Introduction
Among the most unique ecosystems in the Neotropics is cloud forest (Rzedowski, 1996; Williams-Linera, 2002). In Mexico, this forest holds more than 10% of the country's plant species, with high levels of endemism (Rzedowski, 1996). According to González-Espinosa (2011), more than 82% of the country's plant families are found in this ecosystem. Cloud forest is also the most endangered ecosystem in Mexico, in view of its small extent (about 1% of the total area of the country) (González-Espinosa, 2011; González-Espinosa et al., 2012). The ecological complexity of cloud forest is related to its restricted elevational range and narrow intervals of thermal and humidity conditions. These uncommon factors make cloud forest an archipelago in the highlands that may be particularly vulnerable to climate change (Foster, 2001; Gasner et al., 2010; Holder, 2004).
Cloud forest, according to Rzedowski (1996), is characterized by the persistence, frequency, and seasonality of the cloud layer at vegetation height (Aldrich et al., 1997; Foster, 2001; Gual-Díaz & Rendón-Correa, 2014). This linkage with regular cloud formation cycles provides effective fluvial precipitation, high humidity, and reduced solar radiation, as well as low rates of evapotranspiration and evaporation (Gual-Díaz & Rendón-Correa, 2014; Still et al., 1999). Cloud forests in Mexico represent a transitional ecosystem between lowland rain forest and temperate forests (Toledo-Aceves, 2010; Villaseñor, 2010), and have a complex biogeographic history (Figueroa-Rangel et al., 2009).
Cloud forests are under strong pressures from human activities, mainly in the form of conversion to agriculture and fragmentation (Moguel & Toledo, 1999; Pineda et al., 2005; Ramírez-Marcial et al., 2001; Williams-Linera, 2002). However, other threats like global climate change may also pose problems for this ecosystem (Correa-Ayram et al., 2017; Gasner et al., 2010; Rehm & Feeley, 2015; Rojas-Soto et al., 2012; Still et al., 1999). These changes could reduce the biological diversity, and quality of ecosystem services provided by cloud forest (Alvarez-Arteaga et al., 2013; Martínez et al., 2009; Ponce-Reyes et al., 2013; Rehm & Feeley, 2015; Toledo-Aceves et al.,2014).
Ecological niche models (ENMs) have been used frequently to estimate current distributions of species and to anticipate effects of climate change on species' distributions in many ecosystems (Aguilera et al., 2013; Ashraf et al., 2017; Banag et al., 2015; Carroll et al., 2011; Chatterjee et al., 2012; Kearney et al., 2010), including cloud forest (Contreras-Medina et al., 2010; Cruz-Cárdenas et al., 2014; Golicher et al., 2012; Gómez-Mendoza & Arriaga, 2007; López-Mata et al., 2012; Monterroso-Rivas et al., 2013; Ponce-Reyes et al., 2012; Rojas-Soto et al., 2012; Téllez-Valdés et al., 2006; Vega et al., 2000). They also can inform about which climatic factors constrain distributions of species, and how these factors will change into the future (Luna-Vega et al., 2012). Ecological niche models, properly implemented, are presently considered to constitute the best tool with which to assess effects of climate change on distributions of species (Martínez-Meyer, 2005).
This paper assesses how climate change will likely affect tree species richness and composition in Mexican cloud forests. This work is developed under the assumption that climate is an important determinant of species diversity in each cloud forest patch, not the only factor, but one of the most important. We use ENM approaches to assess likely distributional shifts in 12 species of cloud forest tree species, under 2 greenhouse gas emissions scenarios and 20 general circulation models (GCMs; these models provide a predictive view of likely future climate conditions). The outcome is a predictive view of changes in cloud forest tree species composition that can be expected across the range of this ecosystem (with emphasis in eastern Mexico) as a consequence of global climate change.
Material and methods
According to Rzedowski (2006), less than 1% of the surface area of Mexico is covered or was covered originally by cloud forest (INEGI, 2015). Mexican cloud forests (Fig. 1) are located mainly in the Sierra Madre Oriental (Gulf of Mexico influence: patches 1, 2, 3, 4, 5, 6, and 7), and to a lesser extent in the Sierra Madre Occidental (Pacific influence: patches 13, 14, 15, 16, 17, 18, and 19); some patches are located in the Sierra Madre del Sur (Pacific influence: patches 8, 9, 10, 11, and 12) and the Transverse Volcanic Belt (central Mexico: patches 20, 21, and 22).
