1. Introduction
Development banks should be able to contribute to the best performance of the financial system in which they operate in order to compensate for the effects of the inherent problems of the capitalist system itself. Considering the role of development banks, this paper aims to measure the impact of the presence of the branches of a development bank, the State bank of Pará (Banpará), in the municipalities.
The financial centers are known to be responsible for the best innovative aspects of the sector. The peripheries should adapt to those aspects, resulting in a higher concentration of banks in the central regions (more developed). This phenomenon is perceptible because banks are dynamic and innovative capitalist companies with lucrative purposes that aim to generate wealth and value by adjusting assets and liabilities. They align their business portfolio to make a profit advantage (Amado, 1997).
Contrary to commercial banks, the central objective of Banpará is to add value to the State of Pará, working on the economic and social development of the population. Since 2010, the bank has expanded the installation of branches in the municipalities of the State of Pará located in peripheral regions in order to contribute to local financial development. The expansion of branches in such areas opposes the movements led by private institutions. However, it can contribute as a booster of economic growth to broaden access to the financial system in the least developed regions. In this context, Banpará presents a policy contrary to the market, with bank branches in virtually all the municipalities of the State of Pará. This raises questions about the socio-economic importance of such actions for regions.
On this basis, estimating the impact of the presence of development banks in local economies is to assess the effectiveness of public economic policies promoted by regional financial planning. However, calculating causal effects for evaluating policies is challenging for social sciences. Moreover, the impossibility of randomized experiments repeatedly precludes the impact measurement of the actions on specific populations with bias. In this sense, specific econometric techniques must be used to consider such limitations in order to accurately evaluate the policy’s effect.
This study utilizes spatial and impact assessment methodologies to evaluate the financial outcomes resulting from the presence of Banpará branches in the municipalities of the State of Pará, Brazil. The second section of this research explores the concepts related to economic development and the role of banks, particularly development banks. Subsequently, the analysis focuses on branches in less developed regions often exclusively served by Banpará. The methodologies employed in this study are then described. The results section presents spatial analyses to examine the correlation between the presence of branches and the socioeconomic variables under investigation.
Additionally, the impact of branch presence on 2019 production variables is evaluated using matching techniques. The choice of 2019 data is due to the disruptions caused by the 2020 pandemic, which affected production variables and made estimations challenging. Furthermore, the results obtained through the Difference-in-Differences (DD) methodology provide insights into the temporal effects. Finally, in the concluding section, a summary is provided regarding the significance of development banks based on the findings from this study, specifically focusing on the State of Pará.
2. Banks and Economic Development
The finance resource represents the independence of the decision to invest concerning the flow of income saved due to the existence of credit. Corder and Salles-Filho (2006) observed that firms could always resort to short-term sources but with high operating costs. Therefore, firms need to utilize sources that supply funds with costs and terms compatible with the terms of the expected return. Thus, an alternative would be to meet financial needs based on the ability to generate funding, which could be made possible through trading shares in the capital market or the sale of debt securities (Arestis et al., 2017).
The relationship between finance and funding may need to be organized and triggered since financial systems are divided into basic systems in which the capital market and credit-based systems predominate. The relationship between them tends to be stimulated by economies with an organized financial system and a structured capital market as a way of stimulating investment (Studart, 1999).
Considering public banks, such as Banpará, Marshall and Rochon (2019) argue that this type of bank can be used as a countercyclical instrument in the case of an economy in recession but that they can also be used as instruments of economic development, given that credit can be directed in a way that boosts infrastructure, consumer credit, and others.
Development banks play a crucial role in financing investments and fostering economic development. They can address the financial constraints firms face, especially in the context of innovation and infrastructure projects. By providing credit and funding, development banks enable businesses to overcome the difficulty of mobilizing capital and promote the growth and development process. Overall, development banks are vital in providing financial resources, promoting investment, and driving economic development. It highlights their ability to address market failures, to support long-term projects, and to act as catalysts for sustainable growth. These insights are a strong rationale for policymakers and stakeholders to prioritize establishing and strengthening development banks as essential institutions in fostering socioeconomic progress in underdeveloped regions.
The presence of Banpará in the municipalities
In 2019, Banpará branches had a higher coverage in the municipalities of the State of Pará when compared with private bank branches. The state bank was present in 107 municipalities, representing 74% of the municipalities of the State of Pará, whereas private branches were located in 86 municipalities (see Figures 1 and 2).
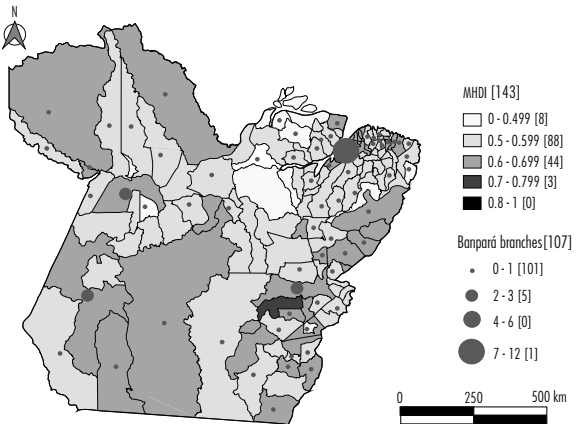
Note: QGIS software (Data from IPEA and BACEN).
Source: own elaboration.
