gra.html.
Gas price from www.eia.gov/dnav/ng/hist/rngwhhdd.htm.
3 This paper does not aim to perform option valuation with t-Student processes as proposed by Cassidy et al. (2010).
Received: August 28, 2020; Accepted: August 27, 2021; Published: August 31, 2021