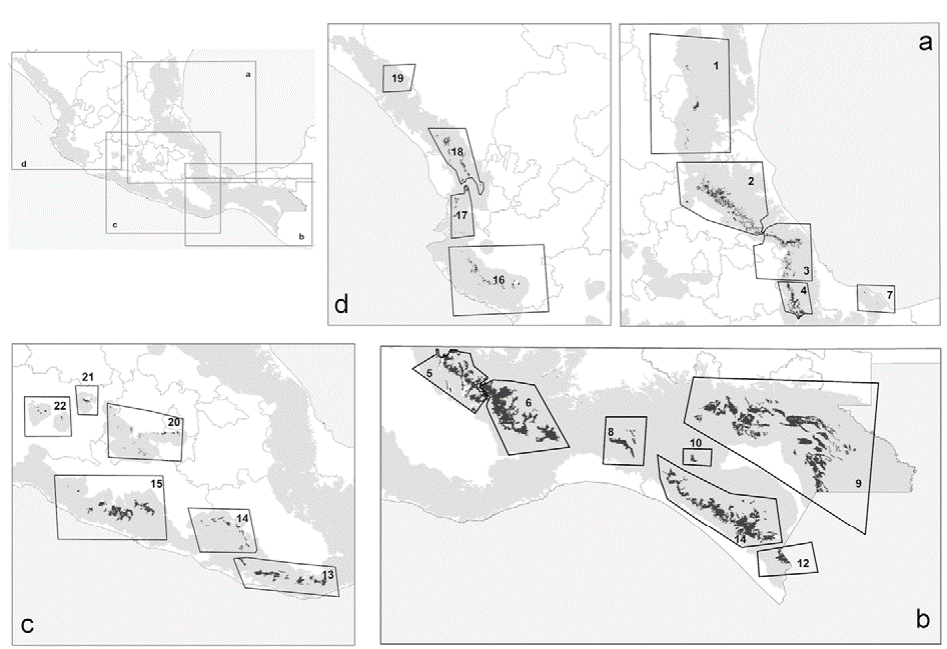
Figure 1 Distribution of cloud forest patches in Mexico and groupings used in our analyses. (a) Sierra Madre Oriental (Gulf influence), (b) Sierra Madre del Sur, (c) central Mexico, and (d) Sierra Madre Occidental (Pacific influence). Calibration area is shown in light gray.
Under the idea that ENMs should be calibrated across the area that has been accessible to the species in question over relevant time periods (Barve et al., 2011; Owens et al., 2013; Peterson et al., 2011), we determine our calibration area with the COSTGROW module in TerrSet (Eastman, 2016), to generate a surface that reflects effort necessary to access adjacent regions. Specifically, we used a digital elevation model (DEM) to summarize friction or resistance to colonization; this raster data layer was reclassified, such that lowlands (0 - 50 m elevation) were prohibited, and the remaining elevations guided estimates of effort necessary to increase the species' range (i.e., low and very high elevations related to high effort to colonize). This module works with a maximum growth distance specified in cost units (buffer); we explored distances of 1 - 5 km. We chose 3.2 km because it yielded an area that approximated the historical known distribution of cloud forest (Graham, 1999; Martin & Harrell, 1957). All spatial analyses were developed using ArcGIS 10.3 and its extension SDMTools (Brown, 2014).
We focused on threatened species (Table 1, Fig. 2) in cloud forest under higher IUCN threat categories: Critical or Endangered (González-Espinosa, 2011; Rodríguez et al., 2011). Occurrence data were obtained from the Global Biodiversity Information Facility (GBIF; https://www.gbif.org/), Red Mundial de Información sobre Biodiversidad (REMIB; http://www.conabio.gob.mx/remib/doctos/remibnodosdb.html), and Herbario Nacional del Instituto de Biología, UNAM (MEXU; http://datosabiertos.unam.mx/biodiversidad/). Most of the occurrences (80%) were from the Sierra Madre Oriental (Veracruz, Puebla, Hidalgo, and Oaxaca), collected between 1972 and 2006, though we include data from across Mexico. Biasing effects of spatial autocorrelation in occurrence data were reduced with SDMTools (Brown, 2014), using a distance filter of 4.5 km, which corresponded roughly to the spatial precision of the occurrence data. We set aside a random 40% of available data for each species for model evaluation (see below).
