1 Introduction
A recent benchmark study has shown that bringing a new pharmaceutical drug product to the market exceeds an investment of two thousand million (US) dollars and takes over ten years of development [35]. Consequently, there is an ever-growing effort to apply new technologies that could help streamline the discovery process. The publication of the article "Next Industrial Revolution:
Designing Drugs by Computer at Merck”, in the early 1980s in the prestigious business magazine Fortune, marked the first public credit to the enormous potential of computers assisting the design of new drugs [1].
Over the past two decades, the explosive advancements in high-performance computing [32], together with the increasing availability of 3-dimensional structures of important pharmaceutical targets, have paved the way to the computer-aided design of drugs.
Particularly, the now wide spread access to supercomputers and the development of more efficient algorithms have positively impacted the discovery and development of new drugs. Computer-aided drug design (CADD) uses computational approaches to discover, develop, and analyze drugs [28].
Although CADD is an emerging discipline, its foundations date back to the seminal works of Emil Fischer (1894) [14] and Paul Ehrlich (1909) [12] when they proposed the lock and key model.
This model rationalizes the mechanism of drug action: a receptor structure (the lock) contains a region with a particular shaped pocket at which an appropriately shaped drug (the key) can interact [6]. Though a number of biomolecules within the human body can work as a receptor, protein molecules frequently play this role. In the same way in which a key should open only one lock - otherwise security may be compromised - drugs should be designed to act on only one receptor [1]. Deleterious side effects are due, at least in part, to the fact that the drug molecule interacts not only with its target receptor but also with other proteins not associated with the disease that it is proposed to treat.
Human serum albumin (HSA), the most abundant protein of the circulatory system, represents a very typical example of a promiscuous protein receptor that interacts with a broad range of drugs impacting their efficacy and safety [4, 31, 26]. This protein plays a crucial role in regulating the osmotic pressure and in transporting endogenous compounds such as fatty acids, hormones, toxic metabolites and metals. Due to its high capacity of binding a wide range of drugs, HSA determines, to a large extent, the safety and pharmacokinetics profile of most drugs. Regardless of the method of administration (e.g. oral, intravenous), drugs are transported through the blood, where they inevitably interact with the human serum albumin (HSA) before reaching their receptors.
Hence, drug molecules can circulate in the bloodstream either bound to HSA (or other plasma proteins) or in their unbound (free) form [26]. Interestingly, only unbound drug molecules can interact with the protein receptor to trigger their therapeutic effect [41]. Moreover, the distribution and elimination of a drug from the body depends on the strength with which it is bound to HSA [17].
Therefore, the study of the binding of drugs to HSA, at the molecular level, results of paramount importance to better outline the pharmacokinetics profile of drugs [23]. Molecular docking is central to the CADD strategies. The computational-based fitting of the 3D structure of a molecule (ligand) within the binding site of a protein receptor is called docking [8].
The docking process, in general, involves two independent steps: (1) the generation of the correct pose (conformation and orientation) of ligands inside the receptor (protein) binding site and (2) the evaluation of the affinity of binding. [8].
Over the last few decades, docking techniques have successfully been incorporated to the standard laboratory experiments to the discovery of new drugs. This strategy has importantly contributed to reducing both the experimental work and the associated costs [1].
Although there are currently a wide range of docking software alternatives, AutoDock Vina [45] remains one of the top choices due to its high reliability [7]. AutoDock Vina is an open source program, free for academic use, that employs the 3D structures of the receptor and ligand to predict the best way of molecular interaction [45]. In the first stage, AutoDock Vina samples the conformational space to explore possible modes of protein-ligand interaction. Subsequently, in the second stage, the program uses a scoring function to determine the most suitable pose in terms of the energy of interaction [21].
The aim of the current study is to harness the significant increase, in recent years, of computing power, and the availability of 3D crystal structures of the HSA to develop a computational method that incorporates molecular docking, using AutoDock Vina, to predict the interactions of drugs with this plasma protein, which may potentially lead to a streamlined drug discovery process.
2 Methods
Figure 1 shows the overall methodology that was applied to conduct this study and some of the tools that were employed.
Protein-ligand docking requires as input a three-dimensional (3D) ligand database and the 3D structure of the protein receptor. Affinity of selected drug ligands against the human serum albumin (HSA) receptor were investigated using AutoDock Vina.