Figure 1 Banpará branches and MHDI
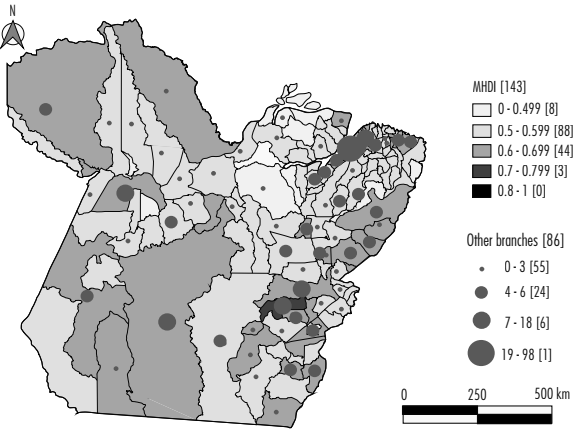
Note: QGIS software (Data from IPEA and BACEN).
Source: compiled by the authors.
Figure 2 Private branches and MHDI
Considering the Municipal Human Development Index (MHDI), the distribution of Banpará branches presented in Figure 1 portrays more homogeneous coverage in municipalities with lower economic development indexes, different from the proved distribution of branches (see Figure 2). On the other hand, the movement of the concentration of private branches in more developed regions, such as the metropolitan region of Belém and the Southeastern region of the State, is evident, especially around the municipalities of Marabá, Parauapebas, and Canaã dos Carajás. These are essential commercial poles in the export of iron ore.
In Pará, most state municipalities, corresponding to 61%, have low MHDI (0.5-0.599), and 30% have medium MHDI (0.6-0.699). Only three municipalities, representing 2.1%, have high MHDI (0.70.799): Belém, Ananindeua, and Parauapebas. Eight municipalities, 5.6%, still have a very low MHDI (0-0.499), which are Melgaço, Chaves, Bagre, Cachoeira do Piriá, Portel, Anajás, Afuá, and Ipixuna do Pará. It should be noted that Melgaço (PA) is also the municipality with the lowest MHDI in Brazil. Among the lowest MHDI municipalities, only Portel, Bagre, and Chaves do not have Banpará branches. The other municipalities have at least one Banpará branch, and only Portel has a private institution (see Table 1). Therefore, more branches are located in the most deprived municipalities.
Table 1 Municipalities in Pará with significantly lower MHDI and bank branches
Municipalities | HDI very low (2010) | Banpará branches (2019) | Other banks branches (2019) |
---|---|---|---|
Melgaço | 0.418 | 1 | 0 |
Chaves | 0.453 | 0 | 0 |
Bagre | 0.471 | 0 | 0 |
Cachoeira do Piriá | 0.473 | 1 | 0 |
Portel | 0.483 | 0 | 2 |
Anajás | 0.484 | 1 | 0 |
Afuá | 0.489 | 1 | 1 |
lpixuna do Pará | 0.489 | 1 | 2 |
Source: compiled by the authors (Data from IPEA and BACEN).
Additionally, of the 101 municipalities with at least one Banpará branch, 35 are exclusively served by the bank. It is therefore clear that the presence of the bank does not seek direct or immediate benefit from the market conditions in which it has been established since 2010.
According to Marques (2019), considering the economic formation of Brazil and the economic-political project implemented in the Amazon region, the condition of general inequality in the country and the current underdevelopment manifested in the State of Pará is a direct consequence of the institutional position taken by the Brazilian federal government during the 20th century. Currently, in the State of Pará, the regional inequalities are demonstrated by observing the economic and social performance presented by the data made available by city halls.
The following sections present the methodologies, databases, and results achieved using the econometric estimation in this paper.
3. Methodologies
The methodology for the impact assessment consists of three steps; the first is a spatial analysis to verify the correlation between the location and the analyzed variables and the presence of branches, the spillover effect. With this information, the impact is calculated based on the methodologies for matching, average treatment effect, and DD.
The spillover effect
The objective is to estimate the spatial correlation referring to the results of Banpará’s policies, checking the existence of a geographic pattern in the formation of the working cluster of the bank and a spillover effect in the territory of the State of Pará. In this sense, when there is spatial independence between the observations, i.e., if there is no relation between the behavior of a variable associated with the same behavior of a close location (neighbor), then there will be spatial dependency or autocorrelation. For example, spatial dependence makes the estimation by Ordinary Least Squares (OLS) inappropriate because the measure would present bias, inconsitency or inefectiveness. Therefore, the models of spatial association allow us to visualize patterns and describe regions and potential spillover in areas through specific estimations for this type of situation.
The spillover effect is characterized by the spatial dependence of a given variable in relation to its neighbors. In other words, if the variables present the spillover effect, they may be affected by their location, and it is necessary to apply methodologies that consider spatial dependence.
Impact assessment
Calculating the causal effect for the evaluation of policies is a challenge for social sciences. Recurrently, the impossibility of randomized experiments precludes measuring the impact of actions on specific populations without bias. In this sense, it is necessary to use specific econometric techniques that in order to consider those limitations to accurately assess the effects of policies.
The calculation of the Average Treatment Effect (ATE) proposed by Cameron and Trivedi (2005) analyzes the measurement problems when evaluating the impact of a particular (treatment) policy on a specific group:
Considering variable D indicates whether there is treatment or the presence of a specific policy (when D = 1, there is the presence of treatment, and D = 0 is its absence, group control). Thus, the ATE calculation shows that under specific characteristics x, the outcome of interest is highlighted by the difference in the results of y, when treatment occurs y1, and when treatment does not occur y0.
