1. Introduction
Photovoltaic devices are one of the most important technologies for renewable, clean, and low-cost energy generation. Perovskite-based cells have emerged as a promising, low-cost alternative with higher efficiencies among the different photovoltaic device technologies. In the last decade, Perovskite solar cells have attracted the attention of materials science researchers, and significant advances in terms of efficiency have been observed. In 2009, Perovskite cells reported efficiencies of around 3.8%; since then, this has grown to 25.5% (Best Research-Cell Efficiency Chart, n.d.), surpassing even the performance of consolidated photovoltaic technologies such as those based on CdTe, CIG, and even polycrystalline silicon. These crucial advances suggest a role for Perovskite cells in the future of the PV industry. Despite the significant advances in this area, numerous contributions are still being made. They are reflected in many publications per year, which contain valuable information and data that can be used to develop new materials or structures of Perovskite-based solar cells. As researchers in this area generate data, new approaches are open for discovering and designing materials with improved properties using data-driven methods for knowledge discovery (machine learning) (Odabaşı et al., 2019; Yılmaz & Yıldırım, 2021; Zhou et al., 2019).
Machine learning is an increasingly explored alternative in materials science for developing Perovskite-based solar cells. But exploiting this wealth of latest information requires collecting data for training and evaluating the proposed models. These data may come from experimental results or theoretical calculations. The generation of theoretical data does not consider experimental factors inherent to the synthesis processes of thin films; therefore, experimental information can be considered the most convenient source of data for the generation of models. Previous studies using machine learning to predict the performance of solar cells have used data from theoretical calculations (Balachandran, Kowalski, et al., 2018; Gladkikh et al., 2020; Takahashi et al., 2018) and from experimental studies (Lu et al., 2018, 2019; Odabaşı et al., 2019; Stanley & Gagliardi, 2019; Wu & Wang, 2019; Yu et al., 2019). However, collecting experimental data is costly unless we take such data from information available in academic literature.
Recently, machine learning tools have been used to estimate and predict the bandgap of the absorber layer (Chaube et al., 2020; Gladkikh et al., 2020); to search for new photovoltaic Perovskites (Balachandran, Emery et al., 2018; Lu et al., 2019; Pilania et al., 2016; Takahashi et al., 2018; Zhang et al., 2020); and, to find trends in the way different cell layer composites are grouped concerning the efficiency value (Li et al., 2019; Odabaşı et al., 2019; Xu et al., 2018). In these reported works, descriptors such as the types of compounds used in the different layers of the cell, the different methods used for the synthesis of the layers, and the annealing times and temperatures have been used. Another descriptor that can be particularly useful in prediction models corresponds to the thickness of the absorbing layer, which, having an inverse relationship with the absorption coefficient, determines to a significant extent, the capacity of a material to absorb photons and generate electrons. However, in previous works, the thickness of the absorbing layer as an input parameter has yet to be reported (Balachandran, Emery et al., 2018; Chaube et al., 2020; Gladkikh et al., 2020; Li et al., 2019; Lu et al., 2019; Odabaşı et al., 2019; Pilania et al., 2016; Takahashi et al., 2018; Velez Sanchez et al., 2022; Zhang et al., 2020).
The present work analyses (from a statistical point of view) the relationship between the thickness of the absorber layer and the main performance characteristics of Perovskite solar cells. In particular, the mutual information measure is employed to quantify the degree of nonlinear statistical relationship between the descriptors and the performance values of the cell. Then, we quantify the degree of contribution of the thickness in the decrease of the estimation error for predicting the electrical characteristics of Perovskite solar cells.
2. Method
Through the method described below, the impact of the thickness of the absorber layer on the estimation of the main electrical characteristics of Perovskite cells is analyzed. For this purpose, the automatic learning approach uses mutual information and multiple linear regression statistical analysis tools. The multiple linear regression was performed using cross-validation, for which the data were divided as follows: 90% of the data was for training and the remaining 10% for validation. The validation was performed successively until all the data were considered for training and validation, giving ten iterations for the multiple linear regression with different input data.
2.1. Data
In this work, we take the data reported and used in the supplementary information of (Li et al., 2019) as a source of information, whose database consists of 333 records of values taken from research articles. The descriptors used are the composition of the absorbing layer, the bandgap, the difference between the highest energy-occupied molecular orbitals (HOMO) of the HTL layer and the absorbing layer (∆HOMO), and the difference between the lowest energy-unoccupied molecular orbitals (LUMO) of the absorbing layer and the ETL layer (∆LUMO). Also, electrical characteristics are included, the open-circuit voltage Voc, the short-circuit current density Jsc and the fill factor FF. To analyze the relevance of thickness, this value was manually extracted from each scientific article. In those cases where this value was not reported, the authors were contacted directly via e-mail. In total, 221 thicknesses were obtained, so our dataset is limited to this number. This dataset is published in (Velez Sanchez et al., 2022).