Before docking, ligand database and HSA receptor were prepared. Open Babel was used to the generation of 3D structures of ligands and to their optimization. HSA was prepared using, Scwrl4, Reduce and Gromacs. Finally, predicted affinity was compared with reported biological activity.
2.1 Receptor Preparation
The 3D crystal structure of human serum albumin (PDB ID 2BXH) [15] was retrieved from the Protein Data Bank [3] and subjected to an initial preparation phase where all water molecules and solutes contained in the structure were removed, including the ligand indoxyl sulfate, which was co-crystallized with the protein. Subsequently, the structure was inspected with Scwrl4 [27] to add missing atoms and side chains and to optimize them with respect to the entire hydrogen bond network of the protein. Scwrl4 uses an energy function based on a backbone-dependent rotamer library to estimate the positioning of residue side chains. When elucidating X-ray crystal structures, misorienting Asp and Glu side chains and His rings is quite common (except at extremely high resolutions) because the electron density is symmetric around these residues. The Reduce [49] program, included in the MolProbity server [48], was used to identify the preferred orientation for these side chains based on van der Waals contacts and H-bonding. In this process, flipping was accepted when the program indicated “clear evidence for flipping.” Reduce software conducts surface protein analysis, employing all explicit hydrogen atoms and van der Waals contacts, to choose the most suitable side chain orientations of Asn, Gln, and His amino acids [49]. The protein was then energy minimized to obtain a stable, low energy conformation. The protein was minimized in vacuo, with no harmonic restraints, using the steepest descent minimizer of Gromacs 4.5.4 [18].
Protonation states were assigned at pH=7.4 using the PDB2PQR [11] software, which incorporates Propka [29] to the calculation of the pKa protein values. Then Gasteiger charges were added and the receptor file converted to the pdbqt format using the “prepare_receptor4.py” script of AutoDockTools [33]. Finally, the quality of the protein receptor was assessed using a series of tests for structural consistency and reliability. The stereochemistry of the refined structure was evaluated through the analyses of Ramachandran plots [39] using the MolProbity server [48]. The statistics of non-bonded residue interactions, which determines the amino acid environment, was assessed with the ERRAT program [10]. This tool helps discriminate between correctly and incorrectly determined regions of protein structures based on characteristic atomic interactions [10].
2.2 Ligand Preparation
A total of 20 chemically and functionally highly-diverse drug molecules (Figures 2 and 3), for which association constants (ka) towards HSA had been determined, were identified from a literature search [51].

Fig. 2. Two-dimensional chemical structures of drugs interacting at the drug-binding site 1 of human serum albumin (HAS)

Fig. 3. Two-dimensional chemical structures of drugs interacting at the drug-binding site 2 of human serum albumin (HAS)
In order to utilize these molecules in the docking procedure, their two-dimensional (2D) structures, in smile format, were retrieved from the PubChem database (https://pubchem.ncbi.nlm.nih.gov/). Smiles strings were subsequently converted to 3-dimensional (3D) coordinates using the “gen3d” option of the Open Babel package [16], version 2.4.0 (http://openbabel.org).
Open Babel package was also used to remove salts and counterions, if present, to energy minimize and to assign protonation states (at pH=7.4) to ligand structures. Finally, the “prepare_ligand4.py” script included in AutoDockTools [33] was employed to assign Gasteiger charges and to convert ligands to the pdbqt format.
2.3 Molecular Docking
Molecular docking is a computationally-based procedure to the prediction of the binding mode and binding affinity of a ligand (drug molecule) with a protein (receptor) of known 3D structure. AutoDock Vina [45] version 1.1.2 was used to dock drug molecules into the drug-binding sites of the HSA protein.
The input of the protocol includes the 3D structure of the protein receptor and those of the ligands, and a defined search conformational space. The output yields a set of poses (receptor-ligand) ranked by ΔG, the predicted energy of binding in kcal/mol. AutoDock Vina was set to generate a maximum of 20 poses, through the num_modes command. This program performs a user-defined number of individual sampling runs through the “exhaustiveness” parameter. In each run, the program perturbates the ligand structure to sample different three-dimensional arrays of the drug molecule within the active site of the receptor followed by a local optimization, using the Broyden-Fletcher-Goldfarb-Shanno algorithm [37].
The resulting ligand conformation is either accepted or rejected according to a Metropolis criterion [45]. Subsequently, the scoring function evaluates multiple times each local optimization. The number of scoring function evaluations is determined heuristically, and depends on the number of rotatable bonds of the ligand and whether the receptor contains flexible amino acids [45].
