1. Introduction
Climate change has severe impacts on agriculture and related sectors. Climate is a limiting factor in agricultural production whose impacts can be more pronounced in the future (Piao et al., 2010; Zuzulova and Vido, 2018). Drought, one of the impacts of climate change, is a decrease in the amount of rainfall received over a period of time (Mishra and Singh, 2010, 2011; Azmi et al., 2016). Therefore, proper planning may reduce drought risk (Dai, 2011; Trenberth et al., 2014).
Drought is caused by high temperatures, low relative humidity, strong winds, lack of rainfall, and improper distribution throughout the year (Bazrafshan and Hijabi, 2017). According to the WMO definition, drought has been classified based on rainfall, rainfall combined with temperature and humidity, soil and crop moisture parameters, and climatic indices, as well as estimation of evapotranspiration (Bokusheva et al., 2016).
The drought phenomenon begins with the lack of rainfall (meteorological drought). This deficiency’s continuation impacts soil moisture, plant growth, and yield (agricultural drought). In the future, a hydrological drought will appear and disrupt the hydrological balance. Finally, the socio-economic effects will vary depending on the water resources used (socio-economic drought) (Huang et al., 2016; Faiz et al., 2018, 2020; Mukherjee et al., 2018; Yihdego et al., 2019). As mentioned, drought has some indicators (precipitation, temperature, streamflow, groundwater and reservoir levels, soil moisture, and snowpack) that describe drought conditions. Besides, many climatic, hydrological, and remote sensing drought indices are typically computed numerical representations of drought severity, assessed using climatic or hydrometeorological inputs. Drought indices aim to measure the qualitative state of droughts on the landscape for a given period, identify dry and wet periods, and monitor the intensity, frequency, and severity of these events (WMO-GWP, 2016). Given the difficulties in defining drought, its understanding can be facilitated through drought indices, which help monitor these events’ intensity, frequency, and severity.
In recent decades, due to the limitations of drought monitoring using climatic and hydrological indices (including the spatial distribution of meteorological and hydrometric stations, especially in arid areas), remote sensing (RS) techniques have been considered (Ebrahimi et al., 2010; Heim, 2002). Accurate image processing and calculating indices can improve the model efficiency for drought prediction (Rhee et al., 2010).
Several researchers have studied drought using RS techniques. For instance, Helali et al. (2022) investigated drought and its effects on vegetation using remote sensing data in the Urmia Lake basin, Iran. Their results showed that the Standardized Precipitation Index (SPI) correlates well with the Normalized Difference Vegetation Index (NDVI). Also, the NDVI and the Normalized Difference Water Index (NDWI) were more suitable for monitoring vegetation and water extent changes. Mikaili and Rahimzadegan (2022) investigated local drought using remote sensing indices in Fars province, Iran, showing that NDVI-based indices can monitor and assess drought on a long-time scale.
Attafi et al. (2021) investigated drought using SPI and NDVI in a region of Iraq. They found that increasing the severity of drought leads to reduced crop production and that NDVI can model the effects of drought on crop production. Lee et al. (2021) studied a region of the USA and concluded that, among several analyzed indices, the Integrated Crop Drought Index (ICDI) could well reflect agricultural drought. Indeed, it can be a good option for agricultural drought monitoring and yield management.
Amin et al. (2020) investigated drought using several drought indices for the Tal Punjab region, Pakistan, during spring. They determined that 28, 12, and 60% of the area is affected by slight, moderate, and non-existent drought, respectively. Marumbwa et al. (2020) evaluated the Standardized Precipitation Evapotranspiration Index (SPEI), the Vegetation Condition Index (VCI), and the NDVI on different types of land cover in South Africa. They revealed that evergreen forests were affected by the 2015-2016 drought, and the lowest VCI values in rural land cover indicated the vulnerability of rural communities to drought. Sun et al. (2020) evaluated six drought indices in a region of China and showed that drought during the summer could delay vegetation growth, which varies from one to six months, depending on the specific type of vegetation. In other research, Wang et al. (2020) evaluated six drought indices in China and showed that SPI was the most appropriate index for assessing drought in steppes and deserts within the study area. Yaghoobzadeh et al. (2017) assessed the effect of climate change on agricultural drought using SPI and the Evapotranspiration Deficit Index (ETDI) in the Neishabour Plain, Iran. They recommended using ETDI since agricultural drought depends on soil water deficits.
There are three main methods (including using a single index, multiple indices, and composite or hybrid indices) for monitoring drought and guiding early warning and assessment (WMO-GWP, 2016). Several studies recommend comparing the results of several indices instead of a single drought index. Since Iran is located in one of the Earth’s dry belts and has experienced many droughts, the objectives of this study are:
analysis using the drought indices NDVI, Soil Adjustment Vegetation Index (SAVI), and Simple Ratio (SR) in three regions with different climatic conditions. These indicators are among the main and basic remote sensing indicators. All indices used in this study highlight various aspects of drought and are compared with indices such as SPEI.
Drought assessment using meteorological SPI, the agricultural drought index SPEI, and the Palmer Drought Intensity Index (PDSI) in three regions with different climatic conditions.
Investigating the relationship and correlation between remote sensing drought indices (NDVI, SAVI, and SR) and meteorological and agricultural drought indices (SPI, SPEI, and PDSI).
2. Methods
2.1 Study area and required data
Iran is located in Asia between 25º-40º N and 44º-64º E, comprising an area of more than 1 648 000 km2 (Ramezani et al., 2023). The climate of Iran includes the four seasons of the year in most parts. Due to low rainfall and high evaporation, most of the country’s area is located in arid and semi-arid regions (Rahnama et al., 2022). Figure 1 displays the distribution of study areas throughout Iran. Birjand is located on the edge of the desert, Shiraz is in the mountainous regions with a semi-arid climate, and Rasht is in the north of Iran with a humid climate. All these regions represent distinctive climate conditions in Iran. Each of the three regions is located in a different part of Iran, comprising a large area within its neighborhood. Based on climate diversity and the de Martonne system (de Martonne, 1926; Aguirre et al., 2018), these areas were selected as very humid (Rasht), semi-arid (Shiraz), and dry (Birjand) climates. The geographical characteristics, surface area, and climate of these regions are presented in Table I.