2.2. Descriptors
Selecting descriptors is a task of significant importance when applying automatic learning methods. Descriptors must have an implicit meaning and be available, which requires that they be easily reportable and universally reported by authors in scientific papers to be considered. Ideally, the number of descriptors should be reduced to avoid over-fitting problems; and not contain redundant information. However, guaranteeing full compliance with the above conditions is difficult. Recent work has used variables such as bandgap, ∆HOMO, and ∆LUMO to represent the information from which cell characteristics could be predicted (Li et al., 2019; Odabaşı et al., 2019). In addition, in works such as those presented by Gladkikh et al. (2020), Pilania et al. (2016), Takahashi et al. (2018), Zhang et al. (2020), characteristics such as electronegativity, the number of atomic orbitals, the Goldschmidt tolerance factor of the elements that make up the absorbing layer are used to perform prediction or classification tasks in machine learning algorithms. However, no works were found in which the thickness of the absorbing layer is included as a descriptor. In the present work, in addition to the variables already mentioned in previous works, it is desired to use the thickness of the absorbing layer as an input characteristic of the model in charge of estimating the performance in the prediction of output electrical variables such as the short circuit voltage Voc, short circuit current Jsc, the filling factor FF and PCE. In addition to the inclusion of the thickness, a modification was made to the database used, which consisted of changing the coding for the compounds that form the Perovskite, which went from 8 to three variables that were as follows: A = MA - FA - Cs, which groups the compounds used in the Perovskite cation A, B = Pb - Sn and X = I - Br - Cl. This modification was made to make the models used in the linear regression more flexible, thus lowering the complexity of the final model and reducing the effects of over-fitting on the results obtained.
2.3. Mutual information as a measure of nonlinear statistical association
The mutual information ́on between two random variables x, y, denoted as I(x, y) measures the mutual dependence between the two variables; that is, it quantifies the amount of information obtained from one random variable through the observation of the other random variable (Bishop, 2006).
Where I(, y) ≥ 0; and I(x, y) = 0 for the case where x, y are independent variables. The units in which this measure is expressed depend on the type of logarithm; in particular, if the logarithm is in base two, the mutual information is in units of bits. There are several methods for estimating I(-, -); however, in the present work, the k-neighbors-based method, reported in (Kraskov et al., 2004; Ross, 2014), is used. I(-, -) is calculated between each descriptor
2.4. Multiple linear regression
The regression function is assumed to be linear for the inputs in multiple linear regression. In our case for
Furthermore, in a second model, if the thickness is included.
Where
3. Results
3.1. Estimation of the degree of statistical-linear association of descriptors
Table (1) reports the estimated bitwise values of the statistical association I(-, -) for different pairs of descriptors vs electrical characteristics. This value was calculated for each pair of variables and 20 different subsets of 177 samples out of 221 (80% of the total), where samples were randomly selected without replacement. Each value reported in Table (1) results from the average of the 20 values obtained for each of the 20 subsets. This strategy allowed us to estimate the standard error associated with each of the estimates and thus develop a t-student hypothesis test to determine whether the estimated values are statistically different from zero. That is, to determine if statistically there is an association. As a result, it is obtained that all except 2 are statistically different from zero. The results show that the absorbing layer's thickness helps explain the behaviour of the Jsc inside the cell. They also allow us to infer that the results obtained for B, formed by B = Pb - Cs, are a product of a large amount of data in which B is lead. Because of this, the metric used cannot perceive the importance of B within the linear regression models.
Table 1 The rows correspond to film descriptors, and the columns to performance indices. The six most significant values (≥ 0.200) are shown in bold.
PCE | Voc | Jsc | FF | |
---|---|---|---|---|
A | 0.241 | 0.327 | 0.172 | 0.016 |
B | 0.0 | 0.042 | 0.038 | 0.0 |
X | 0.340 | 0.262 | 0.157 | 0.060 |
Eg | 0.071 | 0.238 | 0.157 | 0.069 |
ΔHOMO | 0.054 | 0.160 | 0.066 | 0.052 |
ΔLUMO | 0.081 | 0.051 | 0.111 | 0.022 |
Thickness | 0.067 | 0.139 | 0.264 | 0.026 |
From the results obtained and summarized in Table (1), the following behaviors are observed, among others: i) thickness as a descriptor plays a significant role in describing the behavior of Jsc, being this the descriptor with the most significant contribution to this electrical characteristic. ii) Under this same working model, descriptors A, X and Eg are the ones that have the most significant contribution to Voc. iii) A comparable situation is found for efficiency (PCE), which presents a contribution from descriptors A and X. These results are consistent with the physical explanation of the phenomenon of photoconversion of radiation into electrical energy in a photovoltaic device. The thickness of the Perovskite layer, playing an indispensable role in photogeneration, influences the generation rate G described in the models reported in the literature (Le Corre et al., 2019). Similarly, the thickness of this absorbing layer affects the transport process of photogenerated carriers to the selective transport layers (ETL and HTL). Hence, as a parameter, it influences the carrier recombination rate (Le Corre et al., 2019). Likewise, Voc and PCE as electrical characteristics are strongly affected by the Eg of the absorber layer (Jarosz et al., 2020), which in turn depends strongly on the chemical composition of the Perovskite, which is described by A, B, and X (Kato et al., 2017).