The protein receptor was maintained rigid throughout the docking process, while drug molecules were allowed flexibility. The search space was defined to cover two distinct HSA drug-binding sites. Binding site I was covered within a region with coordinates at the center along the X, Y and Z axis as -4.326, -4.756 and 9.449, and dimensions (Å) along the X, Y and Z axis were set to 10 Å. In the case of binding site II, the search space region was centered at the coordinates (X, Y, Z) 9.187, 3.021, -14.306. And the dimensions along these axes were also set to 10 Å. All other parameters were kept as the AutoDock Vina default values.
2.4 Validation of AutoDock Vina Performance
The performance of AutoDock Vina was evaluated assessing whether this program could reproduce accurately the experimental binding mode of ligand indoxyl sulfate within the X-ray crystal structure of Human Serum Albumin (PDB ID 2BXH) [15]. The root-mean-square deviation (RMSD) cut-off of 2 Å was used as a criterion of the correct bound prediction [38].
Also, in a comparative study, the correlation of predicted affinity of binding with experimentally determined associations constants was evaluated as a measurement of the precision of the protocol.
Structure analysis and visualization were performed using PyMOL (https://pymol.org/2/) and lipophilic potential was calculated using VASCo, version 1.02 [43].
3 Results
3.1 Receptor Refinement and Preparation
Using state-of-the-art computational techniques, including side-chain protein optimization and energy minimization, we highly refined the structure of Human Serum Albumin (HSA) from the experimentally determined X-ray crystal. Figures 4 and 5 shows that our refined structure of HSA is composed of a single chain that contains three α-helical homologous domains, namely, I (residues 1-195), II (residues 196-383), and III (residues 384-585). Each domain is divided into subdomains A and B. The protein features a heart-shaped structure with an approximate dimension of 80 x 80 x 30 Å. Studies conducted by Sudlow and co-workers [44] showed the presence of two distinct binding sites. Binding site I is located within the core of subdomain IIA including all its six helices and also a loop-helix fragment (residues 148-154) contributed by subdomain IB.
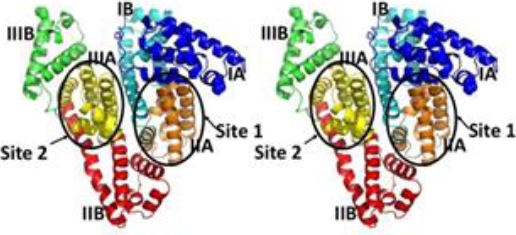
Fig. 4. Stereo view of the overall structure of the refined model of human serum albumin (HSA). Subdomain IA is shown in dark blue; subdomain IB, in light blue; subdomain IIA, in orange; subdomain IIB, in red; subdomain IIIA, in yellow; subdomain IIIB, in green

Fig. 5. Stereo view of the hydrophobic and hydrophilic potentials of the human serum albumin mapped onto a surface representation. Blue: area of predicted highest hydrophilic potential; yellow: area of predicted highest hydrophobic potential
Although the pocket that defines this binding site contains predominantly apolar residues, two groups of hydrophilic residues, Tyr150, His242, Arg257 and Lys195, Lys199, Arg218, Arg222 are also displayed in this pocket. Binding site 1 allocates a series of therapeutically important drugs such as warfarin, phenylbutazone, furosemide, among others (Figure 2).
Drug-binding site 2 comprises the six α-helices of subdomain IIIA and predominantly includes a largely hydrophobic cleft measuring about 16 Å deep and 8 Å wide with a positively charged residue positioned near the surface (Figures 4 and 5).
Although topologically binding site 2 is similar to binding site 1, the former shows some key differences. Pocket of binding site 2 presents a smaller size, and unlike site 1, only contains a patch of hydrophilic residues, including Tyr411, Arg410, Lys414 and Ser489 (Figures 4 and 5).
Binding sites 1 and 2 are distinguishable not only in terms of structural architectures but also in terms of hydrophobicity profile, which ultimately determines the different binding specificities for both pockets. Figure 3 shows the structures of some drugs that bind site 2 (e.g. diazepam, ibuprofen, naproxen, ketoprofen).
3.2 Refined Structure Validation
The quality of the refined model was assessed using MolProbity, a server that includes the generation of Ramachandran plot analysis for structure verification. Figure 6 shows that the structure possesses 98.9% residues located in the most favoured regions of the plot and therefore they are stereochemically valid.