Table I Geographical characteristics of study areas and their climate in the de Martonne climate classification (de Martonne, 1926) during 2002-2020.
Area | Longitude (E) | Latitude (N) | Elevation above sea level (m) | Area (km2) | Precipitation (mm) | Average temperature (°C) | Type of climate |
Rasht | 049º35’20” | 37º16’05” | -8.6 | 1341.12 | 1282.52 | 16.44 | Very wet |
Shiraz | 052º35’00” | 29º35’33” | 1484.0 | 515.653 | 326.74 | 18.45 | Semi-arid |
Birjand | 059º13’34” | 32º51’53” | 1491.0 | 3406.72 | 152.04 | 16.48 | Arid |
In this study, Landsat images (TM, ETM+, and OLI sensors) for 2002 and 2014-2020 were used due to their suitable spatial resolution bands with different wavelengths (http://earthexplorer.usgs.gov). Cloud cover and air pollution are the main problems in calculating indices such as NDVI; for instance, high cloud cover can affect a pixel with high vegetation density. The Landsat ETM images were acquired from 2003 to 2013 but due to the unsuitable quality of the images from the study area, we did not use them. The images of 2002 were used to indicate land use changes during these years. The spectral characteristics of Landsat bands are presented in Table II. So, in this study first the necessary corrections (including radiometric [Radiometric Calibration] and atmospheric corrections [QUick Atmospheric Correction]) were performed using ENVI 5.3 software, and then the vegetation indices (including NDVI, SAVI, SR) were calculated. Finally, Arc GIS 10.2 software was used to map the vegetation of the areas.
Table II Spectral characteristics of Landsat (NASA, 2013).
Spectral band | Wavelength (μm) | Resolution (m) | |
Landsat 8 | Band 1-coastal/aerosol | 0.433-0.453 | 30 |
Band 2-Blue | 0.450-0.515 | 30 | |
Band 3-Green | 0.525-0.600 | 30 | |
Band 4-Red | 0.630-0.680 | 30 | |
Band 5-NIR | 0.845-0.885 | 30 | |
Band 6-SWIR 1 | 1.56-1.66 | 30 | |
Band 7-SWIR 2 | 2.10-2.30 | 30 | |
Band 8-Panchromatic | 0.50-0.68 | 15 | |
Band 9-Cirrus | 1.36-1.39 | 30 | |
Band 10-Thermal 1 | 10.30-11.30 | 100 | |
Band 11-Thermal 2 | 11.50-12.50 | 100 | |
Landsat 7 | Band 1-Blue | 0.45-0.52 | 30 |
Band 2-Green | 0.52-0.60 | 30 | |
Band 3-Red | 0.63-0.69 | 30 | |
Band 4-NIR | 0.76-0.90 | 30 | |
Band 5- SWIR | 1.55-1.75 | 30 | |
Band 6-Thermal | 10.40-12.30 | 60 | |
Band 7-Min infrared | 2.08-2.35 | 30 | |
Band 8-Panchromatic | 0.52-0.90 | 15 | |
Landsat 4-5 TM | Band 1-Blue | 0.45-0.52 | 30 |
Band 2-Green | 0.52-0.60 | 30 | |
Band 3-Red | 0.63-0.69 | 30 | |
Band 4-NIR | 0.76-0.90 | 30 | |
Band 5-SWIR 1 | 1.55-1.75 | 30 | |
Band 6-Thermal | 10.40-12.30 | 120 | |
Band 7- SWIR 2 | 2.08-2.35 | 30 |
Also, meteorological variables, including precipitation, temperature (plus variables required to calculate standard evapotranspiration), and MATLAB R2018a programming, were used to calculate the meteorological and agricultural drought indices employed in this study. In this research, the data of three synoptic stations (Rasht, Shiraz, and Birjand, one for each climate) in the statistical periods 2002 and 2014-2020 on a monthly scale due to the adequacy and completeness of the data and comparison with the remotely sensed drought indicators were used. These data were received from the country’s Meteorological Organization (https://data.irimo.ir). After sorting the data using SPSS software, outlier data were identified and discarded. A brief explanation of the studied indices is given below. The flowchart in Figure 2 shows the steps of this research.
2.2. Simple ratio (SR) vegetation index
The SR index is obtained from the ratio of the wavelength with the highest reflectance for vegetation, obtained with Near Infrared Spectroscopy (NIR), and the wavelength of the deepest chlorophyll uptake (red) helps distinguish between densely and non-densely vegetated areas (Birth and McVey, 1968; Melillos and Hadjimitsis, 2020).
2.3. Normalized Difference Vegetation Index (NDVI)
The NDVI index distinguishes between vegetated and non-vegetated areas (Manandhar et al., 2009). This index is calculated based on the reflection of red and infrared bands (Kogan, 1993; Gessesse and Melesse, 2019). The NDVI is the normalized index of vegetation differential per pixel of satellite imagery, the red is the red band in the image, and the NIR band is near-infrared. The numerical value of this index varies between -1 and +1. The numerical value of 1 indicates areas with more vegetation, while zero and values close to it are related to barren areas without vegetation. In areas with cloud cover, snow, and water, due to the more excellent reflection of sunlight within the visible range compared to near-infrared, this value tends to be less than zero (Rouse et al., 1974; Dutta et al., 2021).
2.4. Soil Adjustment Vegetation Index (SAVI)
The SAVI is a revised NDVI that aims to reduce soil moisture’s effects (Huete, 1988; Stephenson et al., 2021). The L factor is the correction of soil effects, ranging between zero and 1 for areas with dense and low vegetation, respectively. It is helpful in areas with low vegetation such as Birjand (L = 1), medium vegetation such as Shiraz (L = 0.5), and high density vegetation such as Rasht (L = 0.25) (Huete, 1988). Like the NDVI, its numerical value varies between -1 and +1 (Stephenson et al., 2021).
2.5. Standardized Precipitation Index (SPI)
The SPI was first proposed by McKee et al. (1993) to monitor drought in Colorado. The standard precipitation index is based on calculating the probability of precipitation per time window. By comparing the fit of different probability distributions across different climates of Iran (including regular, two-parameter regular log, three-parameter regular log, two-parameter gamma, Pearson type III, Pearson type III log, Gamble), Bazrafshan (2002) concluded that in time windows of 3, 6, 12, and 24 months, the gamma distribution has a better fit on the precipitation data, which is a function of the probability density of this distribution (Bazrafshan and Hijabi, 2017).