Whereas the average treatment effect on the treated (ATET) is defined by:
However, the estimate of the second term of the equation is not observable since it is impossible to verify the results at y0i given the presence of the treatment. Thus, when rewriting the equation, one must consider a bias defined by the second term of the equation below:
In case of any randomness in the policy distribution, we consider that the group treated is equivalent to the control group. In this context,
Suppose there is no randomness in the treatment assignment process to avoid bias in estimating the effect of the policies. In that case, the municipalities of the two groups can be paired in terms of their observable characteristics. In order for the control group to be valid, the conditional independence hypothesis must be respected. Such a hypothesis provides that the treatment assignment is unconditional on the potential outcome for a given set of characteristics X.
Thus, once the treatment assignment for the municipalities with a set of similar features through X is controlled, we can perform the matching, considering the control group and treatment with similar features to a random sample (Rosenbaum and Rubin, 1983).
In addition, it is necessary to validate the SUTVA hypothesis, which requires that the treatment received by a municipality that received the policy does not affect others that did not receive it (untreated). Therefore, this condition is treated according to the previous methodology, starting with identifying the spillover effect and forming clusters.
The matching procedure performed is the propensity score estimation, the conditional probability p(X) of a municipality to be treated based on a set of observable characteristics.
The function p(X) is the propensity score or the probability of treatment T = 1 (Wooldridge, 2010). This set of variables x should not have been affected by the treatment and, therefore, ideally, should have been collected pre-treatment. Thus, the choice was made to use characteristics before the outcomes. Therefore, it is possible to evaluate the municipalities based on the same initial characteristics.
As for the estimation of the probability p(X), we use the logit binary model.
In which Yi is a binary value considering the presence or absence of treatment, and xi represents the observable characteristics that will affect Yi, the probability considered is D = 1.
The literature demonstrates techniques to perform the matching through propensity score. Such techniques aim to minimize selection bias and avoid inadequate matching. Cameron and Trivedi (2005), jointly with Caliendo and Kopeinig (2008), describe four techniques often employed. The first is the Nearest-neighbor (NN), which is about choosing an individual from the comparison group as a matching partner for a treated individual who is closest in terms of the propensity score. That choice can be made “with replacement” or “without replacement”. Using this technique, for each participant i in each municipality, a non-participant j is chosen, whereas the choice with replacement reduces the number of non-participants used to build the counterfactual outcome, thus increasing the estimator’s variance.
Another technique is Caliper and Radius Matching-CRM, an extension of NN. Every participating municipality i and non-participating j with an estimated propensity score within the Caliper is chosen. Stratification and interval matching can also be used, which proposes to divide the propensity score into a set of intervals (strata) and calculate the impact in each interval by taking the average difference in outcomes between treated and control observations. Finally, all treatment units are paired with a weighted average of all control units, according to weights (inversely proportional) related to the distance between the propensity score values of the treated and untreated.
In this way, estimating the average treatment effect after matching according to the selected technique is possible. It is also possible to use the statistics with weights, which are then given by the inverse of the probability estimated in the propensity score if the municipality belongs to a treatment group and by the complement of such a probability if the municipality belongs to the control.
The results show the estimated ATE values from the matched sample using the NN method per caliper (0.2) without replacement.
In addition to the matching model for the identification of the ATE, the DD method was used to compare changes in outcomes over time between municipalities participating in the program (treated) and those not participating (control). The model considers the variations between the interest variables in different periods, specifically 2010 and 2019.
The results consider the estimated observations per OLS (pooling), and the fixed and random effects methodology is used for more robust results. The method used was proposed by Heckman et al. (1998) and identified the impact estimator of a particular program in those who participated (ATT) in a non-parametric form. The DD consider the following equation:
While tratit is a treatment dummy, censoit is a dummy that indicates the treatment period (2010 or 2019), and DDit is the variable that indicates the tratit * timeit interaction. The variable uit represents the error term of the model. Regarding the estimators, α is constant, representing the mean of the estimated effect for the control group in the first period, where γ1 + α represents the mean of the control group for the following period, γ2 is the result of the treatment difference between the control group and the treated group, and finally, the estimated γ3 represents the DD effect or the treatment effect considering the difference between the two groups in the two time periods.
The results consider the estimated observation by OLS, and the methodology of fixed and random effects was used for the more robust results. The propensity score method was also associated with DD in the OLS estimation and fixed effects. They consider that it is the most powerful and reliable method to verify the impact of the treatment on the analyzed population since it enables weighting the variables to avoid bias arising from specification errors potentially present in a conventional regression of fixed effects. Such a procedure, named Double Robust Estimator (Bang and Robins, 2005), estimates a regression in which variables are weighted by the treatment reception probabilities, previously calculated through the propensity score. According to Imbens and Wooldridge (2009), using the estimated probability to weight the variables in the estimation of fixed effects improves the robustness of its results, eliminating the bias of omitted variables.
Data
As for the impact assessment of Banpará branches’ presence in the State municipalities, the variables of economic performances described below in Table 2 were used with data obtained from the years 2010 and 2019 (average salary). In addition, this paper will verify the impact of such branches in that period, considering the Bank’s pre-policy expansion data in 2010. The data was obtained from the Institute for Applied Economic Research (Santos et al., 2020), the Brazilian Institute for Geography and Statistics (IBGE, 2021), and the Annual Social Information Report (MTE, 2021).