Table 1 Species, number of records, and IUCN category used in this study. We use the Pearson et al. (2006) method for species with < 25 records.
Family | Species | # records | IUCN category |
Araliaceae | Oreopanax flaccidus | 20 | CR A4c |
Caprifoliaceae | Viburnum ciliatum | 15 | EN B1AB |
Fagaceae | Quercus germana | 32 | CR A4acd |
Quercus sartorii | 62 | EN A2C | |
Quercus xalapensis | 9 | CR A2C | |
Lauraceae | Cinnamomum effusum | 54 | EN B1ab(iii) |
Ocotea klotzschiana | 41 | EN B1ab(iii) | |
Ocotea psychotrioides | 53 | EN B1ab(iii) | |
Persea longipes | 14 | EN B1AB | |
Simaroubaceae | Picramnia xalapensis | 36 | EN A4c |
Symplocaceae | Symplocos coccinea | 29 | EN A4c; B1ab(iii) |
Theaceae | Ternstroemia huasteca | 20 | EN B1ab(iii) |

Figure 2 Occurrence data used in development of ecological niche models in this study for 12 endangered tree species.
We used 19 bioclimatic variables from WorldClim in model calibration (Hijmans et al., 2005); these variables were derived from monthly averages of temperature and precipitation over the period 1950-2000. For future conditions, we used data from 20 general circulation models obtained from Climate Change, Agriculture and Food Security (CCAFS) downscaled general circulation model (GCM) data portal (http://www.ccafs-climate.org/data_spatial_downscaling/), for 2 emissions scenarios (RCP 4.5 and 8.5) for 2050 (Table 2). All analyses were performed at a spatial resolution of 2.5'. To reduce dimensionality, we used Spearman rank correlations based on 10,000 random points inside the calibration area (Fig. 1), removing one of each pair of variables presenting correlation coefficients above 0.80, using routines in Statistica V.8.0. Variables used in final models (i.e., after variable reduction) were precipitation seasonality, isothermality, temperature seasonality, mean diurnal temperature range, and temperature annual range.
Table 2 General circulation models used in ENM projections in RCP 4.5 and RCP 8.5
General circulation model acronym | Institution |
bnu_esm | Beijing Normal University Earth System Model |
cesm1_bgc | NSF-DOE-NCAR |
cesm1_cam5 | NSF-DOE-NCAR |
csiro_access1_3 | Commonwealth Scientific and Industrial Research Organization (CSIRO) and Bureau of Meteorology |
(BOM), Australia | |
csiro_access1 | Commonwealth Scientific and Industrial Research Organization (CSIRO) and Bureau of Meteorology |
(BOM), Australia | |
gfdl_cm3 | NOAA Geophysical Fluid Dynamics Laboratory |
gfdl_esm2g | NOAA Geophysical Fluid Dynamics Laboratory |
gfdl_esm2m | NOAA Geophysical Fluid Dynamics Laboratory |
giss_e2_h_cc | NASA Goddard Institute for Space Studies USA |
giss_e2_r | NASA Goddard Institute for Space Studies USA |
inm_cm4 | Russian Institute for Numerical Mathematics |
miroc_esm | University of Tokyo, National Institute for Environmental Studies, and Japan Agency for Marine-Earth |
Science and Technology | |
miroc_esm_chem | University of Tokyo, National Institute for Environmental Studies, and Japan Agency for Marine-Earth |
Science and Technology | |
miroc_miroc5 | University of Tokyo, National Institute for Environmental Studies, and Japan Agency for Marine-Earth |
Science and Technology | |
mohc_hadgem2_cc | UK Met Office Hadley Centre |
mohc_hadgem2_es | UK Met Office Hadley Centre |
mri_cgcm3 | Meteorological Research Institute |
ncar_ccsm4 | US National Centre for Atmospheric Research |
ncc_noresm1_m | Norwegian Climate Centre |
nimr_hadgem2 | UK Met Office Hadley Centre |
When initial model evaluations were unsuccessful (see below) using the full data set, we further reduced the number of variables to only the first 3 in the list above (Table 1).