The SPI relies on two assumptions: (i) the variability of precipitation is much higher than other variables (e.g., the evaporative demand of the atmosphere), and (ii) the other variables are stationary (i.e., they have no temporal trend). The importance of variables other than precipitation is negligible in this framework, and droughts are assumed to be mainly controlled by the temporal variability of precipitation (Yang et al., 2020; Abbasian et al., 2021). To determine the severity of drought in different years, it is necessary to define thresholds for SPI. Table III outlines these thresholds.
Table III Limits and descriptions of different classes of SPI indices.
Class condition | Wet | Normal | Drought |
Mild | +1 ≤ SPI ≤ +1.5 (W1*) | -1.0 ≤ SPI ≤ +1.0 (N**) | -1.5 ≤ SPI ≤ -1.0 (D1***) |
Moderate | +1.5 ≤ SPI ≤ +2.0 (W2) | -2.0 ≤ SPI ≤ -1.5 (D2) | |
Severe | +2.0 ≤ SPI (W3) | SPI ≤ -2.0 (D3) |
*Wet; **normal; ***drought.
2.6. Standardized Precipitation Evapotranspiration Index (SPEI)
The SPEI was introduced by Vicente-Serrano et al. (2010) as a climatic drought index. Monthly rainfall and air temperature data are required to calculate this index, which is based on calculating the climatic water balance (Eq. [1]):
where P and PET are the normal rainfall and evapotranspiration, respectively, D denotes difference, and I is the month number. The Penman-Monteith-FAO method (Allen et al., 1998; Zarei and Mahmoudi, 2020) is used to calculate PET if data are not restricted. It is also possible to use the simple Thornthwaite method (Thornthwaite, 1948). Neither of these methods has a limit for SPEI. To get a complete description of this index, refer to Bazrafshan and Hijabi (2017).
If the SPEI index is within the range of positive numbers, it indicates a high (positive) balance; if it is in the range of negative numbers, it indicates a low (negative) balance. When the values of this index reach -1, the drought begins and ends with a positive drought. The classification of this index is the same as in the SPI (thresholds in Table III) (Byakatoda et al., 2016; Tirivarombo et al., 2018; Zarei et al., 2021).
2.7. Palmer Drought Severity Index (PDSI)
The PDSI was used to capture fluctuations in soil moisture storage in the United States (Palmer, 1965). This index is based on the soil water balance equation. It includes meteorological and soil factors such as rainfall, temperature, and available soil moisture. Its time scale for calculations is monthly. Therefore, to calculate this index, the first four coefficients, α, β, γ, and δ, which are the evapotranspiration, feed, runoff, and loss coefficients, respectively, according to the natural and potential values of evapotranspiration, feeding, runoff, and losses, are obtained for each month of the year (Hamarash et al., 2022). To get a complete description of this index, refer to Bazrafshan and Hijabi (2017).
According to Palmer (1965), the monthly time series of this index varies between +4 and -4 (Dehghan et al., 2020). If it is in the negative range, it indicates dry periods. In contrast, within the positive range, it indicates wet periods, with values close to zero also indicating a climatic situation with standard conditions (Hamarash et al., 2022). The complete classification of the PDI is reported in Table IV (Balbo et al., 2019).
Table IV Different classes of Palmer drought intensity indices.
Class condition | Wet | Normal | Drought |
Incipient | +1.0 ≤ PDSI ≤ +0.5 (W1) | -0.5 ≤ PDSI ≤ +0.5 (N) | -0.5 ≤ PDSI ≤ -1.0 (D1) |
Mild | +2.0 ≤ PDSI ≤ +1.0 (W2) | -1.0 ≤ PDSI ≤ -2.0 (D2) | |
Moderate | +3.0 ≤ PDSI ≤ +2.0 (W3) | -2.0 ≤ PDSI ≤ -3.0 (D3) | |
Severe | +4.0 ≤ PDSI ≤ +3.0 (W4) | -3.0 ≤ PDSI ≤ -4.0 (D4) | |
Extreme | +4.0 ≤ PDSI (W5) | PDSI ≤ -4.0 (D5) |
2.8. Statistical analysis
In this study, the correlation coefficient (r) and the RMSE were used to evaluate and compare remote sensing indices (NDVI, SAVI, and SR), as well as meteorological and agricultural drought indices (SPI, SPEI, and PDSI). The Pearson correlation coefficient is calculated to measure the relative relationship between two variables with values ranging from +1 to -1 (Moriasi et al., 2007; Zuzulova and Vido, 2018). A correlation with a value of +1 indicates a complete positive correlation. In contrast, a value of -1 shows an entirely negative correlation and a value of zero reveals the absence of relationship between the two variables. The RMSE shows the root mean deviation between the two variables (Wang et al., 2012; Elavarasan et al., 2018). Many researchers, such as Peled et al. (2010), Zuzulova and Vido (2018), and Attafi et al. (2021), have employed these indices to evaluate changes.
2.8.1. The Mann-Kendall trend test
The trend in the time series of water and meteorological parameters is established using different tests divided into parametric and non-parametric. Parametric tests have more power to detect trends than non-parametric tests. When using parametric tests, data should be independent and random plus have a normal distribution. Non-parametric tests are not sensitive to the normality of the data and can be used if the data is random (Chen et al., 2007). This test was first developed by Mann (1945) and then by Kendall (1948). A strength of this method is its suitability for time series that do not follow a specific distribution. The insignificant effectiveness of this method, based on the limited values that are observed in some time series, is another of its advantages. This test is suitable to determine the significance of linear and non-linear trends. In this test, the null hypothesis (H 0 ) and the opposite hypothesis (H 1 ) are respectively equivalent to no trend and a trend in the time series of observational data. In a two-domain test to check the trend of the data series, the null hypothesis (absence of trend) is accepted if the relationship holds (Eq. [4]):
where α is the significance level considered for the test, and Z α is the standard normal distribution statistic at the α significance level. If the Z statistic is positive, the trend of the data series is considered upward, and if it is negative, the trend is considered downward. If the absolute value of the Z statistic is greater than 1.96 at the significance level of 0.05, then the null hypothesis is rejected, and a significant trend exists (Nejadrekabi et al., 2022). This research used XLSTAT software to calculate the trend through the Mann-Kendall test.