Among this set, the selected variables that would constitute the preliminary analysis of the bank’s impact were: GDP, ISS, and Average salary. This data includes a consistent parameter for evaluating this impact since it result from labor productivity, public revenue, and household wealth. In the next section, the results will be presented.
Table 2 Economic performance variables used in the estimation (2010 and 2019)
Variable | Description | Source |
---|---|---|
Average income | Monthly income of families in the municipalities (BRL$). | IBGE |
Average salary | Formal remuneration of the municipalities (BRL$). | MTE |
ISS | Municipal tax for performing services provided by companies or self-employed professionals (BRL$). | IPEA |
GDP | The sum of the value of goods and services produced in the municipality in the year at current prices (BRL$). | IBGE |
Population | Number of registered inhabitants of municipalities. | IBGE |
GDP per capita | The sum of the value of goods and services produced in the municipality in the year per inhabitant at current prices (BRL$). | IBGE |
GVA (public service) | The sum of the value of services provided by the government in the year at current prices (BRL$). | IBGE |
GVA (agriculture) | The sum of the value of production in the agricultural sector in the year at current prices (BRL$). | IBGE |
GVA (industry) | The sum of production value in the industrial sector in the year at current prices (BRL$). | IBGE |
GVA (services) | The sum of production value in the services sector in the year at current prices (BRL$). | IBGE |
Employment (agricultural) | Number of formal employment relationships registered in the agricultural sector. | MTE |
Employment (industry) | Number of formal employment relationships registered in the industrial sector. | MTE |
Employment (services) | Number of formal employment relationships registered in the service sector. | MTE |
Employment (trade) | Number of formal employment relationships registered in the industrial sector. | MTE |
Employment (civil construction) | Number of formal employment relationships registered in the industrial sector. | MTE |
Source: compiled by the authors.
4. Results
To verify the spatial correlation referring to the results of Banpará’s policies, first, we evaluated the existence of a cluster concerning the number of the bank’s branches in the municipalities in December 2020. Then, the queen method was used as a weighing matrix, considering the relation of all border municipalities as neighbors.
The result indicates that Moran’s I= 0.090 is positive, revealing spatial autocorrelation. The result means that municipalities with a higher number of Banpará branches are neighbors of municipalities with a large number of branches. This correlation, however, is not high, as shown in the gradient of the straight line in Figure 3.
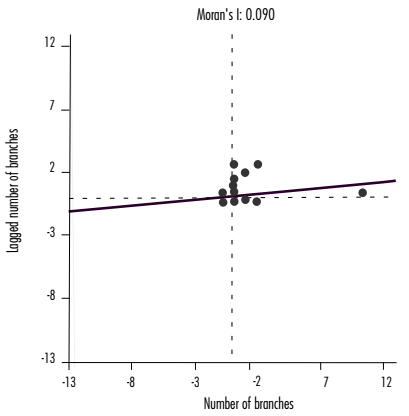
Note: software GEODA. Data from IBGE (2021) and BACEN database (2020).
Sources: compiled by the authors.
Figure 3 Moran’s scatterplot of Banpará branches per municipality
In Figure 4, we can see a pattern of high-high autocorrelation in the municipalities in dark gray, especially in the metropolitan region of Belém. This indicates that the region has formed a cluster representing a high concentration of Banpará branches. Additionally, municipalities indicated as high-low and low-high indicate a higher concentration of branches in these municipalities.
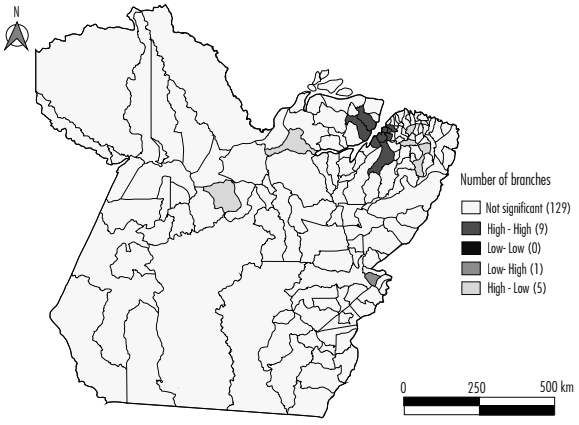
Sources: compiled by the authors.
Note: software GEODA. Data from IBGE (2021) and BACEN database (2020).
Figure 4 Cluster of municipalities with Banpará branches
The same analysis was conducted for private or public banks and all branches throughout the State of Pará. Banpará presented the highest number of municipalities with spatial autocorrelation. Regarding credit availability, we verified the behavior of rural and real estate credit. The statistics of Moran’s I presented a low but positive outcome (0.035), demonstrating the existence of low autocorrelation for the availability of rural credit at Banpará branches.
Following this methodology, we aimed to estimate cluster formation within the production variables, i.e., the aggregate value of agriculture and cattle ranching and services. The Moran scatterplot for the agricultural and cattle ranching value presented a result of 0.191. Thus, we can affirm that there is a positive autocorrelation. The municipalities with a high agricultural and cattle ranching aggregate value also have neighbors with increased agricultural and cattle ranching aggregate values. This occurs mainly in the Lower Amazon region, such as Óbidos, Alenquer, Monte Alegre, Curuá, Juruti, and their neighbors, corresponding to around 12% of the agricultural value added in Pará.