Fig. 6. Ramachandran plot of the refined model of human serum albumin (HAS). 98.9% of the residues were located in the most favored regions
The non-bonded residue interactions were further evaluated by means of the ERRAT analysis. Our refined structure showed a 94.2% ERRAT score. High quality structures generally show >85% score [10].
The consistency of the folding pattern, the geometric quality of the backbone, and the non-bonded residue interactions of the refined model are within the limits established for high quality structures. Therefore, the refined model of human serum albumin can be reliably used in further structural studies.
3.3 Validation of the Performance of AutoDock Vina
One approach to the validation of a docking program is to inspect whether the predicted mode of binding (docked conformation) of a ligand within the receptor is capable of reproducing the bound ligand in the experimental crystal structure. Consequently, in order to evaluate the reliability of AutoDock Vina, the bound ligand indoxyl sulfate was removed from the crystal structure of human albumin (PDB ID 2BXH) and re-docked.
AutoDock Vina had the ability to reproduce the positioning of the ligand within the crystal structure of human serum albumin as shown in Figure 7. Molecular docking of indoxyl sulfate within the crystal structure of HSA resulted in an RMSD value of <2 Å for the top 3 predicted poses (Figure 7). Also, the number and the nature of the interactions of these poses were consistent with those of the co-crystallized ligand indoxyl sulfate (Table 1).
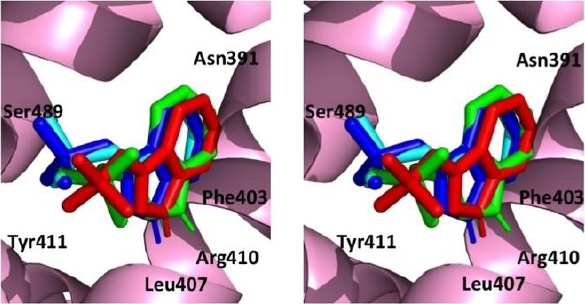
Fig. 7. Stereo view of the re-docking of indoxyl sulfate using AutoDock Vina. Top 1 pose (green), top 2 pose (light blue) and top 3 pose (dark blue) compared with the co-crystallised ligand indoxyl sulfate (red) viewed from the outside of the internal cavity of human serum albumin (HAS)
Table 1 Interactions of co-crystallized indoxyl sulfate with human serum albumin (Ids-HSA*). For comparison, the interactions of the top 3 poses obtained from re-docking experiments are shown
Ids-HSA* X-ray crystal structure | Pose 1 | Pose 2 | Pose 3 | |
---|---|---|---|---|
Hydrophobic interactions | Ile388 | ✘ | ✘ | ✘ |
Asn391 | ✔ | ✔ | ✔ | |
Phe403 | ✔ | ✘ | ✘ | |
Leu407 | ✔ | ✔ | ✔ | |
Arg410 | ✔ | ✘ | ✘ | |
Tyr411 | ✔ | ✔ | ✔ | |
Leu430 | ✔ | ✔ | ✔ | |
Gly434 | ✔ | ✔ | ✔ | |
Ser489 | ✘ | ✔ | ✘ | |
Hydrophilic interactions | Arg410 | ✔ | ✘ | ✘ |
Tyr411 | ✔ | ✘ | ✔ | |
Leu430 | ✔ | ✔ | ✔ |
3.4 Correlation of Predicted Affinities with Experimentally Determined Association Constants (Ka)
Many studies have investigated the interactions of human serum albumin with drugs using spectroscopic studies such as fluorescence quenching [26, 51, 34, 42, 22, 36, 47, 5, 13, 30]. These studies have allowed the quantification of equilibrium binding of drugs to purified human serum albumin and provided an estimate of the constant of association (Ka). In a comparative analysis, we have subjected some of these drugs to computational docking simulations to investigate whether exists a potential correlation between predicted affinities and experimentally determined association constants.
A highly diverse set of 20 drugs (Figures 2 and 3) was prepared and all molecules docked into the refined model of human serum albumin using the docking protocol described in the methods section. Predicted affinities were then compared with reported experimentally determined association constants (Table 2).