3. Results and discussion
Drought values from the NDVI, SAVI, and SR remote sensing indices in three regions with different weather conditions during the studied years are indicated in Figure 3. As can be seen, the NDVI and SAVI indices are very close to each other. It can be stated that the corrected SAVI index is the NDVI index, which eliminates the effect of soil. Indeed, in the Rasht region, where the amount of vegetation is high, these two indices are very close to each other, while in the Shiraz and Birjand regions, where vegetation is low compared to the wet area of Rasht, the effect of soil is more significant. The distance between these two indices increases, showing that SAVI performs better for Shiraz and Birjand than NDVI.
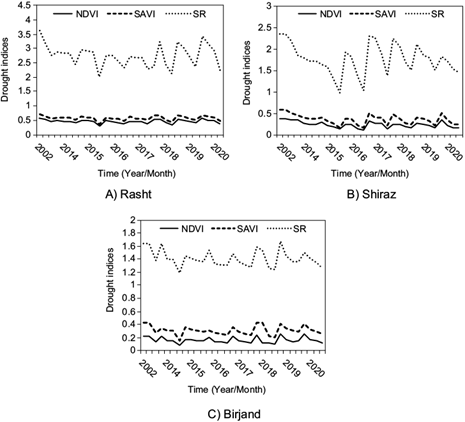
Fig. 3 Changes in remote sensing indices (NDVI, SAVI, and SR), during the studied years in (a) Rasht, (b) Shiraz, and (c) Birjand.
Researchers such as Sun et al. (2020) and Zou et al. (2020) found similar results to the findings of this study in parts of northern China and northwestern Costa Rica, respectively. Figure 4 shows images of the driest months investigated in these areas in terms of the NDVI, which in Rasht was 0.30, Shiraz 0.13, and Birjand 0.08. These changes indicate the effect of drought on vegetation in the study areas, and it can be stated that the change in climate trend towards drought or wet season has also altered the values of the vegetation index and has behaved similarly (Marumbwa et al., 2020). Darwish and Faour (2008) set the NDVI as the most common index in assessing vegetation. Also, many researchers have used this index in their studies (Rahnama et al., 2022; Asam et al., 2023; Das et al., 2023).
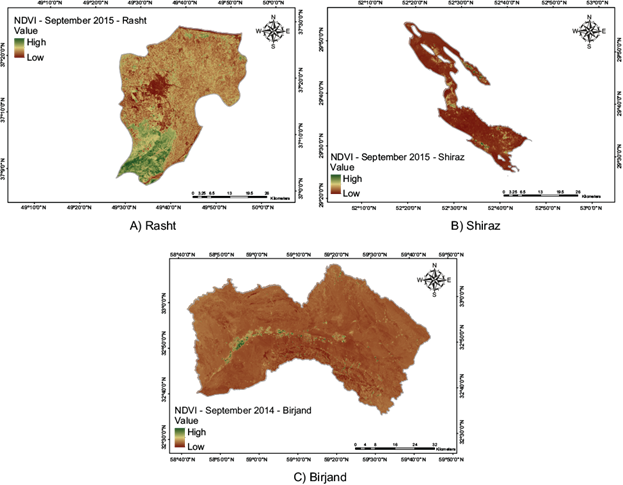
Fig. 4 Map of the average drought indices in the driest month in the studied years in different regions in terms of the NDVI. (a) Rasht, (b) Shiraz, and (c) Birjand.
Changes in the NDVI from May to September during 2002, 2014, and 2020 in Rasht indicate a downward trend and a reduction in the level of vegetation. Gradually, as the fall season approaches, the amount of this index drops. These changes have a milder slope in 2014, while the slope of changes reaches its maximum in 2020. The maximum value of this index in 2002 is related to May and the minimum to September, being 0.59 and 0.46, respectively. In 2014, the maximum and minimum values for May and September were 0.49 and 0.41, respectively. NDVI values in 2020 were 0.56 in May and 0.38 in September.
The changes in NDVI from May to September in 2002, 2014, and 2020 in Shiraz have also a downward trend. These changes have a milder slope in 2014, while the slopes in 2002 and 2020 are higher. The maximum value of this index in 2002 is related to May, and the minimum to September, being 0.43 and 0.35, respectively. In 2014, the maximum and minimum values for May and September were 0.36 and 0.25, respectively. NDVI values for 2020 were 0.35 in May and 0.16 in September.
This downward trend is also present in the values of the NDVI index for Birjand, with the slope of changes in 2020 and 2014 being sharp and 2002 being gentler than the other two periods. Changes in the NDVI index in Birjand show poorer vegetation than in Rasht and Shiraz; the maximum value of this index in all periods is about 0.2, with its minimum values in 2002, 2014, and 2020 being 0.14, 0.08, and 0.11, respectively.
The results of the SAVI index (0.37 in Rasht [September 2015], 0.16 in Shiraz [September 2015], and 0.15 in Birjand [September 2014]) indicate a more severe drought situation in these areas (Fig. 5). This study’s results are in line with the findings of Fadaei (2018) and Mirmousavi et al. (2010), who obtained good results using this index in connection with the analysis of agricultural drought.
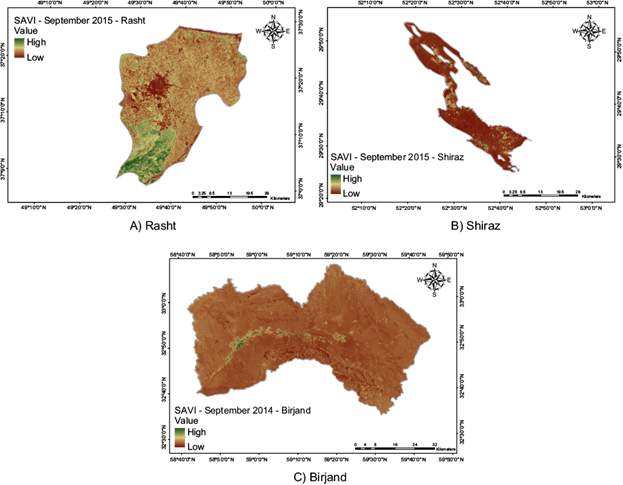
Fig. 5 Map of the average drought indices in the driest month in the studied years in different regions in terms of the SAVI. (a) Rasht, (b) Shiraz, and (c) Birjand.