It is worth mentioning that the service value-added, except for administration, defense, education, public health, and social security, presented by the Moran’s I scatterplot has a Moran’s I = 0.020, thus concluding that there is a negative autocorrelation related to the value-added of services in Pará. In contrast, when we analyze the value-added, including the variables of administration, defense, education, public health, and social security, there is a positive autocorrelation with a positive Moran’s I = 0.078. A concentrated high-high cluster is also found in the metropolitan region and Tocantins.
Based on the results obtained, we can highlight the variable GDP that directly influences the arrangement of branches in the territory following the “money route”, in which the banks tend to concentrate in cities with a higher income. In this context, we also verified Moran’s I for such a variable, presenting Moran’s I positive spatial autocorrelation = 0.096. With these results, there is a low-low cluster, which identifies that the municipalities with low GDP also have low GDP neighbors. This low-low pattern can be observed especially in Marajó and the northeast region of Pará. Nevertheless, despite its significance, the results demonstrated that the variables have a weak spatial dependence, except for the municipalities in the metropolitan region and some regions with clusters in the analyzed variables. Thus, it was decided not to consider the spatial effects of the impact assessment analysis.
As for the realization of the estimations, the 143 municipalities of the State of Pará, registered by the Brazilian Institute of Geography and Statistics in 2010 (IBGE, 2021), were divided into two groups treatment and control (see Table 3). As a treatment policy, the presence of Banpará branches in the municipality was applied. This data was obtained from the Central Bank of Brazil’s Banking Statistics by Municipality program (BACEN). Each municipality can be allocated to one of two groups: treatment when it has branches or control when it has not.
Table 3 Distribution of municipalities in Pará in Jan/2019 according to treatment and control
Total municipalities | Treatment | Control group |
---|---|---|
143 | 99 | 44 |
Source: compiled by the authors according to data from BACEN.
The preliminary database analysis showed a significant difference in the data from the non-treated groups and treated municipalities except for GVA agribusiness. It is also possible to note that the variables have significantly higher results in municipalities with the Banpará branch (see Table 4).
Table 4 t-statistic of the average sectorial data in 2019 without matching
Variable | Mean for the control group (BRL$) | Mean for the treatment group (BRL$) | P-value |
---|---|---|---|
GDP per capita | 12 210.81 | 20 777.44 | 0.04474(*) |
GDP | 280 875 467.00 | 1 675 235 215.00 | 0.0016(**) |
ISS per capita | 45.75 | 138.16 | 0.028(*) |
ISS | 1 114 593.00 | 13 043 384.00 | 0.01377(*) |
Average salary | 1 880.80 | 2 041.40 | 0.0075(***) |
GVA (services) | 50 140 843.00 | 553 255 390.00 | 0.011 (*) |
GVA (industry) | 21 170 169.00 | 550 881 366.00 | 0.01002(**) |
GVA (agricultural) | 97 911 439.00 | 107 061 198.00 | 0.7295 |
GVA (public services) | 100 754 178.00 | 302 643 415.00 | 0.00083(***) |
Note: Rstudio software. Significance codes: 0.000 (***) significant at 0.01%; 0.001 (**) significant at 0.1%; 0.01 (*) significant at 1%; 0.05 (.) significant at 5%; 0.1 ( ) significant at 10%.
Source: compiled by the authors.
The logit regression model was estimated for the effect of GDP per capita on the possibility of having Banpará branches in the municipalities. The results showed, with a significance of at least 0.1%, that the increase in 1 unit in 2010 GDP per capita significantly influences the presence of the bank. The result verifies whether or not Banpará’s presence was random and to check the validity of the difference of means with unbiased ATE (see Table 5).
Table 5 Estimation of the binomial model (logit)
Variable | Coefficient estimate | Standard error | P-value |
---|---|---|---|
GDP per capita | 1.0002364 | 0.00008656 | 0.00632 (**) |
Note: Rstudio software. Significance codes: 0.000 (***) significant at 0.01%; 0.001 (**) significant at 0.1%; 0.01 (*) significant at 1%; 0.05 (.) significant at 5%; 0.1 ( ) significant at 10%.
Source: compiled by the authors.
Thus, we verified that there is effectively no randomness in the distribution of branches since the domestic product of municipalities directly influences the installation of new ones. Therefore, it is necessary to perform matching to ensure non-biased results by the performance of other sectors of the local economy. The larger the matching sample and the comprehensiveness of the variables for pair formation, the more reliable the estimates for assessing the impact of the policy since the municipalities would constitute a more robust comparison group.
Artificial comparison groups were created using the propensity score matching method based on GDP per capita and Employment (civil construction). The 2010 GDP per capita grouped the municipalities through productivity parameters, the consumer market. Employment constitutes a parameter for verifying the economic dynamism of municipalities in terms of labor relations and local infrastructure development.
The propensity score represents, through a score from 0 to 1, the probability of each municipality receiving treatment. The matched variables of the comparison groups did not mandatorily present an overlap of their values. Therefore, we used a caliper optimization filter to match, with a margin of 0.02 in the differences in the common support data.
According to Gertler et al. (2018), this is the most appropriate overlap between the propensity scores of the treatment or enrolled group and those of the non-written group.