3.2. Estimation of the performance values
Figure (1) shows the comparison graphs of the descriptor
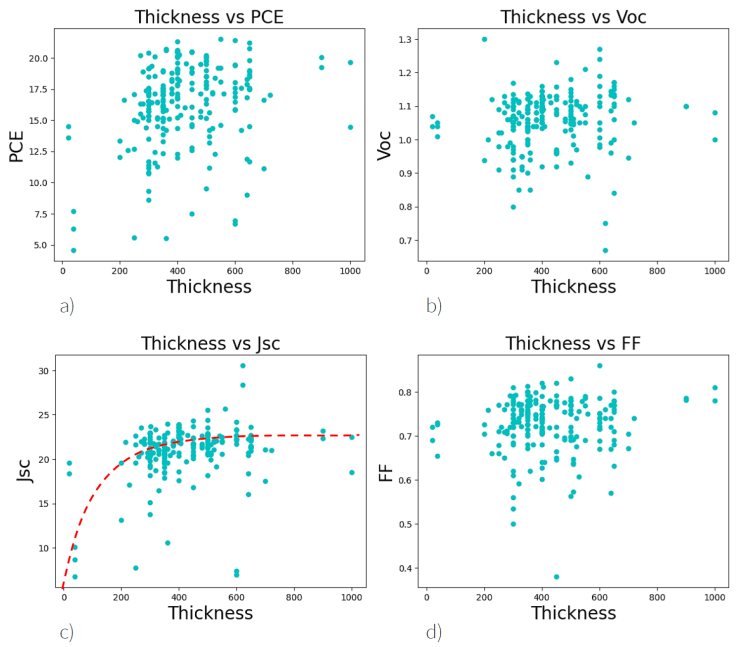
Figure 1 Example of scatter plots of some inputs versus some outputs. a) Thickness vs PCE, b) thickness vs Voc, c) thickness vs Jsc, d) thickness vs FF.
The Jsc, Voc, FF, and PCE characteristics were estimated using multiple linear regression. For each electrical variable to be estimated, two models were applied by selecting those described in equations 2 and 3 belonging to the Z and
Table 2 Estimation results of Jsc, Voc, FF, and PCE using linear regression. We also report the improvement in performance by including the squared root of thickness √δ as a descriptor. The value in parenthesis corresponds to the standard deviation of the measurement.
On the other hand, to determine whether the differences in performance in terms of RMSE are statistically significant, a two-sample t-student test is applied. The results show that the inclusion of the thickness of the absorbing layer (√δ) as a descriptor improves the prediction of the Jsc and PCE values. The thickness information, transformed in the √δ form, provides relevant information for Jsc and PCE estimation purposes. As a result, it is found that for the case of Jsc and PCE, the improvement in performance by adding the thickness.
The Jsc, Voc, FF, and PCE characteristics were estimated using multiple linear regression. For each electrical variable to be estimated, two models were applied by selecting those described in equations 2 and 3 belonging to the Z and
On the other hand, to determine whether the differences in performance in terms of RMSE are statistically significant, a two-sample t-student test is applied. The results show that the inclusion of the thickness of the absorbing layer (√δ) as a descriptor improves the prediction of the Jsc and PCE values. The thickness information, transformed in the √ δ form, provides relevant information for Jsc and PCE estimation purposes. As a result, it is found that for the case of Jsc and PCE, the improvement in performance by adding the thickness.
To visualize this improvement, we have compared the predictions obtained vs the real values for the four models. Figure 2 shows the behaviors of the estimated values. Of these four models, Jsc's prediction is the best performing.
4. Conclusions
The results obtained show that the thickness of the absorbing layer is a relevant variable in predicting electrical measures of performance. It could be inferred that the thickness is related to electrical variables, especially with the Jsc, for which higher improvement percentages were observed. An explanation for this fact is that the thickness of the absorbing layer influences the process of photogeneration and carrier transport. On the other hand, these results show that the inclusion of new features, such as thickness, can positively influence the models used in machine learning tasks. Unfortunately, it requires the scientific community to report these parameters in research articles.
On the other hand, extracting new variables from the available scientific literature is a time-consuming task that could be improved through natural language processing tools or by standardizing the reporting formats of solar cell parameters published in academic reports.