The SAVI changes from May to September 2002, 2014, and 2020 in the Rasht region, similar to the NDVI index, had a downward trend and decreasing vegetation. The slope of its changes was high in 2002, while in 2014 it was gentler. The minimum values of this index in September 2002, 2014, and 2020 were 0.56, 0.51, and 0.47, respectively, and the maximum values in May were 0.80, 0.61, and 0.69.
The descending trend of the SAVI index in Shiraz was observed from May to September 2002, 2014, and 2020, indicating a decline in vegetation in the region. The change slope in 2020 was steeper than in the other two years, while 2002 and 2014 had a gentle and close slope. The highest value was in May 2002 (0.60) and the lowest in September (0.52). This index’s minimum and maximum values in 2014 were 0.38 and 0.45, respectively, being related to September and May. In 2020, the maximum value was 0.52, and the minimum was 0.24 in May and September.
There is also a falling trend in changes in the SAVI index in Birjand, but it has been lower due to the dry climate and less vegetation than in the other two regions. The maximum in 2002 was 0.49 (May), and the minimum was 0.27 (September). In 2014, the maximum and minimum values for May and September were 0.34 and 0.15, respectively. In 2020, the maximum value was related to May and the minimum to September (0.41 and 0.25, respectively). Changes in this index in 2002 had a steeper slope, while the slope in 2014 and 2020 was less pronounced.
The SR index in the Rasht region had higher values due to high vegetation compared to Shiraz and Birjand, which was also confirmed by the results of Adeyeri et al. (2017). Also, in this study the value of the SR index showed the highest drought in Rasht and Shiraz in September 2015, and Birjand in September 2014 (Fig. 6). Thus, it can be stated that the area’s vegetation density has diminished over time. These results align with Yaghoobzadeh et al. (2009) and Barati et al. (2011).
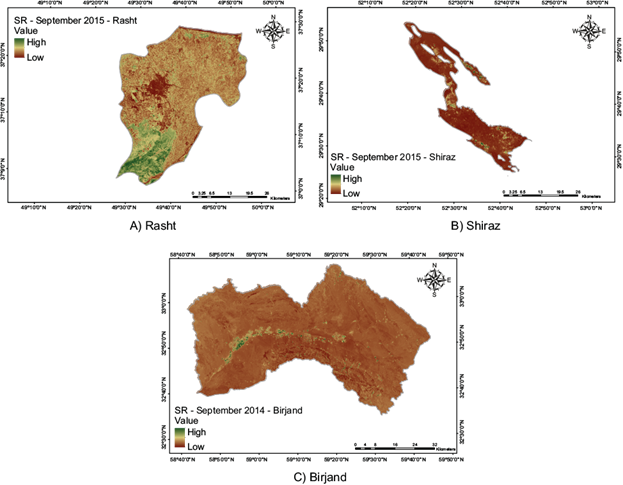
Fig. 6 Map of the average drought indices in the driest month in the studied years in different regions in terms of the SR index. (a) Rasht, (b) Shiraz, and (c) Birjand.
The declining trend in the SR index changes can be seen in the other two indices (NDVI and SAVI). So, the slope of changes in 2002 and 2020 was steep, while in 2014, it was gentler at Rasht. The maximum values of this index in 2002, 2014, and 2020 were 3.82, 2.89, and 3.41 in May, respectively, suggesting the density of vegetation. This index’s minimum values during these three September periods were 2.74, 2.44, and 2.22, respectively.
In Shiraz, the maximum slope was observed in 2002 and the minimum in 2020. The highest value of this index was 1.82 in May 2020, and the lowest was 1.46 in September. In 2002, the maximum value was 2.45 in May, and the minimum was 2.19 in September.
In the Birjand region, the slope of SR changes in the three years analyzed was relatively gentle, approximately equal to 0.1. The minimum values during these periods (2002, 2014, and 2020) in September were 1.38, 1.19, and 1.27, respectively, while the maximum rate varied from 1.78 in May 2002 to 1.51 in 2020. Given that Rasht is a humid region with rich vegetation, it was expected that the values of NDVI, SAVI, and SR would be maximum, which is evident in the graphs and results. On the other hand, the Shiraz region, with a semi-arid climate, has less vegetation, and the index values were about 0.4. In contrast, Birjand, a dry area, has similar values to Shiraz, with an index of about 0.3. In the semi-arid and arid regions of Shiraz and Birjand, respectively, a more significant effect of rainfall on vegetation variables was observed (Hamzeh et al., 2017; Heydari et al., 2018).
Also, the results of the survey maps of the studied indices revealed that May 2002 was the wettest month in all three regions. These results coincide with the studies of Wilson et al. (2016) and Fadaei (2018). Using these indices provides a better understanding of the drought situation in an area.
The results also indicate that the value of these indices was high in all years under study in Rasht, but over time, the drought increased, and the amount of vegetation decreased in this region. In Shiraz, a significant decline in the average value of the indices in August and September 2015 and 2020 concurred with drought. This reduction was also seen in the average values of the indices in Birjand from September 2002 to 2020. The study of Zarei et al. (2013) in different regions of Iran also indicated an increase in vegetation density in 2002 and a decline during the study period. Note that soil moisture, temperature, wind, cloud cover, and temporal rainfall distribution affect drought (Zarei et al., 2013).
Figure 7 shows the average temperature, evapotranspiration, and annual precipitation in the studied stations during a 30-year period (1991-2020). It shows an increasing trend for temperature and a decreasing trend for precipitation at the studied stations, which confirms the effects of global warming and climate change. The results of Mansouri et al. (2019), Vaghefi et al. (2019), and Banihashemi et al. (2021) in different climates of Iran also confirm these changes. The results of meteorological and agricultural drought indices, including SPI, SPEI, and PDSI, in three regions with different weather conditions, are presented in Figure 8. According to the SPI values, Rasht suffered a severe drought (-2.82) in September 2018, Shiraz suffered a moderate drought (-1.59) from May to August 2018, and Birjand experienced a moderate drought (-1.17) in July 2018. Since the amount of precipitation is the only effective parameter in estimating this index, based on precipitation charts (Fig. 7), a light wet season, a regular wet season, and a light wet season were estimated in Rasht, Shiraz, and Birjand, respectively.