ENMs were calibrated with Maxent 3.3.3k (Phillips et al., 2006). We used a combination of model selection approaches (Warren & Seifert, 2011), significance testing, and performance testing to choose optimal parameter settings for each species. In model selection, we tested regularization multiplier values of 0.1, 0.3, 0.5, 0.7, 1, 2, 3, 5, 7, and 10, and response types including linear, quadratic, threshold, hinge, and product.
We used the Akaike information criterion with correction for small sample size (AICc) for model selection (Aho et al., 2014; Warren, & Seifert, 2011) for species with >25 occurrences; we used ENMTools version 1.4.4 (Warren et al., 2010) for AICc calculations. Partial ROC was used to evaluate model predictions, given strong concerns about the use of typical ROC approaches (Lobo et al., 2008; Peterson et al., 2008; Somodi et al., 2017). In particular, partial ROC considers only user-defined acceptable ranges of omission errors (here, up to a maximum of E = 5%). Partial ROC tests were executed in NicheToolBox (http://shiny.conabio.gob.mx:3838/nichetoolb2/), based on Peterson et al. (2008), with code developed by Osorio-Olvera et al. (2016). Probability values were determined by direct count of AUC ratios < 1.0 among 500 replicate 50% bootstrap resampling iterations. Model evaluations for species with low sample sizes (n < 25) were achieved via methods proposed and code provided by Pearson et al. (2006), using a jackknife-based approach modified from the cumulative binomial.
Models were calibrated under present-day conditions in Maxent with bootstrap subsampling and 10 random replicates. Models were then transferred to future climate scenarios (Table 3), and median outputs used to summarize outputs across the different future scenarios. For thresholding, we used a modified version of least training presence threshold, under an acceptable omission rate of E = 5% (calibration data) to create binary (presence-absence) maps from which we calculated omission rates (independent testing data). Our model selection approaches, which included dimensions of (1) statistical significance, (2) performance in terms of avoiding high omission error, and (3) model simplicity and avoidance of overfitting, should avoid or reduce many common criticisms of ENM approaches as regards underfitting or overfitting (Araújo & Peterson, 2012).
Table 3 Models selected for each of 12 cloud forest species in Mexico, providing regularization parameter values, feature, likelihood, number of parameters in the model, AICc values, AUC ratio, presence threshold, and omission rates. Entries for Cinnamomum effusum* include averages from 3 different models.
Family | Species | Regularization parameter | Feature | Log-likelihood | Parameters | AICc score | AUC ratio | Presence threshold | Omission rates |
Araliaceae | Oreopanax flaccidus | 0.3 | Quadratic | -223.5 | 4 | 457.9 | 0.2527 | 33.3 | |
Caprifoliaceae | Viburnum ciliatum | 0.1 | Quadratic | -136.9 | 5 | 293.8 | 0.101 | 16.7 | |
Fagaceae | Quercus germana | 3 | Hinge | -374.6 | 6 | 764.8 | 1.56 | 0.4922 | 0.0 |
Quercus sartorii | 2 | Hinge | -726.5 | 16 | 1497.4 | 1.13 | 0.2735 | 25.0 | |
Quercus xalapensis | 2 | Linear | -100.3 | 1 | 203.2 | 0.4842 | 12.5 | ||
Lauraceae | Cinnamomum effusum* | 1, 2, 2 | Threshold | -38.8 | 13, 4, 5 | 1262.1 | 1.33 | 0.2564 | 0.0 |
Ocotea klotzschiana | 1 | Hinge | -463.2 | 14 | 971.3 | 1.16 | 0.0924 | 0.1 | |
Ocotea psychotrioides | 1 | Hinge | -581.3 | 18 | 1219.3 | 1.62 | 0.388 | 9.1 | |
Persea longipes | 7 | Quadratic | -452.2 | 2 | 908.8 | 0.5206 | 7.7 | ||
Simaroubaceae | Picramnia xalapensis | 1 | Threshold | -401.0 | 16 | 864.2 | 1.48 | 0.1200 | 0.0 |
Symplocaceae | Symplocos coccínea | 0.7 | Quadratic | -338.2 | 3 | 683.4 | 1.38 | 0.2982 | 18.2 |
Theaceae | Ternstroemia huasteca | 0.1 | Quadratic | -220.7 | 5 | 456.0 | 0.3583 | 5.3 |
To summarize likely global climate change impacts on cloud forest tree species, we used the IUCN endangerment criteria (Rodríguez et al., 2011). Three general categories were used based on proportional change in range area as compared with the present: a) species loss, in which species lose > 80% of present-day distributional area; b) range reduction, in which species lose 20-80% of present-day area; and c) stability or increase, in which species retain > 80% of present-day distributional area. Finally, we evaluated our model transfers using a MOP analysis (Owens et al., 2013) for each combination of GCM and RCP, to identify areas of extrapolation in model transfer.