Table 2 Predicted affinity (free energy of binding) of drugs docked, using AutoDock Vina, into the refined model of human serum albumin (HSA) and experimentally determined association constants (Ka) for the interactions of these drugs with the protein. *: 6-methoxy-2-naphthylacetic acid
Drug | Ka (M-1) | Log Ka | Predicted affinity (kcal/mol) |
---|---|---|---|
Azapropazone | 280000 | 5.45 | -8 |
Bucolome | 1500000 | 6.17 | -7.2 |
Furosemide | 200000 | 5.30 | -7.4 |
Indomethacin | 1400000 | 6.15 | -7.1 |
Iodipamide | 9900000 | 6.99 | -6.2 |
Iophenoxic_acid | 77000000 | 7.89 | -5 |
Phenylbutazone | 1500000 | 6.18 | -7.3 |
Sulfisoxazole | 180000 | 5.26 | -7.3 |
Tolbutamide | 40000 | 4.60 | -7.6 |
Warfarin | 340000 | 5.53 | -7.9 |
6-MNA* | 1200000 | 6.08 | -7 |
Clofibrate | 760000 | 5.88 | -6.2 |
Diazepam | 1300000 | 6.11 | -6.9 |
Diclofenac | 3800000 | 6.58 | -6.6 |
Diflunisal | 530000 | 5.72 | -7.7 |
Etodolac | 200000 | 5.30 | -6.8 |
Ibuprofen | 3500000 | 6.54 | -6.6 |
Iopanoic_acid | 6700000 | 6.83 | -5.5 |
Ketoprofen | 2500000 | 6.39 | -7.1 |
Naproxen | 1200000 | 6.08 | -7.4 |
The level of correlation of predicted energy binding and experimentally- determined Ka yielded by the protocol in which AutoDock Vina was incorporated was high.
The docking protocol that set the sampling to exhaustiveness values of 100 resulted in a correlation coefficient of 0.6136 (Figure 8).

Fig. 8. Correlation between predicted affinities with experimentally determined association constants (Ka) for drug-humans serum albumin (HSA) interactions. Predicted affinity was estimated by docking drugs into the refined model of HSA using AutoDock Vina
Correlation studies showed that our protocol incorporating AutoDock Vina, with the parameters described, was capable of distinguishing quantitatively strong binders from weak binders, i.e. correlated with predicted affinity over a 1000-fold range in Ka values (Table 2). Therefore, the predicted affinity could be regarded as an index of experimental binding affinity.
4 Discussion
A crucial step in the development of new and effective drugs is the understanding of their ability to bind human serum albumin (HSA), as this protein plays a key role in the Absorption, Distribution, Metabolism, Excretion, and Toxicity, ADMET, of drug molecules, which ultimately determines their biosafety and pharmacokinetics profile. Spectroscopic studies such as fluorescence quenching have successfully been utilized to determine the association constants of drug with HSA. However, these techniques are costly, time consuming, and, fundamentally, they lack the capacity of providing a mechanistic interpretation of the interaction at the molecular level.
In recent years, the meteoric rise in computing power has been harnessed trying to streamline the drug discovery and development process by means of significantly reducing the need of chemical and biological resources, and by filtering or eliminating molecules with undesirable ADMET properties to select the most promising candidates [24].
The availability of the X-ray crystal structure of HSA (PDB ID 2BXH) [15] allowed us to develop a computer protocol in an attempt to characterize, at the molecular level, the interactions of drugs with this protein. Although particularly this X-crystal structure of HSA deposited in the Protein Data Bank possessed a relatively high resolution (2.25 Å), it is very common that atoms, side chains, or even whole residues are not included in the protein structure.
Furthermore, atomic clashes, overall inconsistencies in the folding pattern, and high potential energies associated to their geometries are common problems in most X-ray structures. To address these issues, and previous to perform molecular docking simulations, we subjected the X-ray model of HSA to a phase of thorough structural refinement using the most advanced computational techniques (see Methods section). We obtained a highly refined structure that represented the typical geometry of the HSA and which has been assumed to correspond to the physiologically relevant state.
The structure of HSA revealed the presence of three homologous domains (I, II and III), which consequently showed similar topology and 3D architecture. Each of the three domains is further divided into subdomains (A and B). The result of various internal and global geometry evaluations, to which the refined model of HSA was subjected, demonstrated its high geometric consistency and robustness.
The finding that 98.9% of the residues of the refined HSA model were located within most favored areas of the Ramachandran plot suggested excellent stereochemical quality. Also, the outcome of the analysis of non-bonded residue interactions yielded an ERRAT score of 94.2%, which is well above the lower limit (85%) accepted for high-quality structures. Altogether, these tests demonstrated the high structural quality of the refined model of HSA.