As a sample, a set of 68 municipalities (34 in each group) (out of the total 143) were paired by GDP per capita and Employment (construction). Figure 5 presents the graphical distribution of propensity scores between paired and unpaired municipalities for the control and treatment samples. The pairing tends to level down the scores since a large part of the control group’s municipalities are in a political and economic backlog.
Table 6 shows the average estimates of propensity scores between municipalities in the control and treatment groups for the samples with and without pairing. The average numbers presented show an approximation of 99.6% in the propensity scores after pairing, which considerably improves the possibilities of comparison for verifying the causality of Banpará’s influence on the variables between treated and non-treated municipalities.
Table 6 Pairing per Propensity Score (PPS) for the 2010 GDP per capita jointly with the employment in civil construction in 2010
Propensity score: control | Propensity score: treatment | Standard mean difference (%) | Propensity score: matched control | Propensity score: matched treatment | Standard mean difference (%) |
---|---|---|---|---|---|
0.5828 | 0.7410 | 79.83 | 0.5590 | 0.5596 | 0.32 |
Source: compiled by the authors.
Once the matching sample is established, the average treatment effect can be estimated. The impact of the presence of Banpará branches in the treated municipalities can be evaluated with a low risk of bias since the PPS methodology has grouped the municipalities that have GDP per capita and Employment (construction) as closely as possible into similar groups, based on the caliper optimization filter.
In Table 7, the estimated t-statistic demonstrates a significant approximation if compared to the sample without pairing but still indicates variations between them. In general, Banpará’s presence was more significant in municipalities with positive GDP, services, industry, and ISS variables, results representing a solid contribution to developing the productive forces and institutions of the municipalities in which it operates. However, the causality of the bank’s impact was only verified based on two variables: the GVA (services), with a 5% significance, and the GVA (public services), also with a 5% significance, to which we will include the evaluation of the ATE (see Table 7).
Table 7 t-statistic of the average sectorial data in 2019 with pairin
Variable | Mean for the control group (BRL$) | Mean for the treatment group (BRL$) | P-value |
---|---|---|---|
GDP per capita | 10 610 | 9 679 | 0.3846 |
GDP | 243 192 384 | 310 606 238 | 0.2599 |
ISS per capita | 39.15 | 29.44 | 0.311 |
ISS | 948 556 | 837 418 | 0.7551 |
Average salary | 1 848 | 1 939 | 0.1803 |
GVA (services) | 42 211 012 | 61 938 202 | 0.048 (*) |
GVA (industry) | 12 668 705 | 15 893 038 | 0.5008 |
GVA (agricultural and livestock) | 89 166 115 | 93 517 368 | 0.903 |
GVA (public services) | 90 026 947 | 126 293 021 | 0.009 (*) |
Note: significance codes: 0.000 (***) significant at 0.01%; 0.001 (**) significant at 0.1%; 0.01 (*) significant at 1%; 0.05 (.) significant at 5%; 0.1 ( ) significant at 10%.
(.) Source: compiled by the authors.
In comparison, Table 8 shows an estimation in BRL$ 474 098 629.00 for the average effect of the presence of GVA (services) in the sample without pairing, with a value-p relevant at 1%. As for the paired sample, the ATE was estimated in BRL$ 127 770 276.00 for GVA (services), with a significance of the p-value at 5%. As for GVA (public services), the non-paired ATE was estimated in BRL$ 189 210 502.00, with significance at 0.1%, while for the paired sample, the value of ATE was estimated in BRL$34.067.367,00, significant at 1%.
Table 8 Average treatment effect in the GVA of services and public services
Variable | Average treatment effect without pairing | Mean effect of treatment with pairing(BRL$) |
---|---|---|
GVA (services) | 503 114 547 | 19 727 190 |
GVA (public services) | 201 889 237 | 36 266 074 |
Note: significance codes: 0.000 (***) significant at 0.01%; 0.001 (**) significant at 0.1%; 0.01 (*) significant at 1%; 0.05 (.) significant at 5%; 0.1 ( ) significant at 10%.
Source: compiled by the authors.
Therefore, we can conclude that the presence of Banpará branches grants the municipalities a higher gross value added (GVA) in the sector of services and public services, according to the 2019 data. At the same time, the average effect of having branches is approximately BRL$ 19 million in the GVA of services and BRL$ 36 million in the GVA of public services. In this sense, the presence of Banpará branches ensured, in 2019, an average of 9% more in the production of services and 15% in the value of public administration.
In turn, the DD method is employed to estimate the effect of Banpará branches, comparing the modifications in the results over time in municipalities with a branch and the municipalities that do not have them (treatment and control). It requires pre- and post-intervention data, which refers to the information of municipalities in which the bank opened branches during that time and municipalities that did not receive any branches. Panel data estimations were done using two selected periods, 2010 and 2019. The choice of periods was based on the branch expansion policy starting in 2010 and the choice of more recent municipal economic effects data before the pandemic in 2019.
According to the result of the Hausman test, the fixed or random effects are selected. If the test was insignificant, suggesting no correlation between the fixed effects and the independent variables, the fixed effects model is preferable as it more accurately controls unobserved individual effects, ensuring consistent parameter estimates.
Table 9 presents the results obtained from employing OLS models with fixed effects to analyze the changes in GDP per capita over the period under investigation, specifically examining the impact of the presence of Banpará branches on this economic variable. The findings indicate that establishing a causal relationship between the presence of Banpará and GDP per capita is unattainable. Nevertheless, a noteworthy and consistent long-term increase in GDP per capita of around BRL$ 12 thousand is worth noting, as evidenced by the variable “Time” results.