Results
All models developed in this study resulted better than random expectations (all p < 0.005). For the 7 species with large sample sizes, partial ROC tests were uniformly better than null expectations (all p < 0.0002). For Quercus xalapensis, Persea longipes, Viburnum ciliatum, and Oreopanax flaccidus, for which sample sizes were lower, the Pearson small-sample test also indicated predictions better than random (p < 0.05), although in one case (Cinnamomum effusum), we had to simplify models to just 3 environmental layers.
Model selection based on AICc generally identified hinge, quadratic, and threshold response types as best. Quadratic response types were most common for small sample sizes (n < 25), except for Q. xalapensis, for which linear features were selected. AICc scores were lowest for species with small sample sizes, except for S. coccínea, probably because models with larger sample sizes generally incorporated more parameters. Regularization multiplier values were generally relatively high, which makes for relatively smooth and simple response surfaces (Table 1). The most important variables for models were related to seasonality (rather than absolute amounts) of precipitation and temperature.
Present-day predictions reflected an irregular and highly restricted distributional pattern for the 12 species within Mexican cloud forest (Fig. 3a). Southern, northwestern, and central Mexico all largely lacked habitable conditions for these species. Highest predicted species richness was in the mountains above the Mexican Gulf, in the states of Veracruz, Puebla, and Hidalgo. Lower diversity was along the Pacific Coast (of the species analyzed), where Ocotea klotzschiana, Q. sartorii, and Picramnia xalapensis had their only occurrences in our dataset. Species like O. flaccidus, Q. germana, and V. ciliatum had populations in both regions, increasing predicted species richness in the Pacific area. Another predicted hotspot for these species was in the Sierra Norte, in the northern part of the state of Oaxaca.
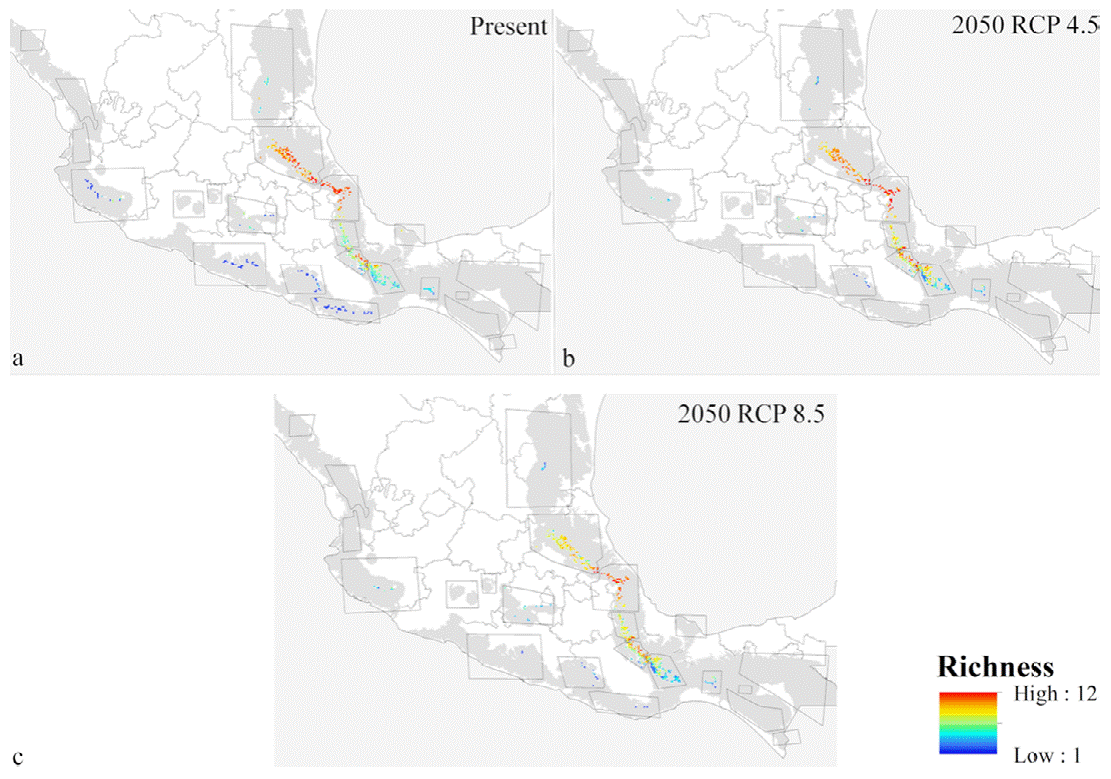
Figure 3 Alpha diversity predicted for 12 tree species in Mexican cloud forest, visualized within cloud forest patches only. Diversity (i.e., number of species) is shown for: (a) present, (b) RCP 4.5 in 2050, and (c) RCP 8.5 in 2050.