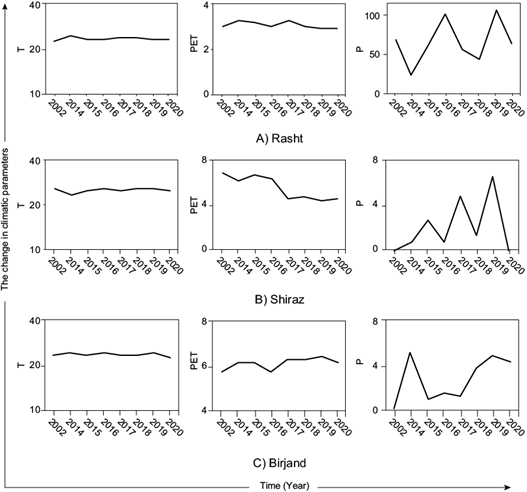
Fig. 7 Changes in temperature (T), evapotranspiration (PET), and precipitation (P) during the studied years in (a) Rasht, (b) Shiraz, and (c) Birjand.
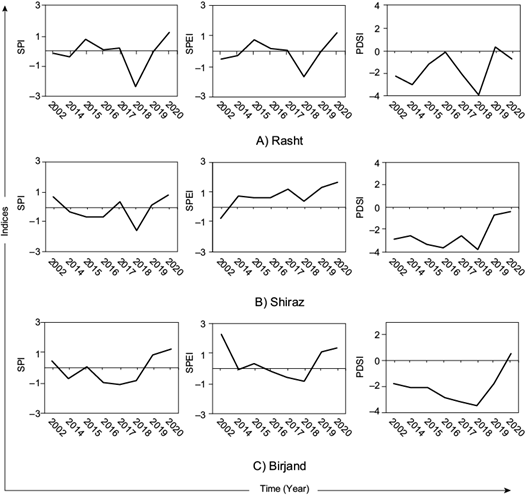
Fig. 8 Changes in drought values of meteorological and agricultural indices (SPI, SPEI, and PDSI) during the studied years in (a) Rasht, (b) Shiraz, and (c) Birjand.
SPI values in Rasht have a descending trend, so the maximum slope was observed in 2014 while a mild slope was detected in 2002. During these three periods, including May and August 2020, the maximum SPI value was 1.48; in May 2014, the minimum value was -0.08. The SPI variation in Shiraz also has a decreasing trend, which simultaneously decreases the amount of rainfall. According to the SPI, the situation is typical of this region in all three years under study. The variations of SPI in Birjand between 2002 and 2014 have been decremental and, in 2020, incremental. So, in 2002 and 2014, the values of SPI were about 0.13 and -0.53, respectively, which are under average conditions. Furthermore, 2020 has been a mild, wet season with a value of about 1.16. Ma’rufah et al. (2017) indicated that although the duration of meteorological drought varies in each region, it generally occurs from June to November.
According to the SPEI, the drought in Rasht, Shiraz, and Birjand occurred in September 2018 (-1.80, moderate drought), August 2005 (-0.90, mild drought), and May 2019 (-0.89, mild drought), respectively. The SPEI changes in Rasht have a decreasing trend. The trend of changes in 2002 is more than in 2020. The values of SPEI in 2002 during May, July, August, and September were -0.09, 0.05, -0.82, and -1.18, respectively, indicating a mild drought situation in September and a normal state in other months. In 2014, these values ranged from 0.04 in May to -0.67 in September, indicating a normal situation. Also, in 2020, May had a moderate wet season with a value of 1.51; July and August had a mild wet season with values of 1.03 and 1.47; and September had a typical wet season with a value of 0.77. In Shiraz, the SPEI changes had a decreasing trend and almost the same slope every three years. The SPEI values in 2002 varied from -0.66 in May to -0.87 in September, as presented in Table III. In the four months studied in 2014, the SPEI values of about 0.70 were typical in terms of drought. Also, in 2020, with the increase of this index to about 1.60, it depicted a moderate wet season. In Birjand, the changes in the SPEI between 2002 and 2020 were upward, while they were downward in 2014. With average values of 1.34 and 0.31, respectively, 2020 and 2014 show a typical situation during these months. A severe wet season occurred in 2002, with a maximum of 2.40 in July. These results are consistent with those of researchers such as Jiang et al. (2017) and Behrang et al. (2019).
According to PDSI values, September 2018 in Rasht region (PDSI = -3.33), May 2018 in Shiraz (PDSI = -3.35), and May 2018 in Birjand (PDSI = -4.27) were affected by severe drought, severe drought, and very severe drought, respectively. It can also be stated that the Rasht region experienced the most severe drought in terms of SPI, SPEI, and PDSI in September 2018.
Researchers such as Unal et al. (2018) and Zhao et al. (2018) achieved similar results in their studies. Also, the values follow those of precipitation during these years; with decreasing rainfall, the value of these indices has increased and vice versa (Fig. 7). These indices show a direct relationship with the values of evapotranspiration and temperature (Fig. 7). Jiang et al. (2015) also found that due to temperature elevation according to PDSI, SPI, and SPEI criteria, the drought severity could grow with decreasing rainfall and more water demand.
The changes in PDSI in Rasht in 2002 and 2014 had a descending trend and an upward trend in 2020, when the lowest value of -1.37 (mild drought) was obtained in July and the highest value of 0.80 (initial and very mild wet season) in August. In 2014, the minimum values were obtained in September (-3.12) and the maximum in May (-1.38), which represents severe and mild drought, respectively. In 2002, the value of this index varied from -1.85 in May to -1.90 in September, which indicates a mild drought situation during this period. The changes in PDSI in Shiraz during 2002 and 2014-2020 have an upward trend; in these years the slope of changes was about 0.2. The values of this index in May and July of 2002 (about -2.22) had a moderate drought status, and August and September had a mild drought status with values of -1.96 and -1.76, respectively. In May 2014, the value was -2.34, indicating moderate drought. July, August, and September also had mild drought conditions with about -1.82. The results of this index in July, August, and September 2020 are similar, with a slope of changes of about -0.06%, showing the typical situation of these months in terms of drought. The slope of changes in Birjand between 2002 and 2014 is positive and upward, and in 2020 it is downward. Note that 2002, with a value of -2.16, had moderate drought, and other months had a mild drought. May and August 2020 had an early and light wet season, respectively, while the other months were typical. Sun and Liu. (2014) and Mu et al. (2018) found that the PDSI index could be helpful to identify agricultural drought in June, July, August, and September.