Table 9 Difference-in-differences results: GDP per capita
Pooling MQO | Fixed effects | |||
---|---|---|---|---|
Time | 12 272** | 12 280** | 12 273* | 11 402* |
(4 207) | (4 215) | (5 083) | (5 130) | |
Treated | 5 485 | -224.6 | 252.7 | 3 642 |
(4 738) | (4 918) | (7 282) | (7 820) | |
DD | 8 462 | 8 587 | 8 532 | 4 415 |
(6 384) | (6 433) | (7 834) | (8 568) | |
Constant | 6 512 | -48.58 | ||
(2 241) | (2 266) | |||
Population | 0.002 | 0.0304 | ||
(0.011) | (0.258) | |||
R² | 0.14 | 0.14 | 0.23 | 0.24 |
N | 286 |
Note: significance codes: 0.000 (***) significant at 0.01%; 0.001 (**) significant at 0.1%; 0.01 (*) significant at 1%; 0.05 (.) significant at 5%; 0.1 ( ) significant at 10%.
Source: compiled by the authors.
Table 10 presents the findings regarding average salary, specifically examining the impact of Banpará branches on the average wage within municipalities. The results reveal a marginal effect whereby the presence of Banpará branches contributes to a favorable increase in the average salary by approximately BRL$ 134. It implies that municipalities with Banpará branches exhibited higher average salary values in 2019. Furthermore, the “Treatments” variable also demonstrates significance, indicating a notable difference of BRL$ 128.65 in average salary between municipalities with and without a Banpará branch. The “Time” variable also marginalizes the average salary, amounting to approximately BRL$ 900 between the two years.
Table 10 Difference-in-differences results: 2019 average salary
Pooling MQO | Fixed effects | |||
---|---|---|---|---|
Time | 902.14*** | 905.1*** | 946.27*** | 935.92*** |
(53.75) | (50.77) | (41.57) | (41.71) | |
Treated | 128.65* | 36.84 | -24.629 | 15.65 |
(60.17) | (59.24) | (59.555) | (63.59) | |
DD | 123.21 | 139(.) | 134.966* | 86.02 |
(82.72) | (85.29) | (64.072) | (69.68) | |
Constant | 916.70*** | 894.7*** | ||
(28.64) | (27.3) | |||
Population | 0.008*** | 0.00361(.) | ||
(0.0013) | (0.002) | |||
R² | 0.75 | 0.77 | 0.93 | 0.93 |
Note: significance codes: 0.000 (***) significant at 0.01%; 0.001 (**) significant at 0.1%; 0.01 (*) significant at 1%; 0.05 (.) significant at 5%; 0.1 ( ) significant at 10%.
Source: compiled by the authors.
Table 11 presents the results obtained for the ISS, revealing a positive and statistically significant outcome of BRL$ 5 million associated with the installation of Banpará.
Table 11 Differences-in-differences results: ISS
Pooling MQO | Fixed effects | |||
---|---|---|---|---|
Time | -702 705 | 258 100 | 158 841 | 57 921 |
(6 398 701) | (2 637 000) | (1 764 941) | (1 543 300) | |
Treated | 24 143 187*** | -5 756 000 (.) | -3 401 792 | -3 597 100 |
(7 206 848) | (3 077 000) | (2 496 500) | (2 096 500) | |
DD | -12 196 432 | 3 997 000 | 5 223 703(.) | 2 830.8 |
(9 710 347) | (4 025 000) | (2 727 052) | (2 400) | |
Constant | 1 804 861 | -53 540 00*** | 7 874 944** | -5 862 000 |
(3 409 199) | (1 418 000) | (3 044 266) | (1 384 600) | |
Population | 261.2*** | 260.90 | ||
(7.03) | (8.93) | |||
R² | 0.05 | 0.2 | 0.02 | 0.7 |
Note: significance codes: 0.000 (***) significant at 0.01%; 0.001 (**) significant at 0.1%; 0.01 (*) significant at 1%; 0.05 (.) significant at 5%; 0.1 ( ) significant at 10%.
Source: compiled by the authors.
Tables 12 and 13 display the impacts of Banpará on earnings, taking into account the weighting by the propensity score calculated in the preceding section using probabilities derived from the logit model for each municipality. Using weights in the analysis enhances the robustness of the findings concerning the influence of Banpará’s establishment on the rise in average wages. The results indicate that this effect may change when employing the weighted model. Consequently, the influence of Banpará’s presence on wages is estimated to be around BRL$ 131, while the impact on ISS amounts to approximately BRL$ 6 million. Thus, municipalities that set up branches between 2010 and 2019 have average salaries 6% higher and ISS 64% higher than those that did not.
Table 12 Difference-in-differences results with PSM: Average salary
Pooling MQO | Random effects | |||
---|---|---|---|---|
Time | 900.27*** | 904.2*** | 923.914*** | 929*** |
(62.09) | (58.28) | (44.53) | (43.41) | |
Treated | 136.45* | 41.22 | 51.277 | 9.9 |
(60.18) | (58.50) | (49.54) | (48.64) | |
DD | 97.39 | 142.2(.) | 122.18(.) | 131.02* |
(86.28) | (81.3) | (62.78) | (6.123) | |
Constant | 936.73*** | 914.2*** | 957.342*** | 917.36*** |
(31.98) | (30.23) | (30.06) | (29.26) | |
Population | 0.000762*** | 0.0008*** | ||
(0.0001) | (0.0001) | |||
R² | 0.72 | 0.76 | 0.87 | 0.87 |
Note: significance codes: 0.000 (***) significant at 0.01%; 0.001 (**) significant at 0.1%; 0.01 (*) significant at 1%; 0.05 (.) significant at 5%; 0.1 ( ) significant at 10%.