ENM transfers to future conditions under RCP 4.5 (Fig. 3) showed richness highest in the same regions of Puebla, Veracruz, and Hidalgo, but with lower total species richness predicted (Fig. 3a). Diversity was depressed most in patches 2, 4, and 5, (Gulf influence), and 8, 13, 15, and 16 (Pacific influence). Losses of species (Fig. 4b) were focused in patches 1, 2, 3, 5, and 6 (Gulf influence), and 20 (central Mexico). Eight patches had species losses for Cinnamomum effusum, O. flaccidus, O. klotzschiana, P. longipes, Picramnia xalapensis, and Q. sartorii (Table 4). Range reductions were predicted for O. flaccidus, O. klotzschiana, P. longipes, Q. germana, Q. sartorii, Q. xalapensis, and Symplocos coccinea. Thus, practically all the species under consideration showed losses or reductions somewhere in the region. However, stable status in patches or possible increases in distributional area were focused in the Gulf region (patches 2 and 3; Fig. 4c); in general, we observed stronger changes (both positive and negative) in the Gulf Coast-influenced patches 2, 3, 4, 5, and 6 (Fig. 4a, b).

Figure 4 Proportional changes in species' potential distributional areas in the face of climatic change under emissions scenario RCP 4.5, in terms of species lost or with ranges reduced. Red indicates species losses (a), gray indicates range reductions (b), and blue indicates range stability (c). Species abbreviations: Cinnamomum effusum (CE), Oreopanax flaccidus (OF), Ocotea klotzschiana (OK), Ocotea psychotrioides (OP), Persea longipes (PL), Pricamnia xalapensis (PX), Quercus germana (QG), Quercus sartorii (QS), Quercus xalapensis (QX), Symplocos coccinea (QC), Ternstroemia. huasteca (TH), and Viburnum ciliatum (VC).
Table 4 Species sensitivity to climate change in Mexican cloud forest, including number of species losses and range reductions. Hotspots refer to cloud forest patches 2, 3, 4, and 7, whereas general refers to all patches. Species are represented with the abbreviations: C. effusum (CE), O. flaccidus (OF), O. klotzschiana (OK), O. psychotrioides (OP), P. longipes (PL), P. xalapensis (PX), Q. germana (QG), Q. sartorii (QS), Q. xalapensis (QX), S. coccinea (QC), T. huasteca (TH), and V. ciliatum (VC).