Given the global temperature increase during the last 150 years and that climate change models predict a marked increase during the 21st century (Abbass et al., 2022), it can be expected that the rise in temperature will have dramatic consequences in drought conditions. Therefore, using drought indices that include temperature data, such as the PDSI, seems to be better than relying on indices without temperature information to identify warming-related drought impacts on different ecological, hydrological, and agricultural systems. However, the PDSI lacks the multiscalar character of the SPI, essential for assessing drought in relation to different hydrological systems and also for differentiating among different drought types.
The relationship between remote sensing indices and meteorological plus agricultural drought indices in all three regions was determined during the study period. The values of the correlation coefficient are presented in Table V. As it can be seen, there is a relatively strong relationship between drought indices. Compared to remote sensing drought indices in Rasht, the highest correlation was observed between NDVI and SR (R = 0.98), in the Shiraz region between NDVI and SAVI with a correlation coefficient of 0.98, and in Birjand between SAVI and SR (R = 0.98). Regarding the comparison of meteorological and agricultural drought indices against drought indices based on remote sensing data, it can be stated that the highest correlation was observed in the Rasht region between NDVI plus SAVI and SPI (R = 0.69), in the Shiraz region between SR index and SPEI with a correlation coefficient of 0.84, and between NDVI and SPEI in the Birjand region with a correlation coefficient of 0.95. It can be stated that the more significant impact of precipitation on vegetation, compared to other variables, is clearly visible in the arid and semi-arid regions of Birjand and Shiraz (Hamzeh et al., 2017). Shahabfar et al. (2012) monitored drought in Iran using vertical drought indices and the statistical comparison results showed that the correlation value between the indices is different in several climatic regions.
Table V Correlation coefficients between meteorological and agricultural drought indices and drought indices based on remote sensing data.
Rasht | ||||||
NDVI | SAVI | SR | SPI | SPEI | PDSI | |
PDSI | 1.00 | |||||
SPEI | 1.00 | 0.50 | ||||
SPI | 1.00 | 0.68 | 0.74 | |||
SR | 1.00 | 0.66 | 0.59 | 0.53 | ||
SAVI | 1.00 | 0.92 | 0.69 | 0.63 | 0.51 | |
NDVI | 1.00 | 0.93 | 0.98 | 0.69 | 0.62 | 0.50 |
Shiraz | ||||||
NDVI | SAVI | SR | SPI | SPEI | PDSI | |
PDSI | 1.00 | |||||
SPEI | 1.00 | 0.66 | ||||
SPI | 1.00 | 0.83 | 0.61 | |||
SR | 1.00 | 0.73 | 0.84 | 0.48 | ||
SAVI | 1.00 | 0.95 | 0.67 | 0.82 | 0.48 | |
NDVI | 1.00 | 0.98 | 0.91 | 0.63 | 0.77 | 0.40 |
Birjand | ||||||
NDVI | SAVI | SR | SPI | SPEI | PDSI | |
PDSI | 1.00 | |||||
SPEI | 1.00 | 0.76 | ||||
SPI | 1.00 | 0.74 | 0.61 | |||
SR | 1.00 | 0.36 | 0.53 | 0.52 | ||
SAVI | 1.00 | 0.98 | 0.42 | 0.57 | 0.51 | |
NDVI | 1.00 | 0.63 | 0.59 | 0.84 | 0.95 | 0.67 |
Finally, the RMSE evaluation criterion was used to compare the results, which are presented in Table VI. Based on these results, when comparing the remote sensing drought indices in all three regions, the lowest error rate was estimated between NDVI and SAVI (0.20, 0.125, and 0.162 for Rasht, Shiraz, and Birjand, respectively). Also, when comparing meteorological and agricultural drought indices with remote sensing drought indices, the lowest error rate in the Rasht region was reported between NDVI and SPEI (RMSE = 0.608), in the Shiraz region between NDVI and SPI (RMSE = 0.718), and in the Birjand region between SAVI and SPEI (RMSE = 0.463). Zuzulova and Vido (2018) also showed a strong correlation between drought indices from January to September in Slovakia, and Luo et al. (2020) reported a positive correlation between NDVI and SPEI drought indices in parts of northern China with dry climates. Similar results were reported by Peled et al. (2010), Choi et al. (2013), and Shen et al. (2019) in northern China, as well as Wang et al. (2020) and Ghosh et al. (2021) in parts of Europe.
Table VI RMSE values between meteorological and agricultural drought indices with drought indices based on remote sensing data.
Rasht | ||||||
NDVI | SAVI | SR | SPI | SPEI | PDSI | |
PDSI | 1.00 | |||||
SPEI | 1.00 | 1.744 | ||||
SPI | 1.00 | 0.760 | 1.341 | |||
SR | 1.00 | 2.942 | 2.564 | 4.043 | ||
SAVI | 1.00 | 2.237 | 1.091 | 0.643 | 2.056 | |
NDVI | 1.00 | 0.120 | 2.355 | 1.039 | 0.608 | 1.967 |
Shiraz | ||||||
NDVI | SAVI | SR | SPI | SPEI | PDSI | |
PDSI | 1.00 | |||||
SPEI | 1.00 | 2.805 | ||||
SPI | 1.00 | 0.958 | 2.003 | |||
SR | 1.00 | 2.020 | 1.412 | 3.939 | ||
SAVI | 1.00 | 1.430 | 0.780 | 0.910 | 2.540 | |
NDVI | 1.00 | 0.125 | 1.553 | 0.718 | 0.972 | 2.427 |
Birjand | ||||||
NDVI | SAVI | SR | SPI | SPEI | PDSI | |
PDSI | 1.00 | |||||
SPEI | 1.00 | 2.981 | ||||
SPI | 1.00 | 1.156 | 1.948 | |||
SR | 1.00 | 1.884 | 0.773 | 3.713 | ||
SAVI | 1.00 | 1.079 | 0.915 | 0.463 | 2.647 | |
NDVI | 1.00 | 0.162 | 1.234 | 0.796 | 0.589 | 2.494 |
Table VII shows the trends of drought index values using the Mann-Kendall method. Generally, based on the values of the introduced statistics, if the Z value is greater than 1.96 at the 95% confidence level, the trend is significant and vice versa. Based on this, the increasing trend of drought is confirmed in the three studied regions based on the investigated indicators (SPI, SPEI, and PDSI). This means that the drought is increasing during the statistical period under study, also considering that in all the studied areas the value of the Z statistic is lower than 1.96, no significant trend has been observed at the 95% confidence level. Nagy et al. (2020) found no significant trend in all the indicators (SPI and SPEI) when evaluating stations in Slovakia.