Source: compiled by the authors.
Table 13 Difference-in-differences results with PSM: ISS
Pooling MQO | Random effects | |||
---|---|---|---|---|
Time | -1 288 519 | 55 080 | 76 070 | 45 969 |
(8 178 537) | (3 323 000) | (2 255 004) | (1 945 700) | |
Treated | 27 037 975*** | -5 767 000(.) | -4 190 323 | -4 227 700 |
(7 927 785) | (3 336 000) | (2 729 342) | (2 260 900) | |
DD | -11 454 641 | 3 981 000 | 6 052 941(.) | 3 031 000 |
(11 365 849) | (4 636 000) | (3 133 871) | (2 730 300) | |
Constant | 2 501 957 | -5 274 000** | 11 172 925** | -5 787 000 |
(4 212 414) | (1 724 000) | (3 595 070) | (1 639 900) | |
Population | 262.6*** | 263.10 | ||
(6.953) | (8.750) | |||
R² | 0.05 | 0.84 | 0.02 | 0.75 |
Note: significance codes: 0.000 (***) significant at 0.01%; 0.001 (**) significant at 0.1%; 0.01 (*) significant at 1%; 0.05 (.) significant at 5%; 0.1 ( ) significant at 10%.
Source: compiled by the authors.
In summary, the presence of Banpará branches in the municipalities of the State of Pará contributed to a higher (GVA) in the services and public services sectors, based on data from 2019. The average effect of having Banpará branches was estimated to be around BRL$ 19 million on the GVA of services and BRL$ 36 million on the GVA of public services. It indicates that Banpará branches resulted in an average increase of 9% in the production of services and 15% in the value of public administration in 2019. Additionally, Tables 14 and 15 present the impacts of Banpará on earnings, accounting for propensity score weighting derived from the logit model. The results, obtained using the DD methodology, reveal that Banpará’s presence is associated with an estimated increase of approximately BRL$ 131 in average wages and BRL$ 6 million in ISS. Consequently, municipalities that installed Banpará branches between 2010 and 2019 exhibited 6% higher average salaries and 64% higher ISS than municipalities without such branches.
These findings highlight the critical role played by Banpará’s presence in fostering economic growth and development in the region. The results found in this research, in which the credit made available by banks has real effects on the economy and variables related to economic growth, are compatible with the work of Romero and Jayme Jr. (2009), Taques et al. (2014) and Barbosa et al. (2015) and Quaresma et al. (2019).
Firstly, the higher (GVA) observed in the services and public services sectors represent the positive economic impact of Banpará’s presence. It suggests that development banks can contribute significantly to the production of services and the value of public administration, promoting economic growth and development in these sectors. Moreover, the increase in average wages and tax revenues in municipalities with Banpará branches highlights the potential of development banks to enhance income levels and generate additional fiscal resources. It indicates that development banks can improve living standards and boost local economies. Therefore, the positive outcomes demonstrated by Banpará’s presence make a strong argument for the implementation of development banks in underdeveloped regions like Pará, as they have the potential to drive economic growth, increase employment opportunities, and foster overall development.
5. Final considerations
The municipalities of the State of Pará have a significant heterogeneity concerning the respective stages of their socioeconomic development. It is possible to observe that some regions, such as the metropolitan area, have more substantial growth potential and react differently to incentives. Private banks, in general, tend to concentrate in these regions. However, this paper demonstrates that Banpará is significantly present in municipalities with less development, emphasizing the development policy implemented to expand the bank.
The spatial analysis of Banpará’s spillover effect on neighboring municipalities verified whether there was a spatial correlation. Based on this estimation, no significant results were obtained among the variables, except for the occurrence of a banking network in the metropolitan region and the variable indicating agricultural production. In conclusion, the presence of Banpará branches in the municipalities of the State of Pará positively impacts the local economy. Installing these branches is associated with higher GVA in the services and public services sectors. The average effect of Banpará’s presence translates into increased GVA of services and public services, with approximate values of BRL$ 19 million and BRL$ 36 million, respectively. It indicates a significant contribution to the production of services and the importance of public administration. Additionally, the analysis of average wages and ISS reveals that municipalities with Banpará branches experience higher average salaries and tax revenues than those without such branches. The estimated increases in average wages and ISS amount to approximately BRL$ 131 and BRL$ 6 million.
The findings reinforce that development banks, including public banks like Banpará, are crucial in countering economic downturns and promoting economic development. They can act as countercyclical instruments during recessions by providing credit and liquidity when private banks are reluctant to lend. Additionally, these banks contribute to economic development by directing credit towards essential areas such as infrastructure and meeting consumer needs. It is particularly significant in less developed countries where financing for investment and innovation can be challenging. Development banks can focus on socio-economic development and allocate credit to specific sectors or regions, addressing economic disparities and fostering inclusive growth by strategically channeling credit to sectors with high development potential, such as infrastructure and consumer credit. Development banks can stimulate economic growth, reduce inequalities, and contribute to inclusive economic development.