Although many studies have successfully conducted docking studies in which the search space spans the whole protein receptor [53, 19, 20], to reduce the computational cost, we restricted the search space the regions of HSA reported to be active in the binding of drugs. In all molecular docking simulations carried out in this study, two search spaces were defined in such a way that they covered completely the regions reported to encompass drug-binding site 1 and drug-binding site 2 [15]. Accordingly, drugs were docked into their reported binding site.
Firstly, we attempted to evaluate if AutoDock Vina predicted correctly the binding position of indoxyl sulfate in the experimental crystal structure of HSA (PDB ID 2BXH). This docking program predicted accurately the mode of binding of indoxyl sulfate within the crystal structure and, interestingly, the lowest energy conformations corresponded to the lowest RMSD values. This result suggested that AutoDock Vina could identify reliably the correct pose of a ligand within a receptor and thus may prove useful in molecular docking studies aimed at the characterization of the mechanism of drug-protein interaction.
Spectroscopy fluorescence quenching studies have reported the affinity of a series of drugs towards human serum albumin in terms of their association constants (Ka) [51, 52]. To investigate if there is a correlation between predicted affinities (free energy of binding) computationally calculated and experimentally determined Ka values, a set of 20 drugs were docked into the refined model of HSA.
Our computational studies showed that AutoDock Vina possessed a high capacity to correlate predicted affinities with in vitro experimentally calculated association constants. Interestingly, this capacity augmented as the “exhaustiveness” parameter was increased (data not shown). The correlation coefficient reached a maximum of 0.61 when the “exhaustiveness” was set to 100. Similar studies (Table 3), including QSAR (quantitative structure-activity relationships) and protein-protein-docking, have suggested that a coefficient correlation of 0.6, or better, ensures structural and functional validity of models.
Table 3 The correlation coefficient values for experimental and predicted binding affinities using in silico tools, including molecular docking and protein-protein docking techniques
The promiscuity of HSA to bind a wide range of drugs is a crucial feature of its biological function. The interaction between small molecules and proteins is usually characterized by the value of free energy of binding [26, 2]. The free energy is the thermodynamic quantity directly related to the experimentally measurable value of affinity constant (e.g. Ka). Therefore, these results suggested that predicted affinity by AutoDock Vina could be considered as an index of the experimental binding affinity (i.e. strength of non-covalent drug-HSA interaction).
Our findings showed consistency between computationally predicted molecular interactions with in vitro reported data, thereby confirming the stereochemical robustness, functional validity of the model and a reliable molecular docking protocol. Therefore, our computational protocol may be useful in the conduct of molecular modelling studies and to gain a better understanding of the molecular mechanism of interaction of HSA with drugs.
5 Conclusions
Despite the enormous advancements in the development of new drugs, bringing a new drug product to the market is still extremely expensive and time consuming. Interactions of drugs and drug candidates with human serum albumin have attracted great interest in the biomedical sciences. The nature and magnitude of these interactions are key determinants to the safety and efficacy of drugs and therefore they should be considered in the design process. Although in vitro protocols incorporating spectroscopic methods have historically been used to evaluate such interactions, they are economically demanding and also time intensive. Moreover, importantly, spectroscopic methods frequently lack the atomic resolution needed to provide a rational and mechanistic interpretation to the interactions at the molecular level. Therefore, there still remains a pressing need to incorporate new technologies to speed up the evaluation of drug-human serum albumin interactions, and thus to potentially streamline the drug discovery process.
The computer-based method described here incorporated a molecular docking approach to investigate structural aspects of the interactions of human serum albumin with a diverse set of drugs. Using state-of-the-art computational techniques, an exhaustive refinement protocol was applied to obtain a high quality, three-dimensional structure of human serum albumin. The refined structure was used to study drug-human serum albumin interactions using the docking program AutoDock Vina. Our docking protocol, in re-docking experiments, was capable of reproducing accurately the positioning of a co-crystallized ligand within the binding site of the X-crystal structure of human serum albumin. In addition, our protocol was capable of correlating, with high accuracy, predicted affinities (free energy of binding) with experimentally determined association constants.
We believe based on this study, that our computational-based approach incorporating AutoDock Vina may prove useful in understanding the interactions of human serum albumin with drugs. This may contribute to streamline the drug development process.