Transition | RCP | Groups | CE | OF | OK | OP | PL | PX | QG | QS | QX | SC | TH | VC | Totals |
Species loss | 4.5 | General | 1 | 2 | 1 | 0 | 1 | 1 | 0 | 2 | 0 | 0 | 0 | 0 | 8 |
Hotspot | 0 | 1 | 0 | 0 | 1 | 0 | 0 | 0 | 0 | 0 | 0 | 0 | 2 | ||
8.5 | General | 2 | 0 | 1 | 0 | 1 | 1 | 0 | 1 | 1 | 1 | 0 | 0 | 8 | |
Hotspot | 0 | 0 | 0 | 0 | 1 | 0 | 0 | 0 | 1 | 1 | 0 | 0 | 3 | ||
Species range reduction | 4.5 | General | 0 | 1 | 1 | 0 | 1 | 0 | 1 | 2 | 1 | 1 | 0 | 0 | 8 |
Hotspot | 0 | 1 | 1 | 0 | 1 | 0 | 1 | 0 | 1 | 1 | 0 | 0 | 6 | ||
8.5 | General | 1 | 0 | 0 | 1 | 0 | 0 | 2 | 3 | 1 | 1 | 0 | 0 | 9 | |
Hotspot | 1 | 0 | 0 | 1 | 0 | 0 | 1 | 0 | 1 | 1 | 0 | 0 | 5 | ||
Total (loss + reduction) | 4.5 | General | 1 | 3 | 2 | 0 | 2 | 1 | 1 | 4 | 1 | 1 | 0 | 0 | 16 |
Hotspot | 0 | 2 | 1 | 0 | 2 | 0 | 1 | 0 | 1 | 1 | 0 | 0 | 8 | ||
8.5 | General | 3 | 0 | 1 | 1 | 1 | 1 | 2 | 4 | 2 | 2 | 0 | 0 | 17 | |
Hotspot | 1 | 0 | 0 | 1 | 1 | 0 | 1 | 0 | 2 | 2 | 0 | 0 | 8 |
Model transfers to RCP 8.5 showed stronger differences from the present than projections to RCP 4.5 (Table 4). The biggest differences in alpha diversity were in patches 2 and 3 (Fig. 3c), although we noted species losses and range reductions in patches on both Gulf and Pacific slopes. Under this scenario, 7 species were lost from patches 2, 8, 13, and 15 (Fig. 3a), whereas range reductions included 3 species in patch 2. Species affected at the level of loss from entire patches were C. effusum, O. klotzschiana, P. longipes, P. xalapensis, Q. sartorii, Q. xalapensis, and S. coccinea. Range reductions were in patches 1, 2, 3, and 6 on the Gulf side; 16 and 20 on the Pacific side; and in central Mexico (Fig. 5b). In contrast, some species (C. effusum, O. flaccidus, and O. klotzschiana) were predicted to maintain their distributional areas without notable changes or increases (Fig. 5c). Model transfers under both scenarios were evaluated for extrapolative conditions with a MOP analysis; however, we did not observe any differences in climatic conditions between present and the 20 GCM x 2 RCP future predictions that indicated situations of model extrapolation.

Figure 5 Proportional changes in species' potential distributional areas in the face of climatic change under emissions scenario RCP 8.5, in terms of species lost, reduced in range or stable in range. Red indicates species losses (a), gray indicates range reductions (b), and blue indicates range stability (c). Species abbreviations: Cinnamomum effusum (CE), Oreopanax flaccidus (OF), Ocotea klotzschiana (OK), Ocotea psychotrioides (OP), Persea longipes (PL), Pricamnia xalapensis (PX), Quercus germana (QG), Quercus sartorii (QS), Quercus xalapensis (QX), Symplocos coccinea (QC), Ternstroemia huasteca (TH), and Viburnum ciliatum (VC)
Discussion
Information available about cloud forest tree species is sparse, particularly regarding many of the endangered species (Rzedowski, 1996; Villaseñor, 2010). Cloud forest has an important vulnerability to different threats, particularly to land-use change (Martínez et al., 2009; Ramírez-Marcial et al., 2001) and global change (Figueroa-Rangel et al., 2009), at spatial scales ranging from local (Ledo et al., 2013; Rapp & Silman, 2012; Van Beusekom et al., 2017) to regional (Golicher et al., 2012; Rojas-Soto et al., 2012; Van Beusekom et al., 2017). Our work showed highest diversity of endangered tree species in Gulf region cloud forest (i.e., patches 2, 3, 5, and 6), where no protected areas are present (Fig. 6). Our patches 2 and 3 are the cloud forest areas considered to be the most diverse in the country (Delgadillo-Moya et al., 2017). Some protected areas are nearby, but none includes much cloud forest (Correa-Ayram et al., 2017; Ochoa-Ochoa et al., 2017). An important initiative of the Mexican government is to create a unique preserve area called the Sierra Madre Oriental Ecological Corridor (CESMO), but it is not a protected area per se, and a detailed management plan is lacking (Gillespie et al., 2012). More generally, as endangered species of cloud forest trees are not presently included inside any protected areas, mitigation strategies for climate change effects are compromised from the outset (Ponce- Reyes et al., 2012).