Table VII Analysis of drought trends in the study areas using the Mann-Kendall test at the 95% confidence level.
Z statistics of Mann-Kendall test | ||||||
Rasht | ||||||
NDVI | SAVI | SR | SPI | SPEI | PDSI | |
May | -0.210 | -0.182 | -0.254 | 0.034 | 0.00 | 0.138 |
July | -0.161 | -0.143 | -0.198 | 0.094 | 0.062 | 0.136 |
August | -0.138 | -0.121 | -0.159 | 0.148 | 0.118 | 0.191 |
September | -0.184 | -0.168 | -0.204 | 0.181 | 0.153 | 0.190 |
Shiraz | ||||||
NDVI | SAVI | SR | SPI | SPEI | PDSI | |
May | -0.330 | -0.291 | -0.202 | 0.178 | 0.330 | 0.192 |
July | -0.329 | -0.292 | -0.200 | 0.099 | 0.370 | 0.249 |
August | -0.346 | -0.312 | -0.222 | 0.302 | 0.402 | 0.282 |
September | -0.363 | -0.331 | -0.250 | 0.020 | 0.431 | 0.319 |
Birjand | ||||||
NDVI | SAVI | SR | SPI | SPEI | PDSI | |
May | -0.202 | -0.202 | -0.242 | 0.109 | 0.335 | 0.904 |
July | -0.173 | -0.177 | -0.219 | 0.035 | 0.264 | 0.025 |
August | -0.179 | -0.192 | -0.235 | 0.032 | 0.200 | 0.041 |
September | -0.218 | -0.214 | -0.271 | 0.091 | 0.141 | 0.089 |
Also, since the value of the Z statistic was negative during the study period (May, July, August, and September) in relation to remote sensing indicators such as NDVI, SAVI, and SR, there is a downward trend (reduction of vegetation and increase of drought). In addition, no significant trend was observed in any of these indices at the 95% level. The results of Maroufzade and Attarod (2021) in the northern Zagros region of Iran and He et al. (2022) in parts of China confirm this.
Drought is a process that aggravates the erosion of natural resources and puts heavy pressure on the government to take measures for dealing with this phenomenon. It causes crop reduction, poverty, food shortage, endangering of human and animal health, scarcity of drinking water, degradation of underground water quality, and a decrease in water tables. The evaluation of droughts (especially vegetation droughts) in terms of time and place is one of the most important aspects of agricultural planning and management. In fact, spatial and temporal assessments of vegetation drought are vital for planning appropriate operations to improve agricultural conditions in drought situations. This is more important in areas where a large population depends on agriculture (IPCC, 2007). The use of advanced techniques such as remote sensing and drought indicators can significantly help in discovering and preparing a drought-oriented map of vegetation. Temporal and spatial evaluations are very useful in the decision-making process for monitoring drought and determining operations to reduce its effects. These accurate evaluations depend on new technologies for drought policymakers to prioritize operations. Also, it is vital for experts and researchers to produce technologies and information, including the production of resistant species, as well as crop management and agricultural soil moisture protection operations. The results of this study suggest that the use of remote sensing techniques combined with drought indices can be considered a suitable tool for optimal management of water resources, land use planning, and reduction of costs due to drought.
4. Conclusions
The use of drought indicators has been proposed as a suitable tool for management measures and subsequently for dealing with the drought phenomenon worldwide. Thus, in this study, drought was studied in three different climates of Iran (Rasht, Shiraz, and Birjand) using meteorological, agricultural, and drought indices of remote sensing for 2002 and 2014-2020. The results of different indicators were compared through the correlations between them. The findings can be summarized as follows:
According to the remote sensing drought indices, the highest drought intensity for the Rasht and Shiraz regions occurred in September 2015, with the Birjand region showing the highest drought intensity in 2014. The results also revealed an increase in drought in three different climates; thus, 2002 was selected as the wettest year during the study period. In the Rasht region, where the amount of vegetation is high, NDVI and SAVI were close to each other. On the other hand, in Shiraz and Birjand, where vegetation areas have reduced and the effect of soil is more significant, the distance between these two indices increased, showing better results for the SAVI index in Shiraz and Birjand compared to the NDVI index. Results also show that the severity of drought could grow with decreasing rainfall and more water demand, due to temperature elevation according to SPI, SPEI, and PDSI criteria. Regarding the correlation coefficient, there is a relatively strong relationship between drought indices. In fact, by comparing meteorological and agricultural drought indices against drought indices based on remote sensing data, it can be stated that the highest correlation in the Rasht region was found between NDVI plus SAVI indices and SPI index (R = 0.69), in the Shiraz region between SR and SPEI indices with a correlation coefficient of 0.84, and between NDVI and SPEI indices in Birjand with a correlation coefficient of 0.95. Based on the results of the Mann-Kendall test, the increasing trend of drought in the three studied regions is confirmed based on the investigated indicators (SPI, SPEI, and PDSI). It means that drought is increasing during the statistical period under study. Also, in relation to remote sensing indices such as NDVI, SAVI, and SR there is a downward trend (vegetation reduction and increase) due to the negative value of the Z statistic.
In addition to reducing the available surface and underground resources and causing great damage to the irrigated and rainfed agricultural sector, the occurrence of drought also affects different parts of the pasture ecosystem. If the destruction of natural resources continues as up to date, the depletion of vegetation can reduce the flexibility of the environment against the occurrence of drought and this will trigger a self-intensification process. Drought results from the influence of different environmental parameters in a specific area. Knowledge and awareness of the relationships and mutual effects of these environmental parameters and determining the relative sensitivity of specific areas to drought is considered vital in order to take the necessary measures to reduce the effects of drought and avoid the occurrence of disasters on human life, plants, and animals.