Figure 6 Mexican cloud forest patches included natural protected areas (we include local and communitarian conservation areas) based on actual cloud forest patch locations from the Instituto Nacional de Estadística, Geografía e Informática (INEGI), Serie V, and natural protected areas (ANP's) from the Comisión Nacional de Áreas Naturales Protegidas (Conanp).
The Mexican government has made concerted efforts to identify endangered species and protect them via the law NOM-059-2011-Semarnat. However, this effort does not include a clear strategy by which to preserve those species (Fig. 6), especially in endangered ecosystems like cloud forest. Our results indicated that the most important hotspots for the 12 tree species were patches 2 and 3, where 12 such species are likely co-distributed (Fig. 3); these cloud forest patches, in the states of Veracruz, Puebla, and Hidalgo, hold important diversity (García-De la Cruz et al., 2013; García-Franco et al., 2008; González-Espinosa, 2011; Williams-Linera, 2002; Williams-Linera et al., 2013), mainly in a beta-diversity sense, which is most important for Mexican cloud forest biotas (Carvajal-Hernández et al., 2014; Williams-Linera et al., 2013).
Species losses have important impacts on beta diversity, often narrowing community composition and increasing floristic homogenization (Arroyo-Rodríguez et al., 2013). According to our model predictions, some patches will see strong reductions of tree diversity (Table 4), indicating high climate change sensitivity of these endangered species, as has been pointed out in previous studies (Eigenbrod et al., 2015; Figueroa-Rangel et al., 2009; Rojas-Soto et al., 2012; Vargas-Rodríguez et al., 2010).
Our models provided information about likely sensitivity of threatened tree species of cloud forest to global climate change processes, and how cloud forest will likely see significant changes in distributions of species, both increases and reductions in species diversity at particular sites (Figueroa-Rangel et al., 2009). ENMs were used by Rojas-Soto et al. (2012) to assess climate change influences on 20 Mexican cloud forest tree species, in a first exploration under a previous generation of climate change projections (IPCC, 2001, 2007). We added to this picture consideration of more cloud forest patches across Mexico (e.g., in the Sierra Madre Occidental, Transverse Volcanic Belt, and in the northeast), and by including detailed model selection and evaluation, and careful assessment of uncertainty inherent in our predictions. Both studies indicate considerable instability of Mexican cloud forest biodiversity in the face of climate change, and considerable inadequacy of the present natural protected areas system for Mexican cloud forests (Fig. 6). Téllez-Valdés et al. (2006) assessed a single, restricted-range species (Fagus grandifolia var. mexicana), and also predicted strong range reductions for that species. Our work detailed model selection exercises, and consideration of 20 GCM and 2 RCP emissions scenarios to arrive at the most detailed predictions yet for this ecosystem.
Our future-climate model projections indicated considerable potential for changes in tree species' distributions in cloud forest (Table 4). To the extent that dispersal and colonization are feasible in a naturally fragmented and highly human affected ecosystem like cloud forest (they are probably often not feasible), alpha diversity would shift consistently southward, which is opposite of the usual expectation for the Northern Hemisphere (Zhu et al., 2012). Conservation implications of these changes center on the need for preservation of broad areas of cloud forest, yet no clear conservation strategy presently exists for this ecosystem (Aldrich et al., 1997; Foster, 2001; Ponce-Reyes et al., 2012; Van Beusekom et al., 2017; Vega et al., 2000). Our work provides a geographic summary for endangered tree species that can serve as benchmarks for future species compositions, although balanced and limited by low data availability for cloud forest, which reflects low historical and ongoing monitoring effort (González-Espinosa, 2011). We recognize that another important driver in cloud forest species loss is landscape change; this factor was not evaluated in our study for lack of detailed future scenarios for those changes.
Cloud forest is projected to see significant negative effects in terms of loss of populations of threatened and endangered cloud forest trees in the face of climate change. This result is particularly concerning because the most important genus in this biome is Quercus, and this genus is precisely the one projected to see the most serious negative effects. Our results are limited to climate change implications, although land use change is important as well; however, we summarize likely conservation implications of one important factor affecting cloud forest. We explore our results as regards the conservation of this biome, focusing on the cloud forests of the eastern slopes of the Sierra Madre Oriental, which remain entirely unprotected.